Doubly Stochastic Primal-Dual Coordinate Method for Empirical Risk Minimization and Bilinear Saddle-Point Problem
arXiv: Learning(2015)
摘要
We proposed a doubly stochastic primal-dual coordinate (DSPDC) optimization algorithm for empirical risk minimization, which can be formulated as a bilinear saddle-point problem. In each iteration, our method randomly samples a block of coordinates of the primal and dual solutions to update. The convergence of our method is established in both the distance from the current iterate to the optimal solution and the primal-dual objective gap. We show that the proposed method has a lower overall complexity than existing coordinate methods when either the data matrix has a factorized structure or the proximal mapping on each block is computationally expensive, e.g., involves an eigenvalue decomposition. Furthermore, we give a theoretical lower bound on the iteration complexity of a general family of primal-dual (block) coordinate methods for bilinear saddle-point problems, which also includes DSPDC.
更多查看译文
AI 理解论文
溯源树
样例
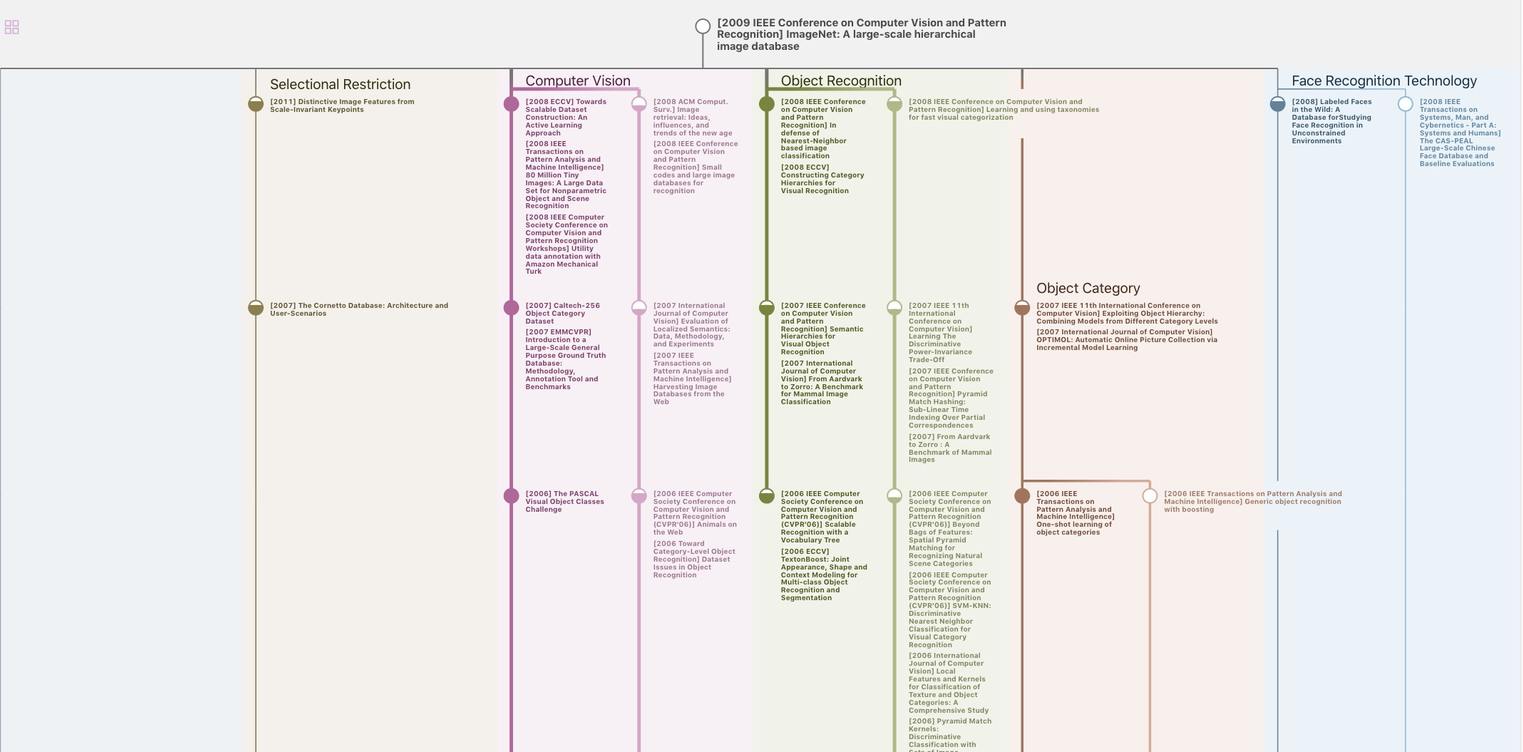
生成溯源树,研究论文发展脉络
Chat Paper
正在生成论文摘要