Class-specific synthesized dictionary model for Zero-Shot Learning.
Neurocomputing(2019)
摘要
Zero-Shot Learning (ZSL) aims at recognizing unseen classes that are absent during the training stage. Unlike the existing approaches that learn a visual-semantic embedding model to bridge the low-level visual space and the high-level class prototype space, we propose a novel synthesized approach for addressing ZSL within a dictionary learning framework. Specifically, it learns both a dictionary matrix and a class-specific encoding matrix for each seen class to synthesize pseudo instances for unseen classes with auxiliary of seen class prototypes. This allows us to train the classifiers for the unseen classes with these pseudo instances. In this way, ZSL can be treated as a traditional classification task, which makes it applicable for traditional and generalized ZSL settings simultaneously. Extensive experimental results on four benchmark datasets (AwA, CUB, aPY, and SUN) demonstrate that our method yields competitive performances compared to state-of-the-art methods on both settings.
更多查看译文
关键词
Zero-Shot Learning,Dictionary learning,Image recognition,Synthesized model
AI 理解论文
溯源树
样例
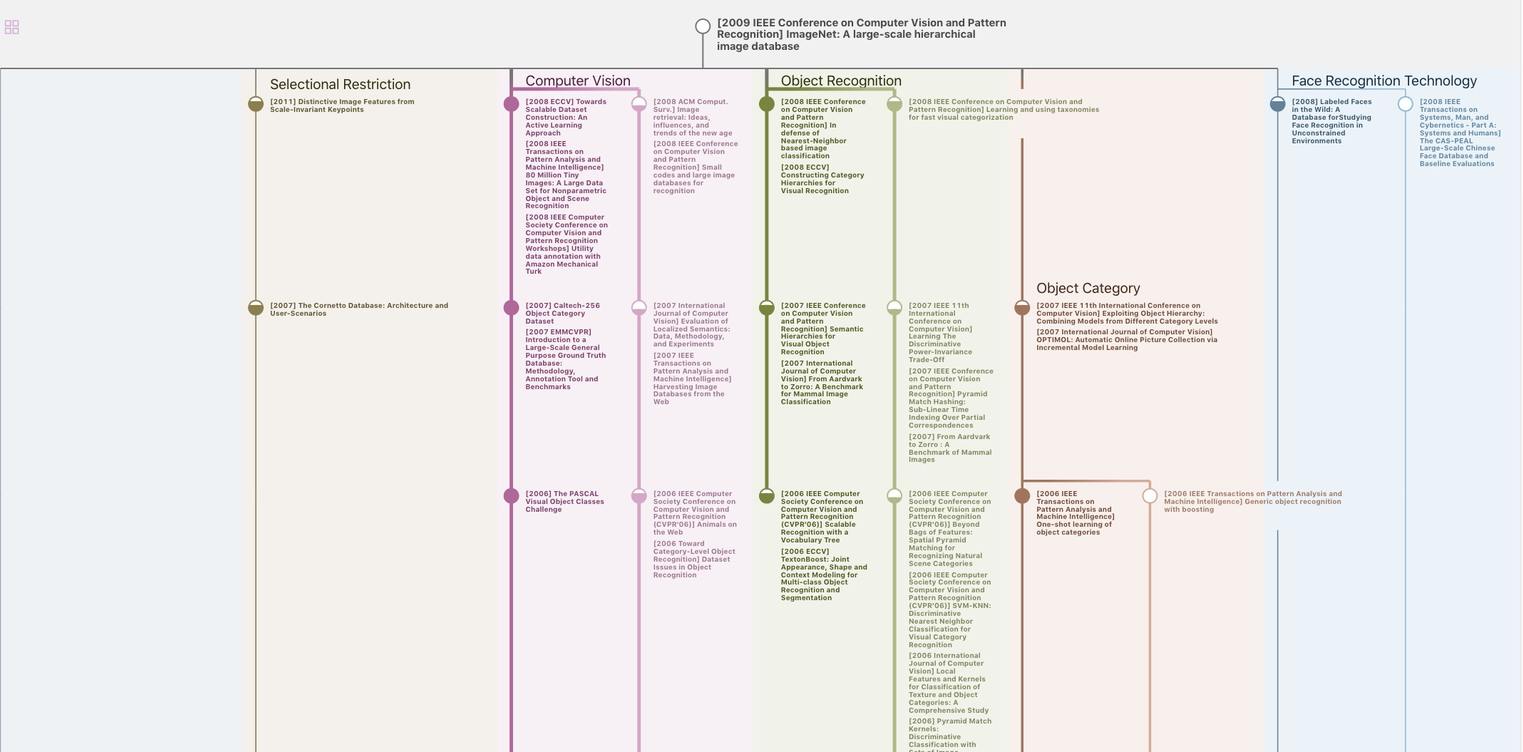
生成溯源树,研究论文发展脉络
Chat Paper
正在生成论文摘要