Sequential double cross-validation for augmented prediction assessment in high-dimensional omic applications
arXiv: Methodology(2016)
摘要
Augmentation of previously established high-dimensional predictive models with new biomolecular markers is an important task in omic applications. We introduce a two-step procedure for the assessment of the augmented predictive value of omic predictors, based on sequential double cross-validation and regularized regression models. We propose several performance indices to summarize the two-stage prediction procedure and a permutation test to formally assess the augmented predictive value of a second omic set of predictors over a primary omic source. The performance of the test is investigated through simulations. We illustrate the new method through the systematic assessment and comparison of the performance of transcriptomics and metabolomics sources in the prediction of body mass index (BMI) using data from the Dietary, Lifestyle, and Genetic determinants of Obesity and Metabolic syndrome (DILGOM) study, a population-based cohort, from Finland.
更多查看译文
AI 理解论文
溯源树
样例
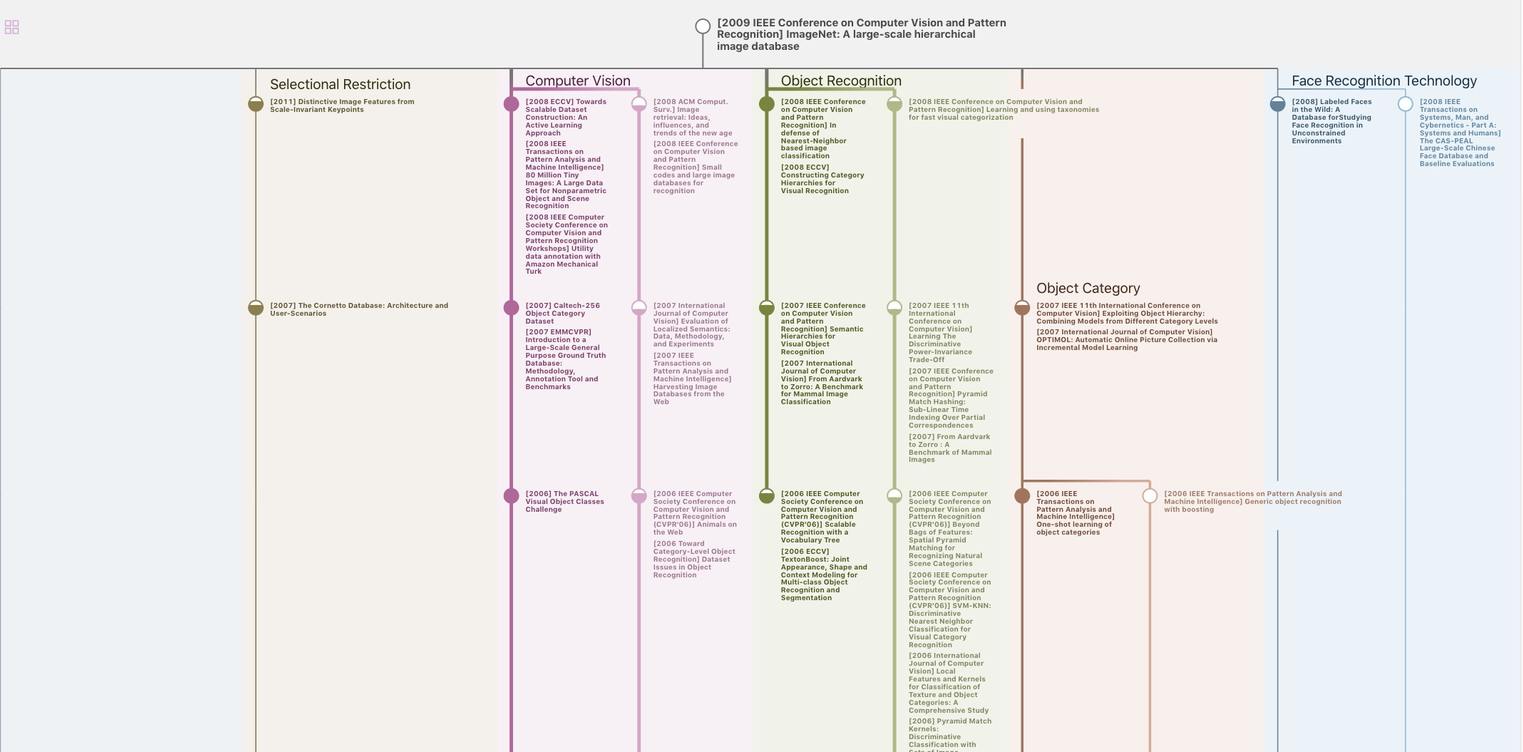
生成溯源树,研究论文发展脉络
Chat Paper
正在生成论文摘要