A hybrid SVR-BO model for predicting the soil thermal conductivity with uncertainty
CANADIAN GEOTECHNICAL JOURNAL(2024)
摘要
This study proposes a generalised framework for developing a hybrid machine learning (ML) model that combines support vector regression (SVR) with hyperparameter optimisation to predict thermal conductivity (k) with uncertainty. The frame-work contains four phases: data pre-processing, determining the best-performing hybrid model, selecting the optimal input combination, and uncertainty implementation. A database containing 2197 data points is first compiled to train the ML model. Three hyperparameter optimisation algorithms are adopted to tune hyperparameters, and their performance is evaluated by model evaluation metrics. Results show that SVR with Bayesian optimisation (SVR-BO) is the best-performing model since it produces more accurate predictions for k than models that employ grid and random searches. Given the sample insufficiency issue encountered in practice, the SVR-BO models with 144 input combinations are analysed. The compassion among models under various input combinations indicates that incorporating temperature as an additional input can provide moderate im-provement in the accuracy and generalisability of the hybrid model. Based on the comparison, a five-input model is selected as the best candidate to implement the uncertainty evaluation for k. Results demonstrate that the predicted k possesses higher reliability for denser datasets and shows promising potential for applications in k with uncertainty assessments.
更多查看译文
关键词
support vector regression (SVR),soil thermal conductivity,Bayesian optimisation,optimal input combination,bootstrap technique,uncertainty
AI 理解论文
溯源树
样例
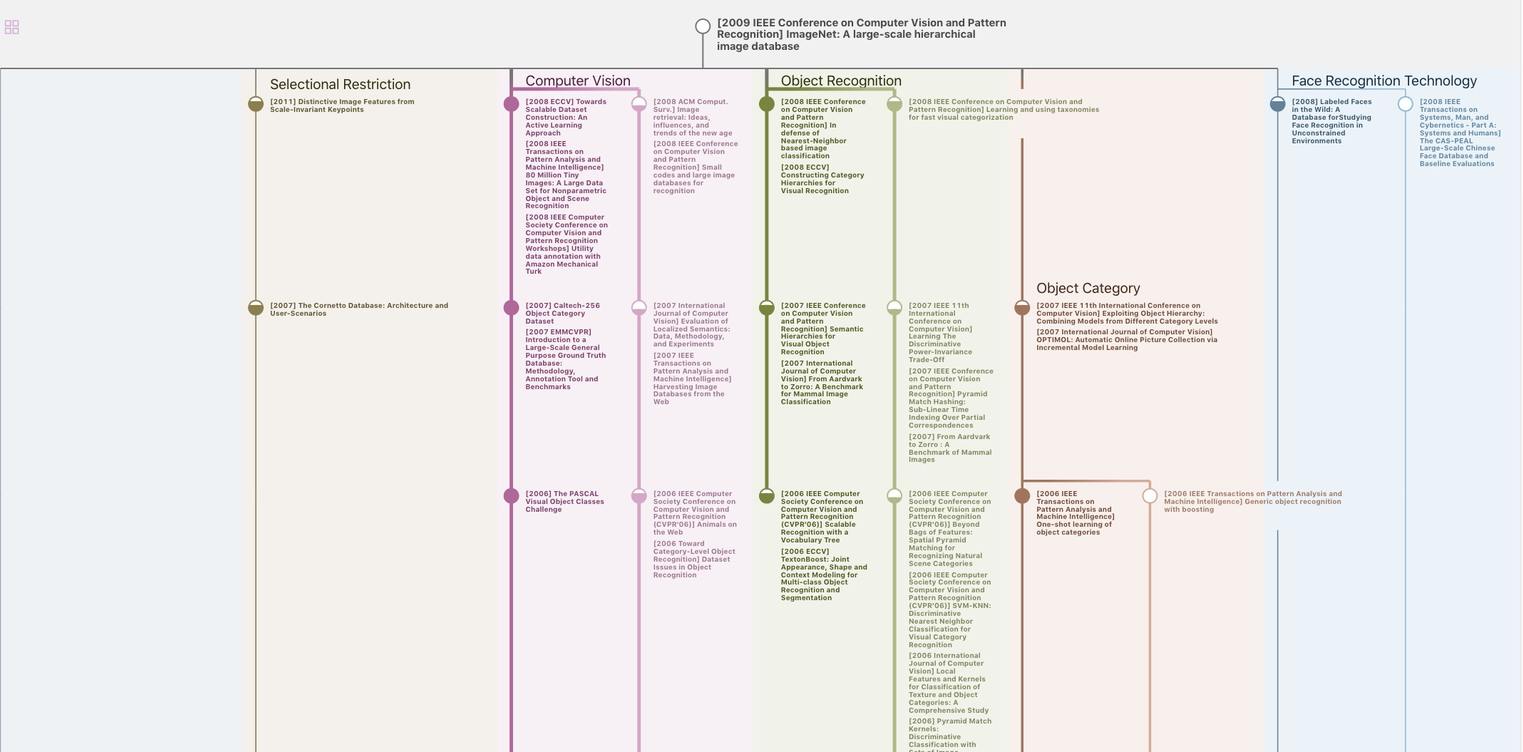
生成溯源树,研究论文发展脉络
Chat Paper
正在生成论文摘要