A genetic programming-based approach and machine learning approaches to the classification of multiclass anti-malarial datasets.
I. J. Computational Biology and Drug Design(2018)
Abstract
Feature selection approaches have been widely applied to deal with the various sample size problem in the classification of activity of datasets. The present work focuses on the understanding system of descriptors of anti-malarial inhibitors by Genetic programming (GP) to understand the impact of descriptors on inhibitory effects. The experimental dataset of inhibitors of anti-malarial was used to derive the optimised system by GP. Additionally, we have developed machine learning models using the random forest, decision tree, support vector machine (SVM) and Naive Bayes on an antimalarial dataset obtained from ChEMBL database and evaluated for their predictive capability. Based on the statistical evaluation, Random Forest model showed the higher area under the curve (AUC), better accuracy, sensitivity, and specificity in the cross-validation tests as compared to others. The statistical results indicated that the RF model was the best predictive model with 82.51% accuracy, 89.7% ROC. We deployed the RF cla...
MoreTranslated text
Key words
classification,programming-based,anti-malarial
AI Read Science
Must-Reading Tree
Example
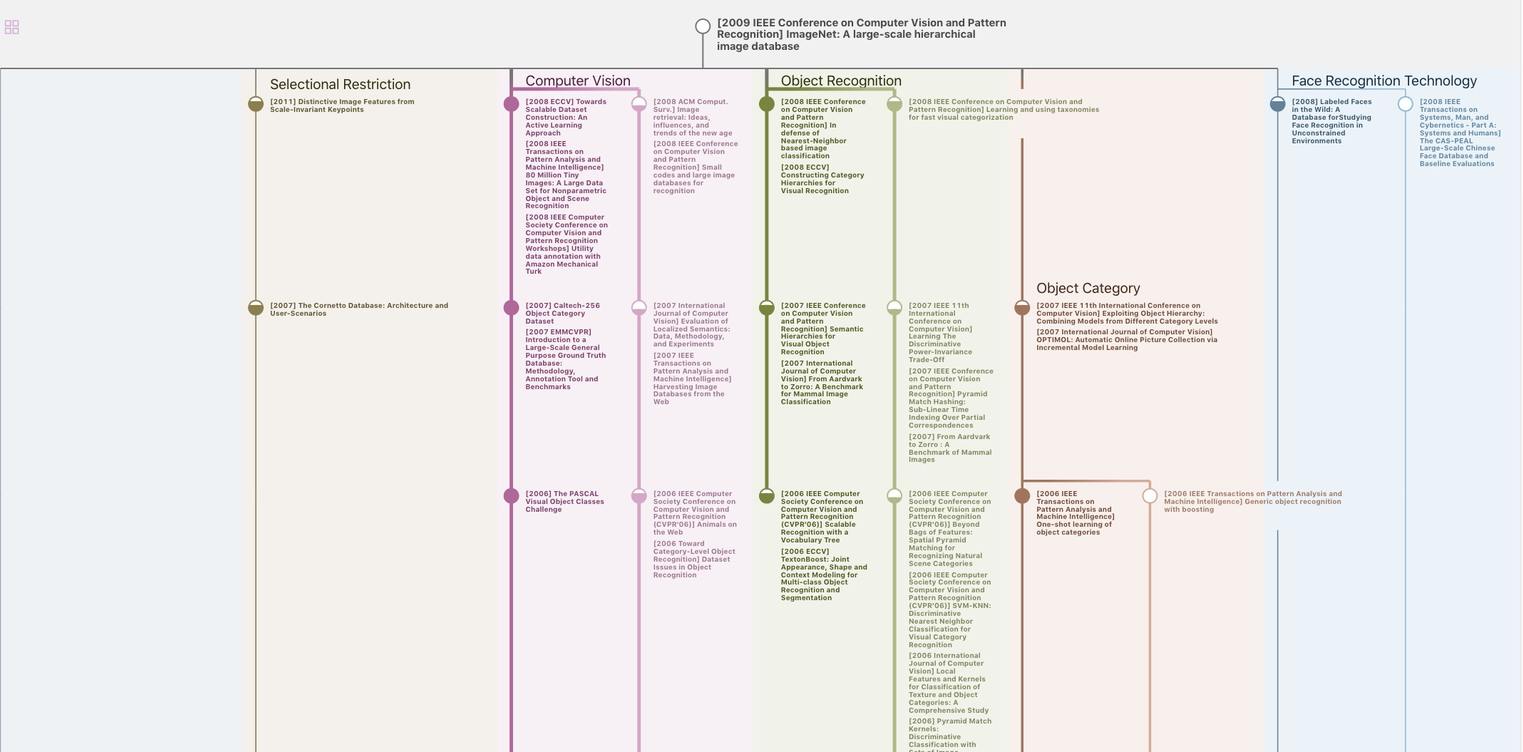
Generate MRT to find the research sequence of this paper
Chat Paper
Summary is being generated by the instructions you defined