Adaptive Cross-Modal Few-Shot Learning
ADVANCES IN NEURAL INFORMATION PROCESSING SYSTEMS 32 (NIPS 2019)(2019)
摘要
Metric-based meta-learning techniques have successfully been applied to few-shot classification problems. In this paper, we propose to leverage cross-modal information to enhance metric-based few-shot learning methods. Visual and semantic feature spaces have different structures by definition. For certain concepts, visual features might be richer and more discriminative than text ones. While for others, the inverse might be true. Moreover, when the support from visual information is limited in image classification, semantic representations (learned from unsupervised text corpora) can provide strong prior knowledge and context to help learning. Based on these two intuitions, we propose a mechanism that can adaptively combine information from both modalities according to new image categories to be learned. Through a series of experiments, we show that by this adaptive combination of the two modalities, our model outperforms current uni-modality few-shot learning methods and modality-alignment methods by a large margin on all benchmarks and few-shot scenarios tested. Experiments also show that our model can effectively adjust its focus on the two modalities. The improvement in performance is particularly large when the number of shots is very small.
更多查看译文
关键词
image classification
AI 理解论文
溯源树
样例
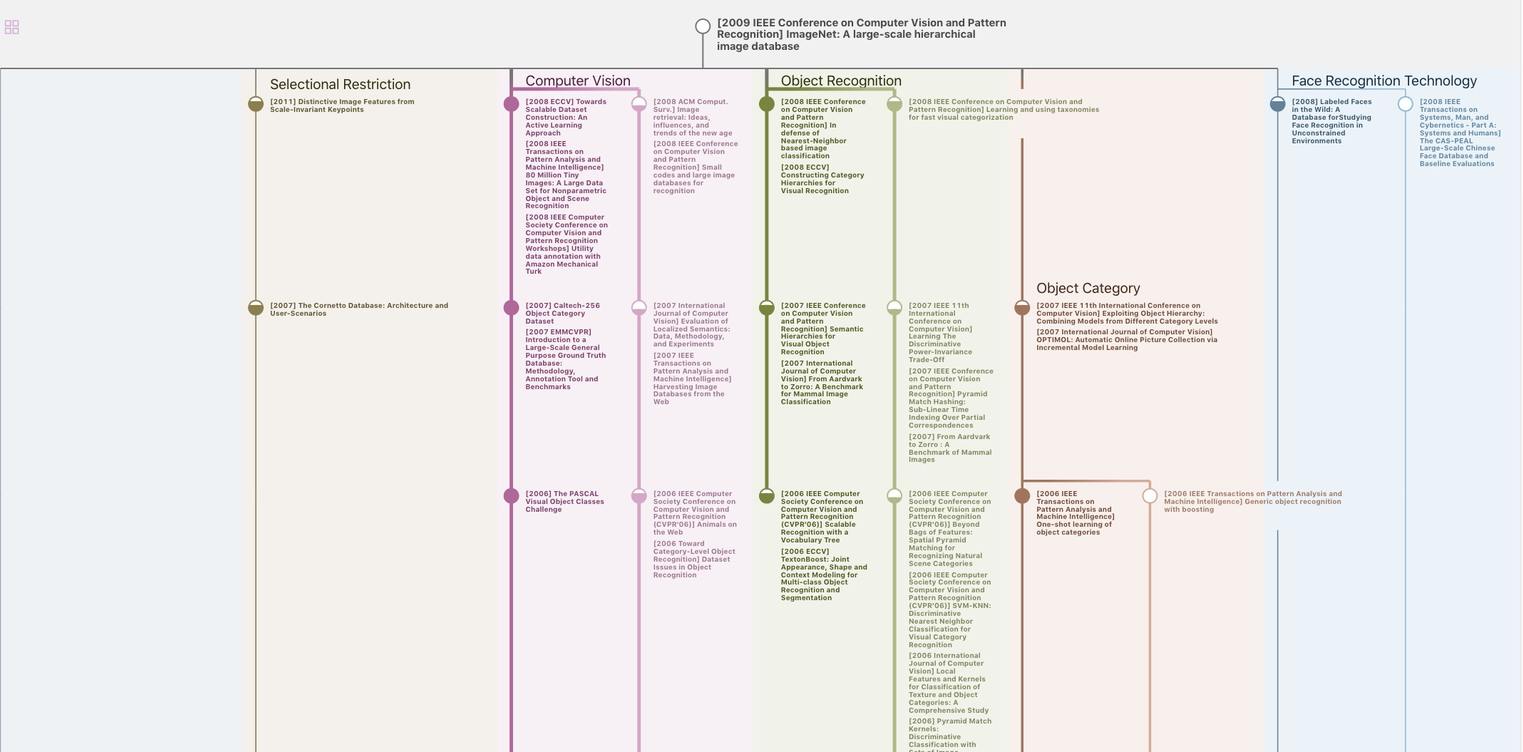
生成溯源树,研究论文发展脉络
Chat Paper
正在生成论文摘要