Effect of model error representation in the Yellow and East China Sea modeling system based on the ensemble Kalman filter
Ocean Dynamics(2016)
Abstract
To increase the accuracy of ocean predictions in the Yellow and East China Sea (YES), the satellite-borne sea surface temperature (SST) data have been assimilated to an operational ocean modeling system by applying an ensemble Kalman filter (EnKF). As the observed SST was assimilated continuously into the model with time, the ensemble spread decreased and the efficiency of data assimilation degenerated. To increase liability of the system and the ensemble spread, model uncertainties were represented stochastically by perturbing the model tendency parameters such as eddy viscosity, bottom drag coefficient, light attenuation depth, as well as atmospheric forcing. Data assimilation experiments were performed with forcing from a regional atmospheric model from September 2011 to February 2012. The assimilation results with and without the stochastically perturbed model parameters and atmospheric forcing were compared. The ensemble with the perturbations has larger spread and smaller root-mean-square deviation (RMSD) in temperature compared with the ensemble without the perturbations. The SST RMSD relative to anther supplementary SST dataset was reduced from 0.91 to 0.81 °C over the YES. The assimilation of the SST data improved the simulated SST compared with the observation at the ocean buoy stations, and also made the subsurface temperature profiles closer to the observed ones. The assimilation experiments showed that a stochastic representation of the model errors by the perturbations of the model parameters and atmospheric forcing increases the spread of the ensemble and improves the structure of background error covariance, which enhances the performance of the ensemble modeling system in the YES.
MoreTranslated text
Key words
Ensemble Kalman filter, Assimilation, Model parameter perturbation, Yellow Sea, SST
AI Read Science
Must-Reading Tree
Example
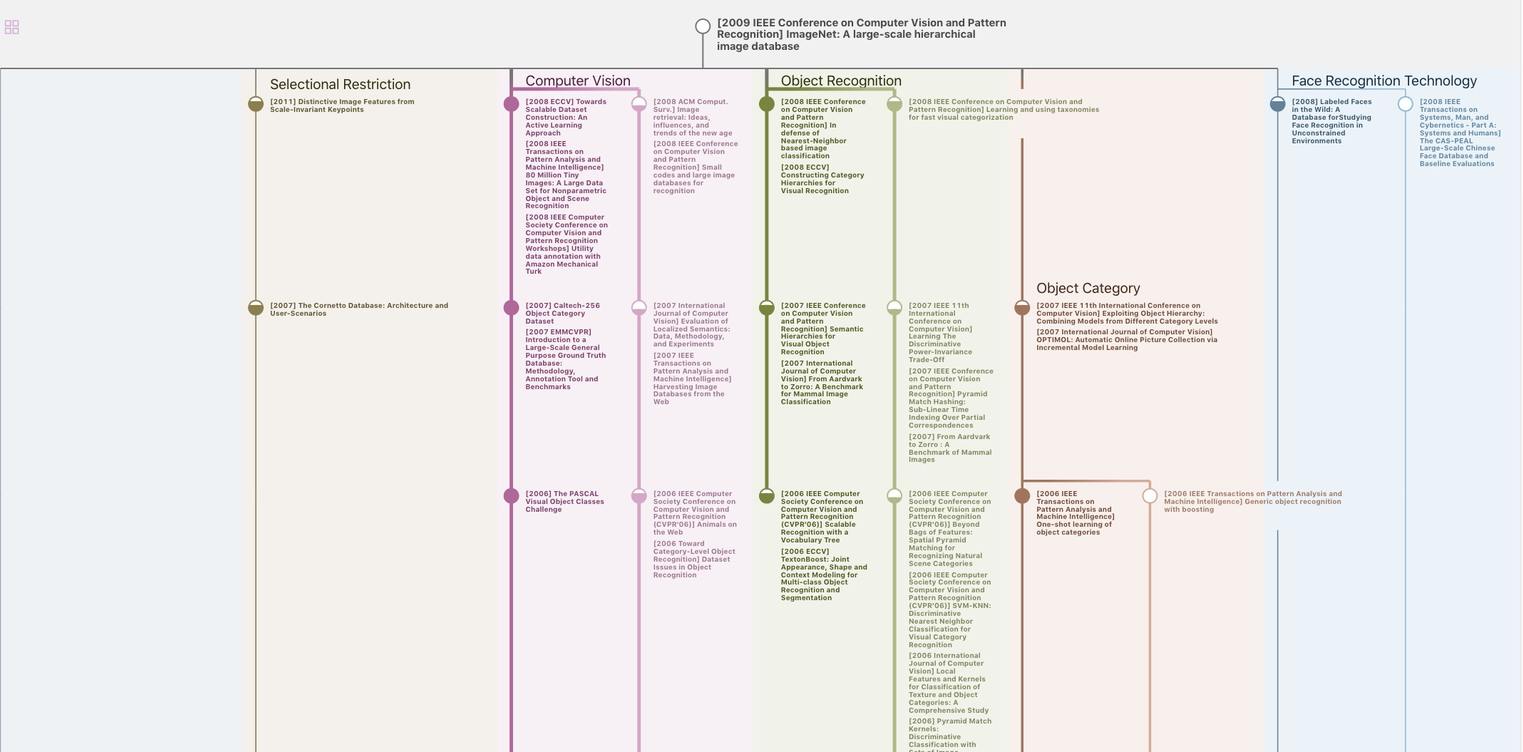
Generate MRT to find the research sequence of this paper
Chat Paper
Summary is being generated by the instructions you defined