Bayesian nonparametric clustering for large data sets
Statistics and Computing(2018)
摘要
We propose two nonparametric Bayesian methods to cluster big data and apply them to cluster genes by patterns of gene–gene interaction. Both approaches define model-based clustering with nonparametric Bayesian priors and include an implementation that remains feasible for big data. The first method is based on a predictive recursion which requires a single cycle (or few cycles) of simple deterministic calculations for each observation under study. The second scheme is an exact method that divides the data into smaller subsamples and involves local partitions that can be determined in parallel. In a second step, the method requires only the sufficient statistics of each of these local clusters to derive global clusters. Under simulated and benchmark data sets the proposed methods compare favorably with other clustering algorithms, including k -means, DP-means, DBSCAN, SUGS, streaming variational Bayes and an EM algorithm. We apply the proposed approaches to cluster a large data set of gene–gene interactions extracted from the online search tool “Zodiac.”
更多查看译文
关键词
Big data clustering, Gene–gene interactions, Predictive recursion, Nonparametric Bayes, TCGA
AI 理解论文
溯源树
样例
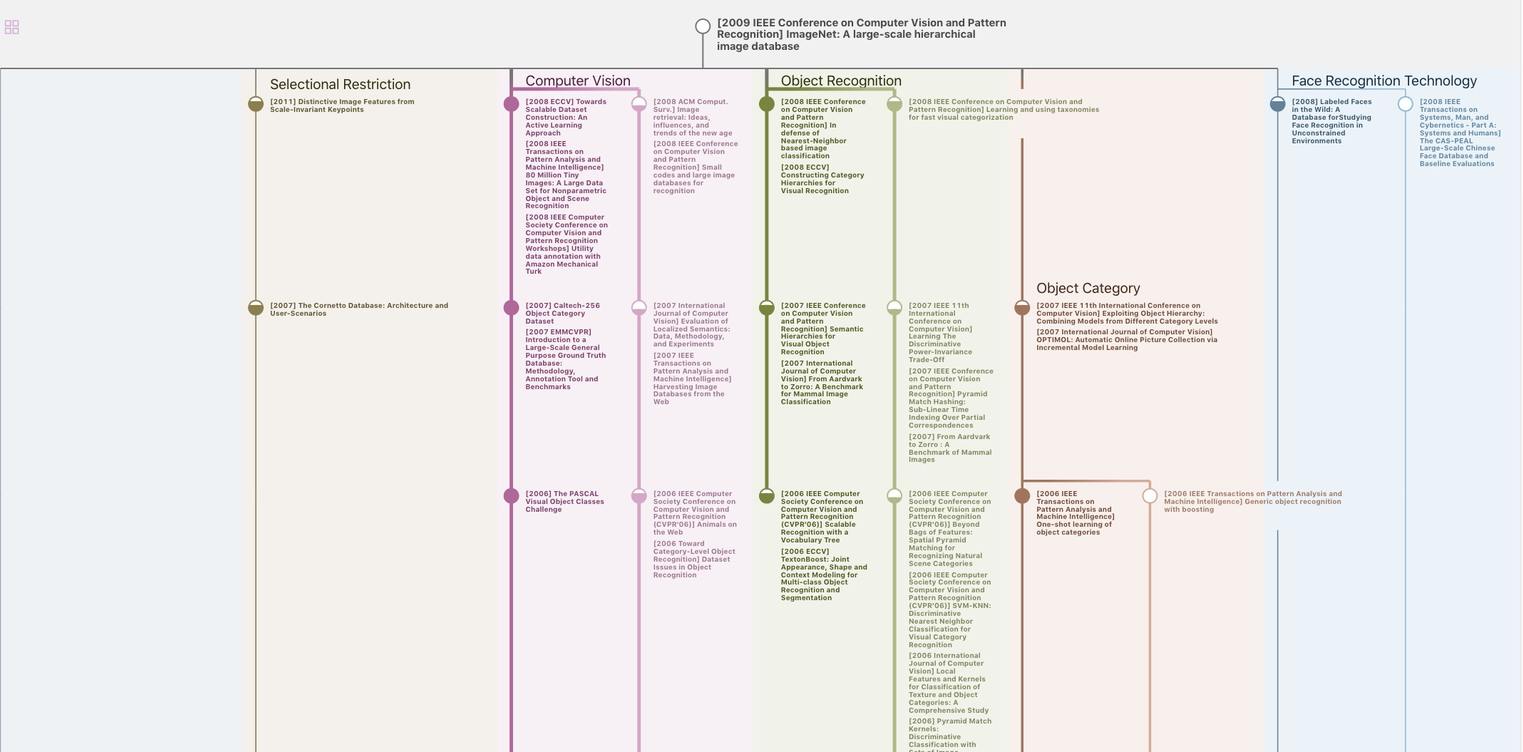
生成溯源树,研究论文发展脉络
Chat Paper
正在生成论文摘要