Nonparametric Estimation and Forecasting for Time-Varying Coefficient Realized Volatility Models
JOURNAL OF BUSINESS & ECONOMIC STATISTICS(2018)
Abstract
This article introduces a new specification for the heterogenous autoregressive (HAR) model for the realized volatility of S&P 500 index returns. In this modeling framework, the coefficients of the HAR are allowed to be time-varying with unspecified functional forms. The local linear method with the cross-validation (CV) bandwidth selection is applied to estimate the time-varying coefficient HAR (TVC-HAR) model, and a bootstrap method is used to construct the point-wise confidence bands for the coefficient functions. Furthermore, the asymptotic distribution of the proposed local linear estimators of the TVC-HAR model is established under some mild conditions. The results of the simulation study show that the local linear estimator with CV bandwidth selection has favorable finite sample properties. The outcomes of the conditional predictive ability test indicate that the proposed nonparametric TVC-HAR model outperforms the parametric HAR and its extension to HAR with jumps and/or GARCH in terms of multi-step out-of-sample forecasting, in particular in the post-2003 crisis and 2007 global financial crisis (GFC) periods, during which financial market volatilities were unduly high.
MoreTranslated text
Key words
Bootstrap method,Heterogenous autoregressive model,Local linear estimation,Locally stationary process,Nonparametric method,Time-varying coefficient models
AI Read Science
Must-Reading Tree
Example
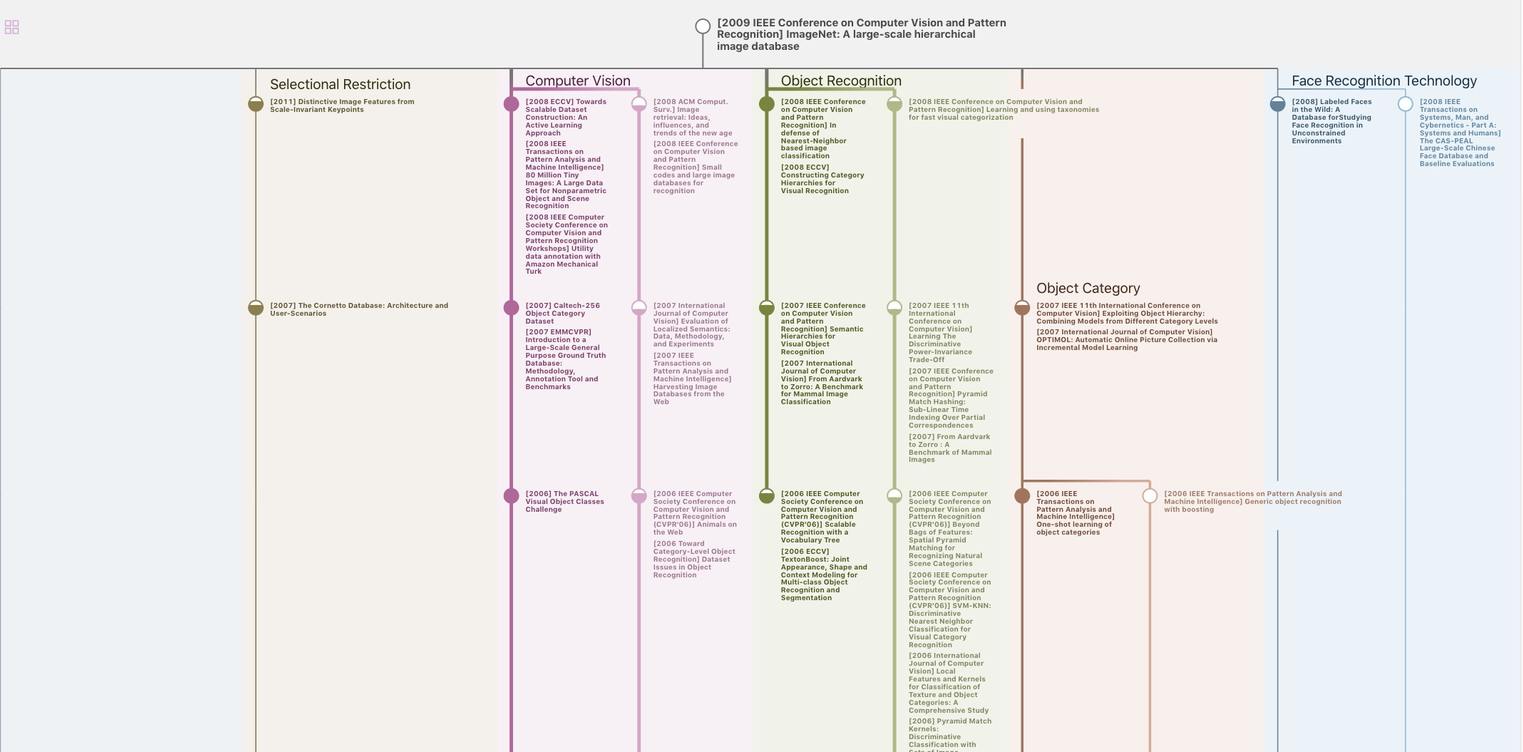
Generate MRT to find the research sequence of this paper
Chat Paper
Summary is being generated by the instructions you defined