An introduction to sampling via measure transport
arXiv: Computation(2016)
Abstract
We present the fundamentals of a measure transport approach to sampling. The idea is to construct a deterministic coupling---i.e., a transport map---between a complex probability measure of interest and a simpler reference measure. Given a transport map, one can generate arbitrarily many independent and unweighted samples from the target simply by pushing forward reference samples through the map. We consider two different and complementary scenarios: first, when only evaluations of the unnormalized target density are available, and second, when the target distribution is known only through a finite collection of samples. We show that in both settings the desired transports can be characterized as the solutions of variational problems. We then address practical issues associated with the optimization--based construction of transports: choosing finite-dimensional parameterizations of the map, enforcing monotonicity, quantifying the error of approximate transports, and refining approximate transports by enriching the corresponding approximation spaces. Approximate transports can also be used to Gaussianize complex distributions and thus precondition conventional asymptotically exact sampling schemes. We place the measure transport approach in broader context, describing connections with other optimization--based samplers, with inference and density estimation schemes using optimal transport, and with alternative transformation--based approaches to simulation. We also sketch current work aimed at the construction of transport maps in high dimensions, exploiting essential features of the target distribution (e.g., conditional independence, low-rank structure). The approaches and algorithms presented here have direct applications to Bayesian computation and to broader problems of stochastic simulation.
MoreTranslated text
AI Read Science
Must-Reading Tree
Example
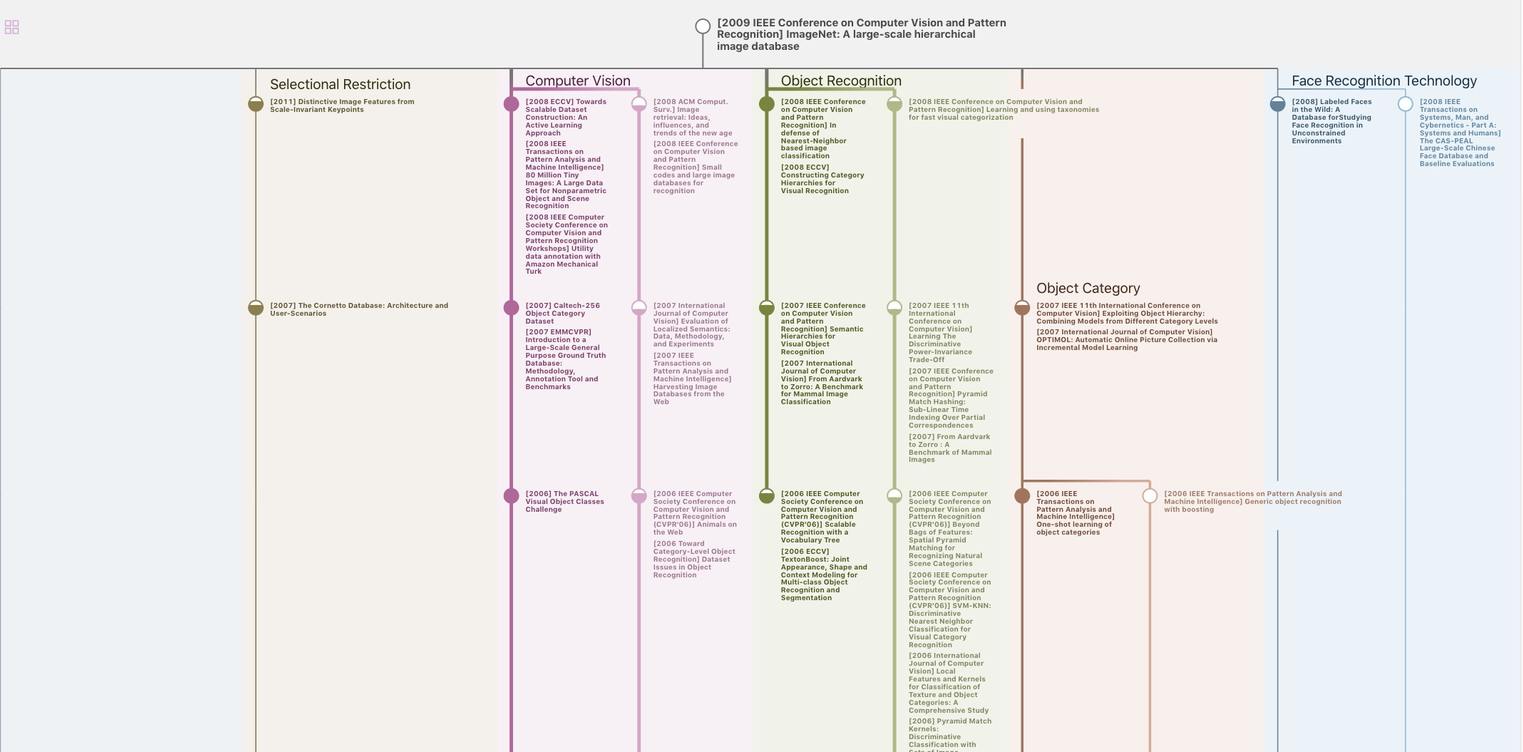
Generate MRT to find the research sequence of this paper
Chat Paper
Summary is being generated by the instructions you defined