Quantile universal threshold for model selection
arXiv: Methodology(2015)
摘要
In various settings such as regression, wavelet denoising and low rank matrix estimation, statisticians seek to find a low dimensional structure based on high dimensional data. In those instances, the maximum likelihood is unstable or might not be unique. By introducing some constraint on the parameters, a class of regularized estimators such as subset selection and the lasso allow to perform variable selection and parameter estimation by thresholding some parameters to zero. Yet one crucial step challenges all these estimators: the selection of the threshold parameter. If too large, important features are missing; if too small, false features are included. After defining a zero-thresholding function, we present a unified framework to select features by identifying a threshold $lambda$ at the detection edge under the null model. We apply our methodology to existing estimators, with a particular emphasis on $ell_1$-regularized generalized linear models with the lasso as a special case. Numerical results show the effectiveness of our approach in terms of model selection and prediction.
更多查看译文
AI 理解论文
溯源树
样例
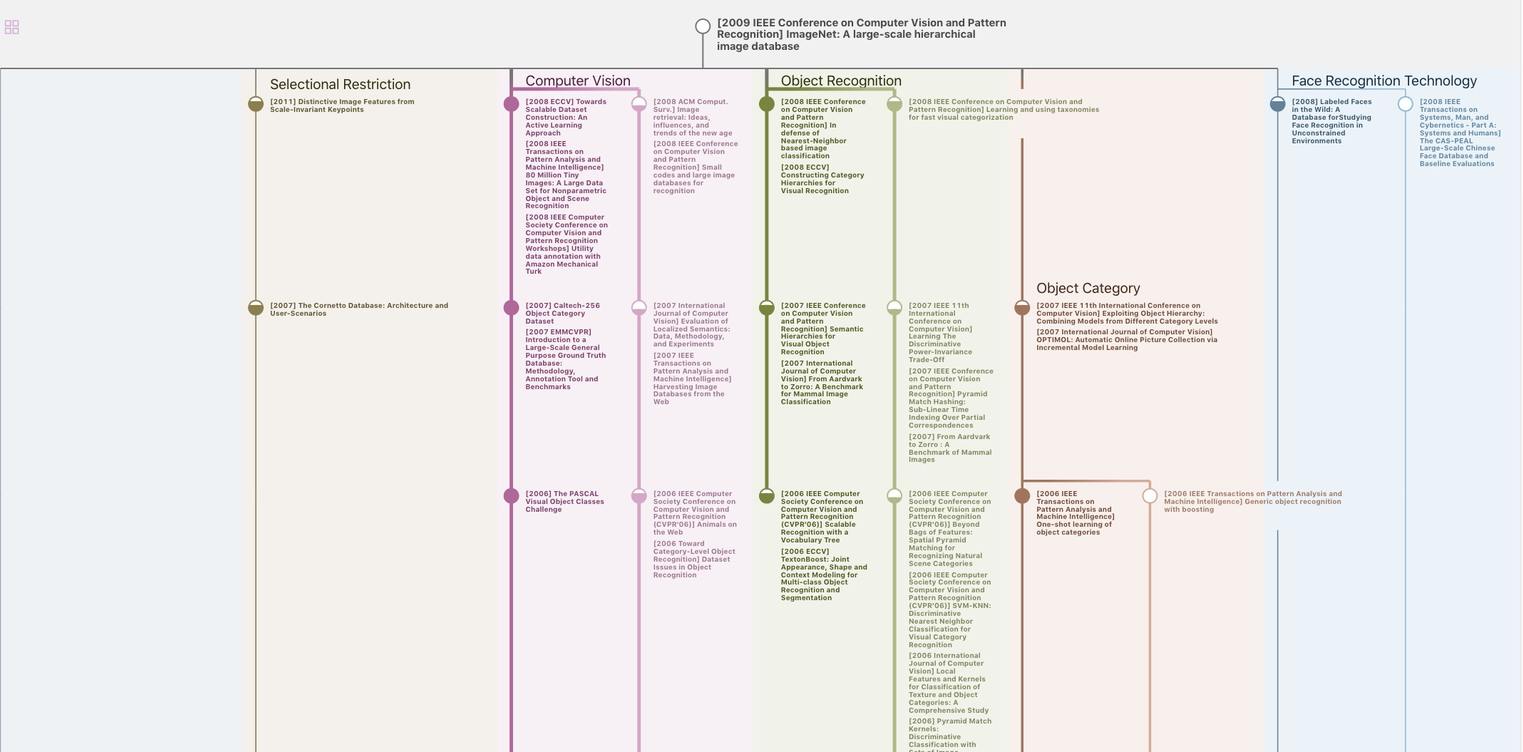
生成溯源树,研究论文发展脉络
Chat Paper
正在生成论文摘要