Optimal Adaptive Testing: Informativeness and Incentives
THEORETICAL ECONOMICS(2018)
摘要
We introduce a learning framework in which a principal seeks to determine the ability of a strategic agent. The principal assigns a test consisting of a finite sequence of tasks. The test is adaptive: each task that is assigned can depend on the agent's past performance. The probability of success on a task is jointly determined by the agent's privately known ability and an unobserved effort level that he chooses to maximize the probability of passing the test. We identify a simple monotonicity condition under which the principal always employs the most (statistically) informative task in the optimal adaptive test. Conversely, whenever the condition is violated, we show that there are cases in which the principal strictly prefers to use less informative tasks.
更多查看译文
关键词
Adaptive testing,dynamic learning,ratcheting,testing experts
AI 理解论文
溯源树
样例
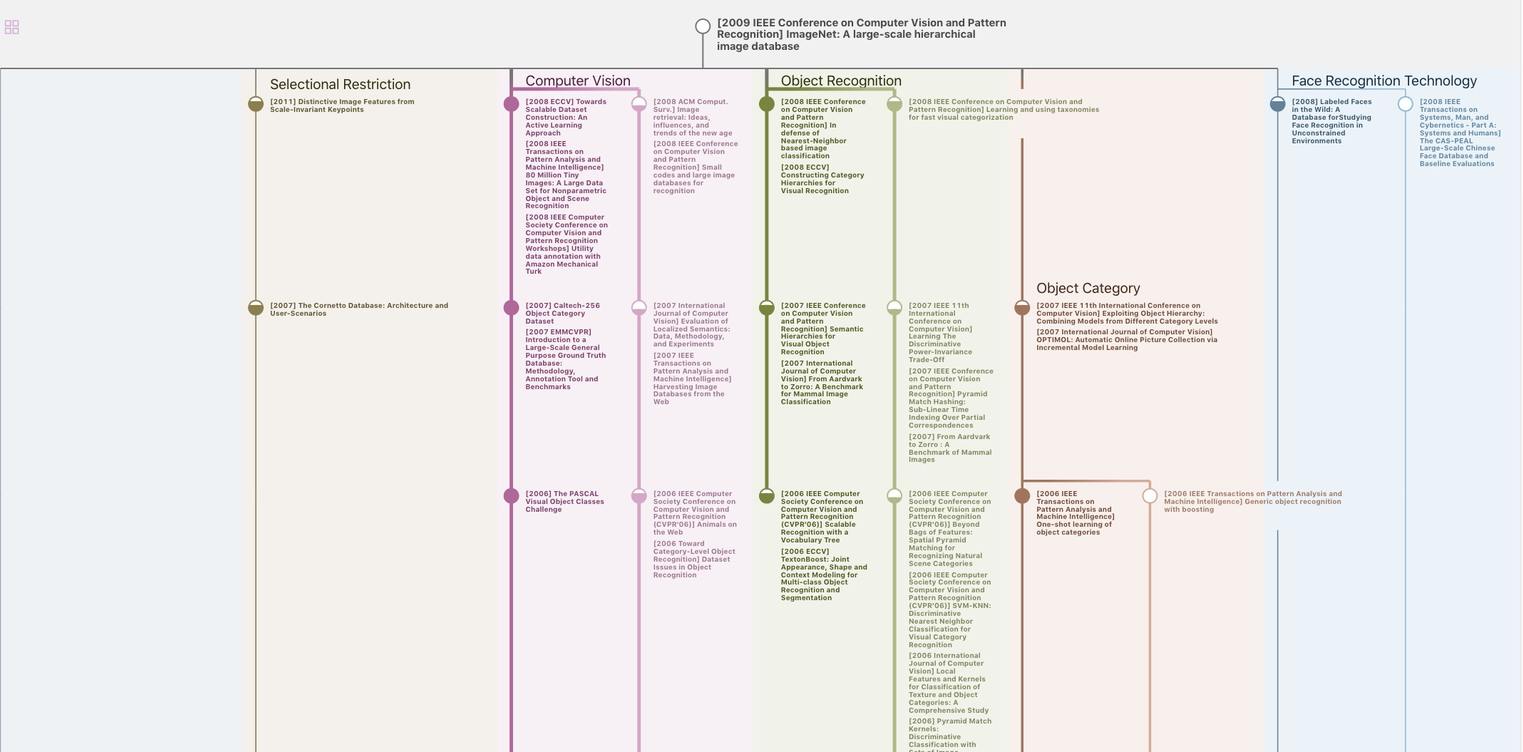
生成溯源树,研究论文发展脉络
Chat Paper
正在生成论文摘要