Abstract TP236: The Stroke Hyperglycemia Insulin Network Effort (SHINE) Trial: A Case Study of a Bayesian Trial Design
Stroke(2013)
摘要
Introduction: The “Adaptive Designs Accelerating Promising Trials into Treatments (ADAPT-IT)” project is a collaborative effort supported by the NIH and FDA to explore how adaptive clinical trial design might improve the evaluation of drugs and medical devices. We use the NINDS-supported Neurological Emergencies Treatment Trials network as a "laboratory" in which to study the development of adaptive clinical trial designs. The Stroke Hyperglycemia Insulin Network Effort (SHINE) trial was fully funded by the NIH-NINDS at the start of ADAPT-IT and is a currently ongoing phase III trial of tight glucose control in hyperglycemic acute ischemic stroke patients. Within ADAPT-IT, a Bayesian alternative design was developed. The primary endpoint is a severity-adjusted dichotomized 90-day modified Rankin scale (mRS). Objective: To present both designs and compare their operating characteristics. Methods: 10000 trials are simulated under treatment effects ranging from 0% to 7%. We present the mean sample size and probabilities of trial success or futility. Results: The SHINE trial design includes a group sequential procedure with 4 interim analyses to monitor for early efficacy and futility. A maximum of 1400 patients provide 80% power to detect a 7% absolute difference between treatment arms with an overall Type I error rate of 5%. The expected sample size is 1050 patients. This design also incorporates sample size re-estimation and response adaptive randomization. The Bayesian alternative would enroll a maximum of 1400 patients, equally randomized, but employs more frequent interim looks based on predictive probabilities and incorporates a longitudinal model of the primary endpoint. This design has similar power and Type I error and would enroll a mean of 733 and 979 patients under the null and alternative hypotheses respectively. Conclusions: Simulations suggest that the designs have similar power and Type I error. The Bayesian alternative, with more frequent looks, has a greater chance of stopping early for overwhelming efficacy or futility. The Bayesian alternative will be retrospectively executed upon completion of SHINE to later compare the designs based on their use of patient resources, time, and strength of conclusions in a real world setting.
更多查看译文
AI 理解论文
溯源树
样例
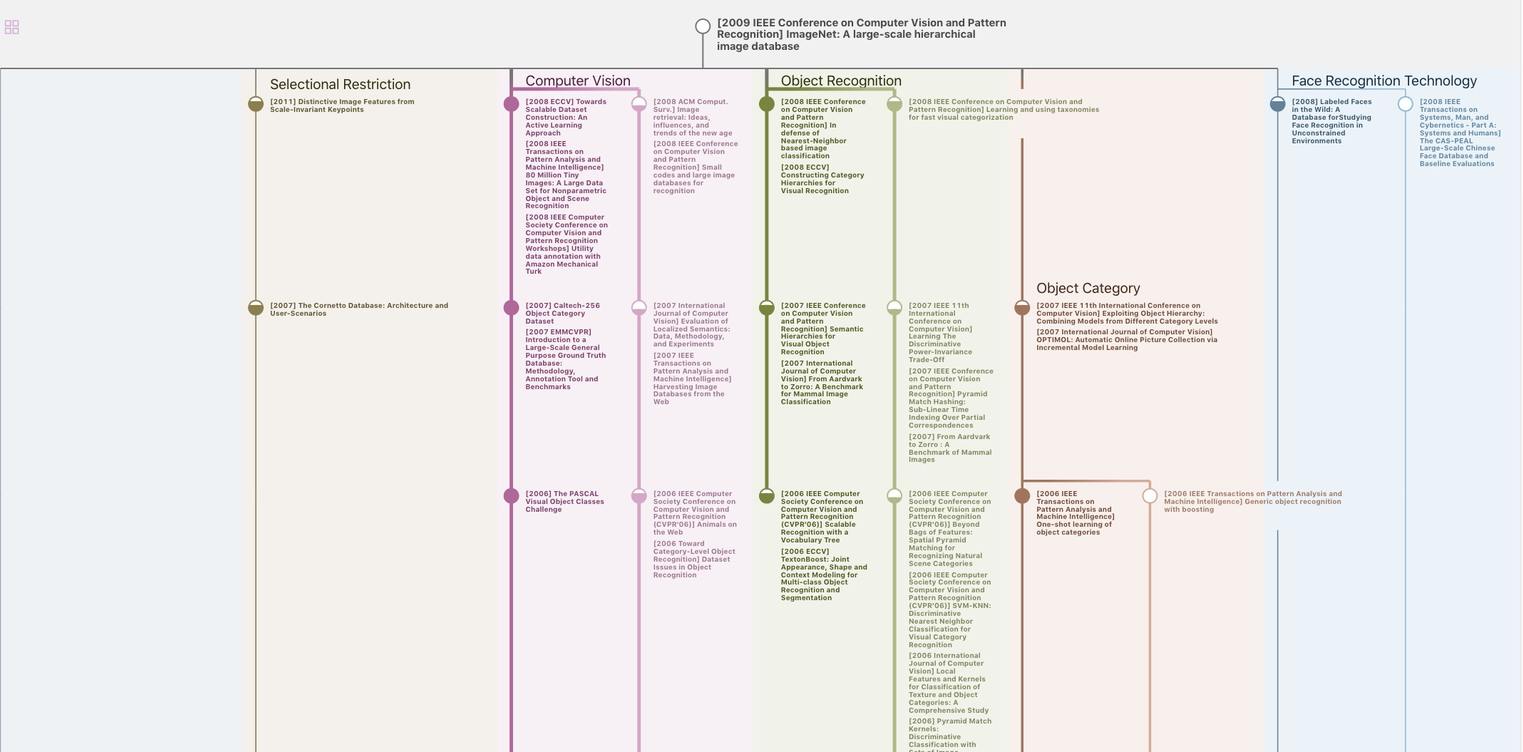
生成溯源树,研究论文发展脉络
Chat Paper
正在生成论文摘要