238 Accuracy, Yield and Clinical Impact of a Low-Cost High Resolution Microendoscope in the Early Diagnosis of Esophageal Adenocarcinoma
GASTROENTEROLOGY(2016)
Abstract
Introduction: Volumetric laser endomicroscopy (VLE) incorporates optical frequency domain imaging technology in a balloon-based system, which is capable of scanning the esophagus circumferentially over 6 cm, up to a depth of 3 mm with near-microscopic resolution.VLE has the potential to improve detection of early neoplasia in Barrett's esophagus (BE).However, interpretation of VLE images is complex due to subtle differences in architecture and gray-scale color and the large amount of images that needs to be scrutinized by the endoscopist (a 6-cm VLE scan contains 1200 frames).A recently developed clinical prediction model of VLE features for BE neoplasia showed a reasonable accuracy (AUC of 0.81).Aim of this study was to investigate the feasibility of a computer algorithm to identify early BE neoplasia on ex vivo VLE images.Methods: Sixty VLE images from a database of high-quality one-to-one VLE-histology correlations were used (30 non-dysplastic (ND)BE and 30 neoplastic images; high-grade dysplasia and early adenocarcinoma), consisting of VLE scans from endoscopic resection specimens of Barrett's patients +/-neoplasia.VLE images were normalized to a height of 400 pixels in order to obtain a standardized zoom factor.Previously identified VLE features predictive for BE neoplasia served as clinical input for the algorithm: 1) higher OFDI surface signal than subsurface signal in tissue, 2) lack of layering.From these VLE features an algorithm feature was developed analyzing both top layers and surface signal.A signal intensity histogram using 8 intensity categories was calculated over the first 4 layers of 50 pixels, starting at the top of the image.Linear support vector machine was used to classify the images according to the used VLE texture features.Leave-one-out cross-validation was employed for validation of the algorithm.Results: Using the correlated histology as the reference standard, sensitivity, specificity and accuracy for the algorithm to detect BE neoplasia were 93%, 70% and 82%, respectively.The performance of the algorithm was good, with an area under the receiver operating curve (AUC) of 0.91 to detect BE neoplasia in ex vivo VLE images.Most distinctive features of the algorithm are the top layers and mid-range intensities of the histogram.Conclusion: This is the first study in which a computer algorithm for BE neoplasia was developed based on VLE images with direct histological correlates.The algorithm showed good performance to detect BE neoplasia in ex vivo VLE images (AUC 0.91).Compared to the performance of a recently developed clinical VLE prediction score (AUC 0.81), this study suggests that an automatic detection algorithm seems to perform at least as good as assessment by VLE experts in detecting early neoplasia on VLE.Future studies on in vivo VLE scans are needed to further validate the algorithm.
MoreTranslated text
Key words
Barrett's Esophagus
AI Read Science
Must-Reading Tree
Example
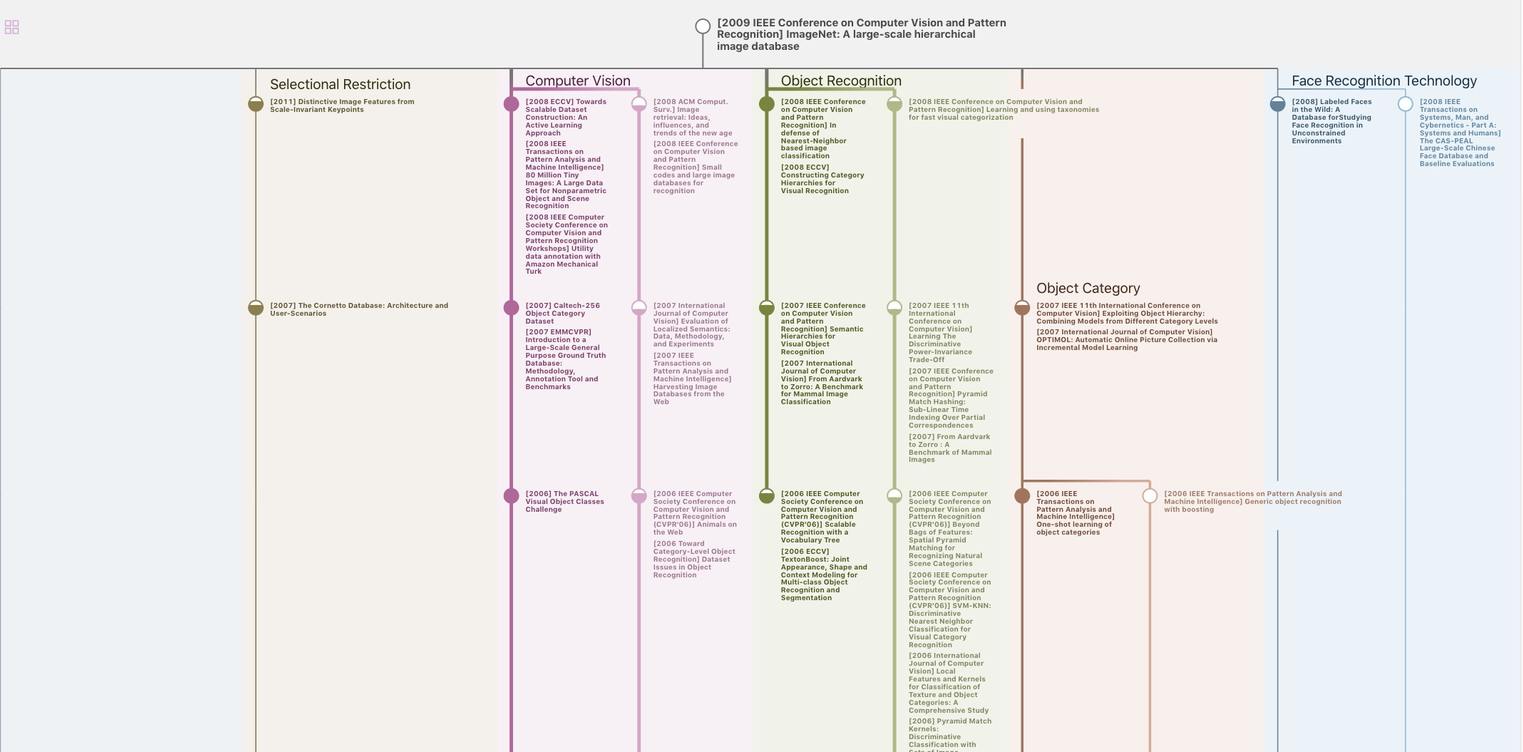
Generate MRT to find the research sequence of this paper
Chat Paper
Summary is being generated by the instructions you defined