Shades of Gray: Seeing the Full Spectrum of Practical Data De-Identification
Santa Clara law review(2016)
摘要
One of the most hotly debated issues in privacy and data security is the notion of identifiability of personal data and its technological corollary, de-identification. De-identification is the process of removing personally identifiable information from data collected, stored and used by organizations. Once viewed as a silver bullet allowing organizations to reap the benefits of data while minimizing privacy and data security risks, de-identification has come under intense scrutiny with academic research papers and popular media reports highlighting its shortcomings. At the same time, organizations around the world necessarily continue to rely on a wide range of technical, administrative and legal measures to reduce the identifiability of personal data to enable critical uses and valuable research while providing protection to individuals’ identity and privacy. The debate around the contours of the term personally identifiable information, which triggers a set of legal and regulatory protections, continues to rage. Scientists and regulators frequently refer to certain categories of information as “personal” even as businesses and trade groups define them as “de-identified” or “non-personal.” The stakes in the debate are high. While not foolproof, de-identification techniques unlock value by enabling important public and private research, allowing for the maintenance and use – and, in certain cases, sharing and publication – of valuable information, while mitigating privacy risk. This paper proposes parameters for calibrating legal rules to data depending on multiple gradations of identifiability, while also assessing other factors such as an organization’s safeguards and controls, as well as the data’s sensitivity, accessibility and permanence. It builds on emerging scholarship that suggests that rather than treat data as a black or white dichotomy, policymakers should view data in various shades of gray; and provides guidance on where to place important legal and technical boundaries between categories of identifiability. It urges the development of policy that creates incentives for organizations to avoid explicit identification and deploy elaborate safeguards and controls, while at the same time maintaining the utility of data sets.u0000
更多查看译文
关键词
data,de-identification
AI 理解论文
溯源树
样例
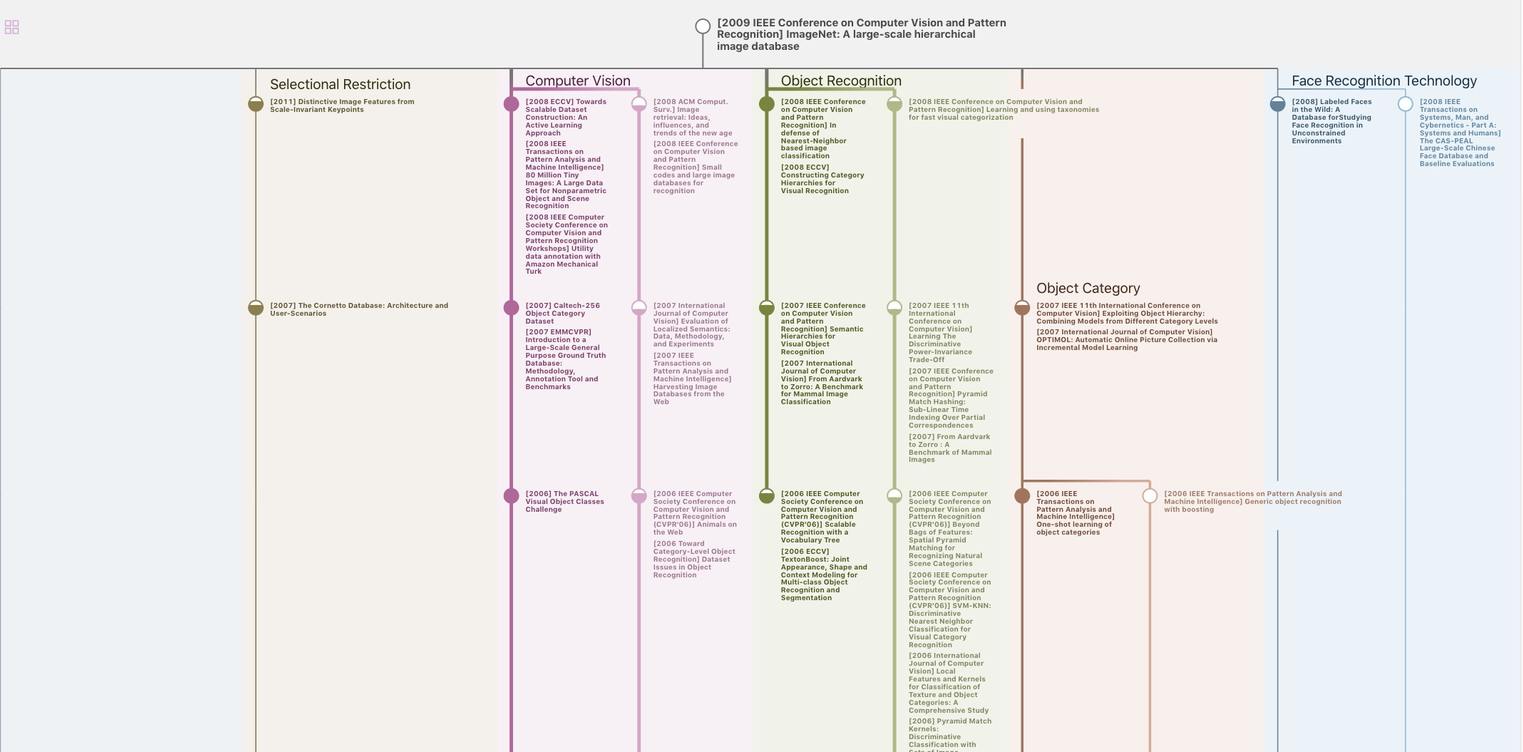
生成溯源树,研究论文发展脉络
Chat Paper
正在生成论文摘要