A Multiscale Strategy for Bayesian Inference Using Transport Maps
SIAM-ASA JOURNAL ON UNCERTAINTY QUANTIFICATION(2016)
摘要
In many inverse problems, model parameters cannot be precisely determined from observational data. Bayesian inference provides a mechanism for capturing the resulting parameter uncertainty, but typically at a high computational cost. This work introduces a multiscale decomposition that exploits conditional independence across scales, when present in certain classes of inverse problems, to decouple Bayesian inference into two stages: (1) a computationally tractable coarse-scale inference problem, and (2) a mapping of the low-dimensional coarse-scale posterior distribution into the original high-dimensional parameter space. This decomposition relies on a characterization of the non-Gaussian joint distribution of coarse-and fine-scale quantities via optimal transport maps. We demonstrate our approach on a sequence of inverse problems arising in subsurface flow, using the multiscale finite element method to discretize the steady state pressure equation. We compare the multiscale strategy with full-dimensional Markov chain Monte Carlo on a problem of moderate dimension (100 parameters) and then use it to infer a conductivity field described by over 10000 parameters.
更多查看译文
关键词
Bayesian inference,inverse problems,multiscale modeling,multiscale finite element method,optimal transportation,Markov chain Monte Carlo
AI 理解论文
溯源树
样例
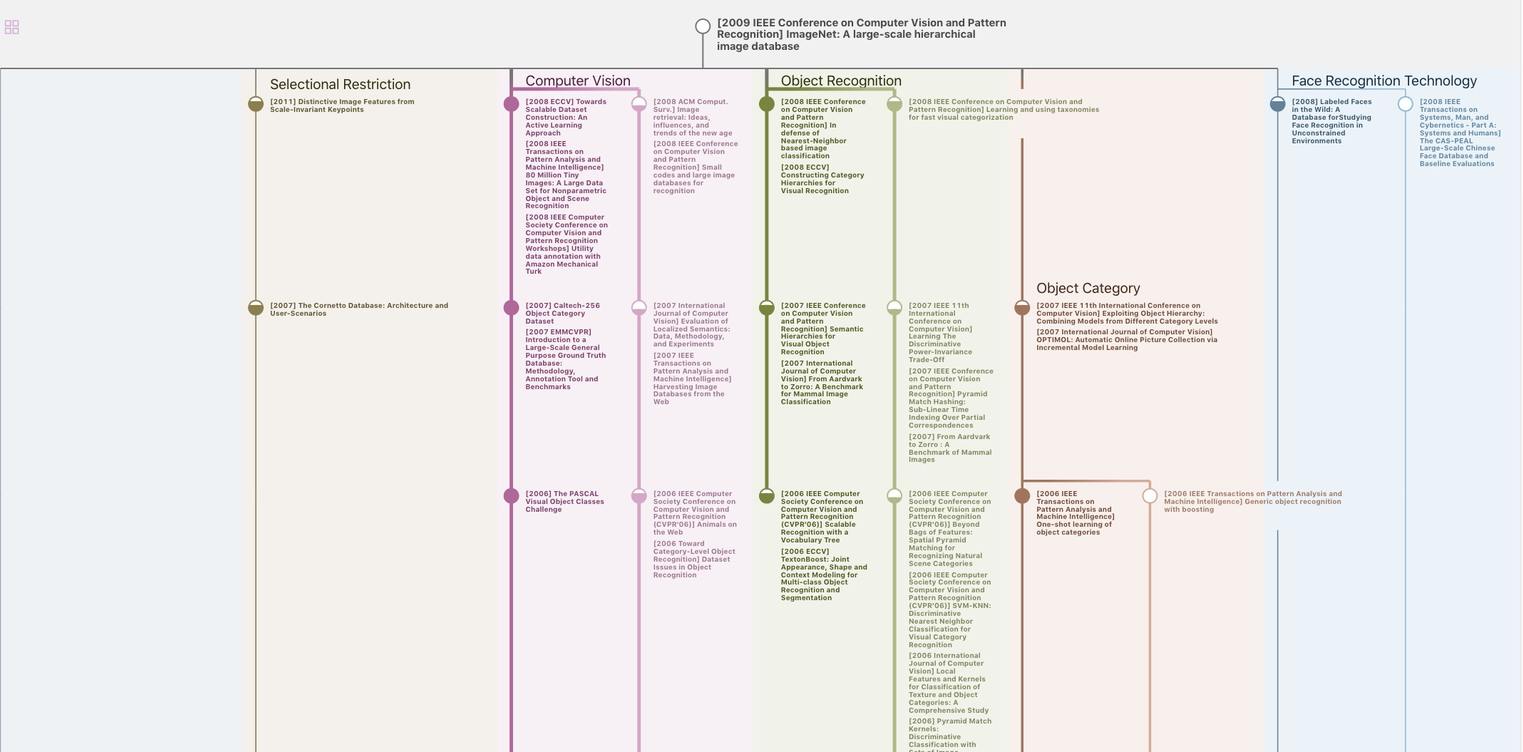
生成溯源树,研究论文发展脉络
Chat Paper
正在生成论文摘要