Fast esophageal layer segmentation in OCT images of guinea pigs based on sparse Bayesian classification and graph search.
BIOMEDICAL OPTICS EXPRESS(2019)
摘要
Endoscopic optical coherence tomography (OCT) devices are capable of generating high-resolution images of esophageal structures at high speed. To make the obtained data easy to interpret and reveal the clinical significance, an automatic segmentation algorithm is needed. This work proposes a fast algorithm combining sparse Bayesian learning and graph search (termed as SBGS) to automatically identify six layer boundaries on esophageal OCT images. The SBGS first extracts features, including multi-scale gradients, averages and Gabor wavelet coefficients, to train the sparse Bayesian classifier, which is used to generate probability maps indicating boundary positions. Given these probability maps, the graph search method is employed to create the final continuous smooth boundaries. The segmentation performance of the proposed SBGS algorithm was verified by esophageal OCT images from healthy guinea pigs and the eosinophilic esophagitis (EoE) models. Experiments confirmed that the SBGS method is able to implement robust esophageal segmentation for all the tested cases. In addition, benefiting from the sparse model of SBGS, the segmentation efficiency is significantly improved compared to other widely used techniques. (C) 2019 Optical Society of America under the terms of the OSA Open Access Publishing Agreement
更多查看译文
关键词
fast esophageal layer segmentation,guinea pigs,oct images,sparse bayesian classification
AI 理解论文
溯源树
样例
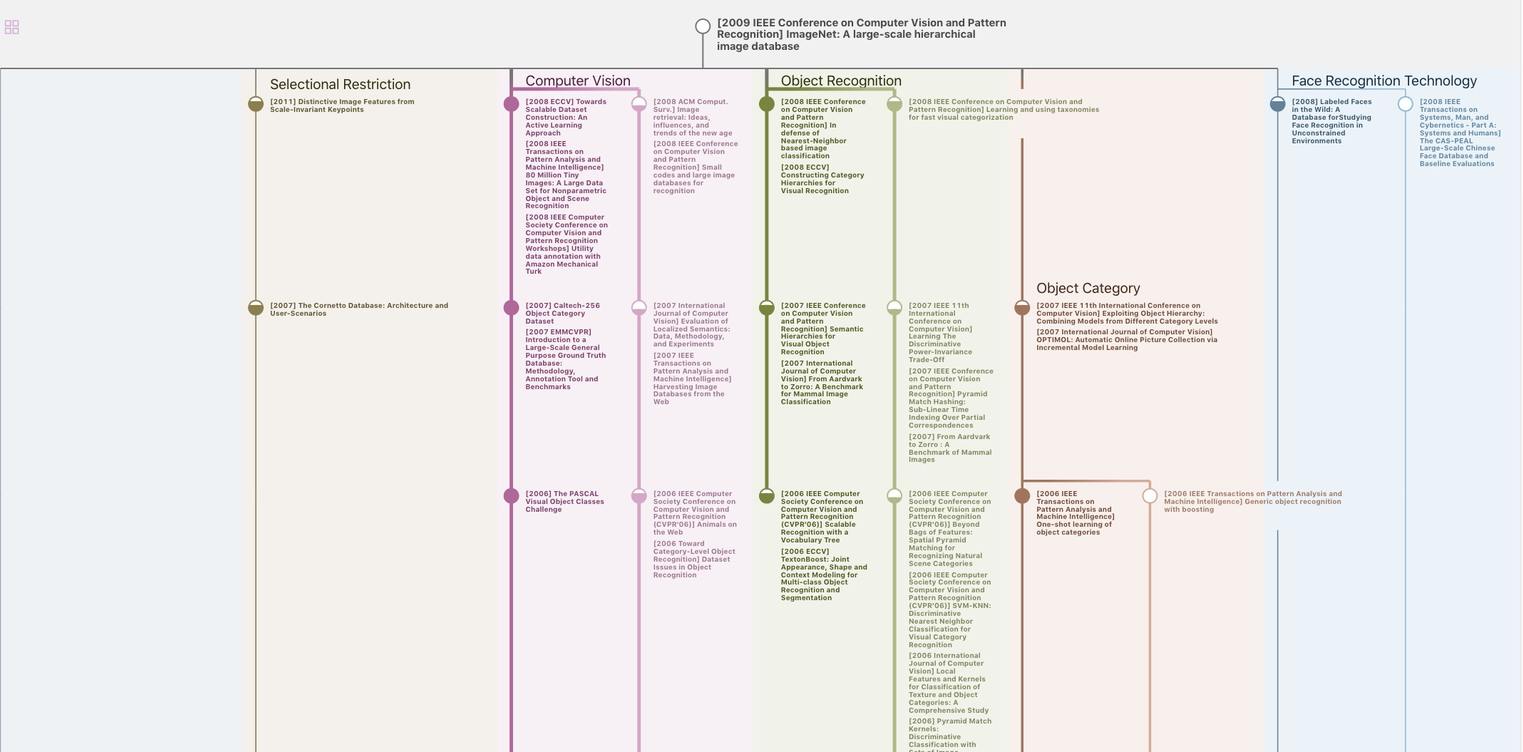
生成溯源树,研究论文发展脉络
Chat Paper
正在生成论文摘要