The Use Of Component-Wise Gradient Boosting To Assess The Possible Role Of Cognitive Measures As Markers Of Vulnerability To Pediatric Bipolar Disorder
COGNITIVE NEUROPSYCHIATRY(2019)
摘要
Background and Aims Cognitive impairments are primary hallmarks symptoms of bipolar disorder (BD). Whether these deficits are markers of vulnerability or symptoms of the disease is still unclear. This study used a component-wise gradient (CGB) machine learning algorithm to identify cognitive measures that could accurately differentiate pediatric BD, unaffected offspring of BD parents, and healthy controls. Methods 59 healthy controls (HC; 11.19 +/- 3.15 yo; 30 girls), 119 children and adolescents with BD (13.31 +/- 3.02 yo, 52 girls) and 49 unaffected offspring of BD parents (UO; 9.36 +/- 3.18 yo; 22 girls) completed the CANTAB cognitive battery. Results CGB achieved accuracy of 73.2% and an AUROC of 0.785 in classifying individuals as either BD or non-BD on a dataset held out for validation for testing. The strongest cognitive predictors of BD were measures of processing speed and affective processing. Measures of cognition did not differentiate between UO and HC. Conclusions Alterations in processing speed and affective processing are markers of BD in pediatric populations. Longitudinal studies should determine whether UO with a cognitive profile similar to that of HC are at less or equal risk for mood disorders. Future studies should include relevant measures for BD such as verbal memory and genetic risk scores.
更多查看译文
关键词
Bipolar disorder,high-risk,machine learning,CANTAB,cognitive
AI 理解论文
溯源树
样例
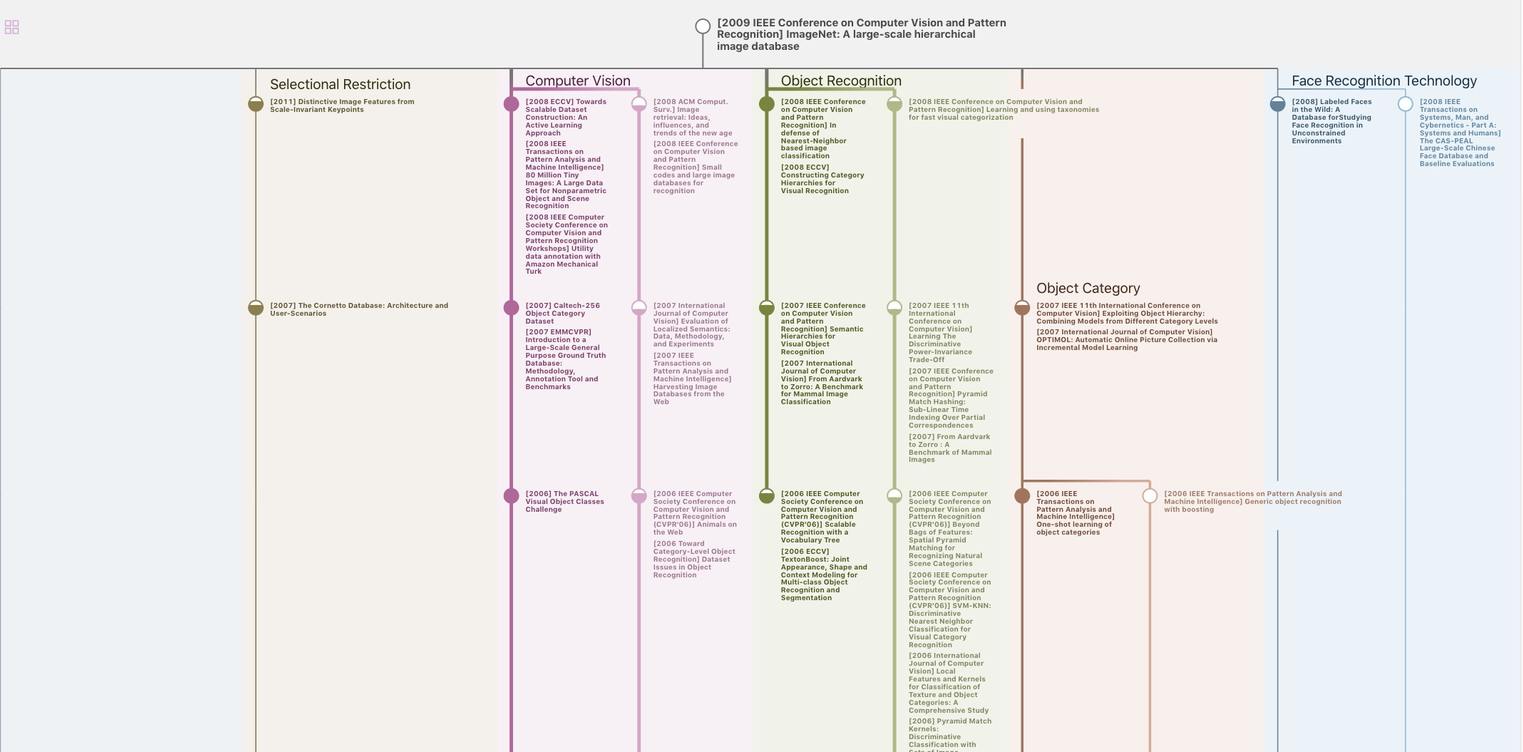
生成溯源树,研究论文发展脉络
Chat Paper
正在生成论文摘要