A Comparative Analysis of Unsupervised Machine Techniques for Liver Disease Prediction
2018 IEEE International Symposium on Signal Processing and Information Technology (ISSPIT)(2018)
摘要
Machine learning is a branch of Artificial Intelligence(AI) which is heavily used in the field of data science. It has a strong potential in health-related data analysis for automated disease prediction. The work focuses on three different machine learning techniques, i.e., DBSCAN, K-Means, and Affinity Propagation to compare their prediction accuracy and computational complexity. The study concentrates on liver disease-related health care data set and uses the Silhouette coefficient for comparative performance measurement of the three techniques mentioned above. The Silhouette coefficient determines prediction accuracy giving K-Means as the optimal method. The overall results will then be analyzed on the basis of prediction accuracy and computational complexity to determine the best technique for prediction of liver diseases using unsupervised machine learning.
更多查看译文
关键词
Machine learning,Clustering algorithms,Liver diseases,Neural networks,Cancer
AI 理解论文
溯源树
样例
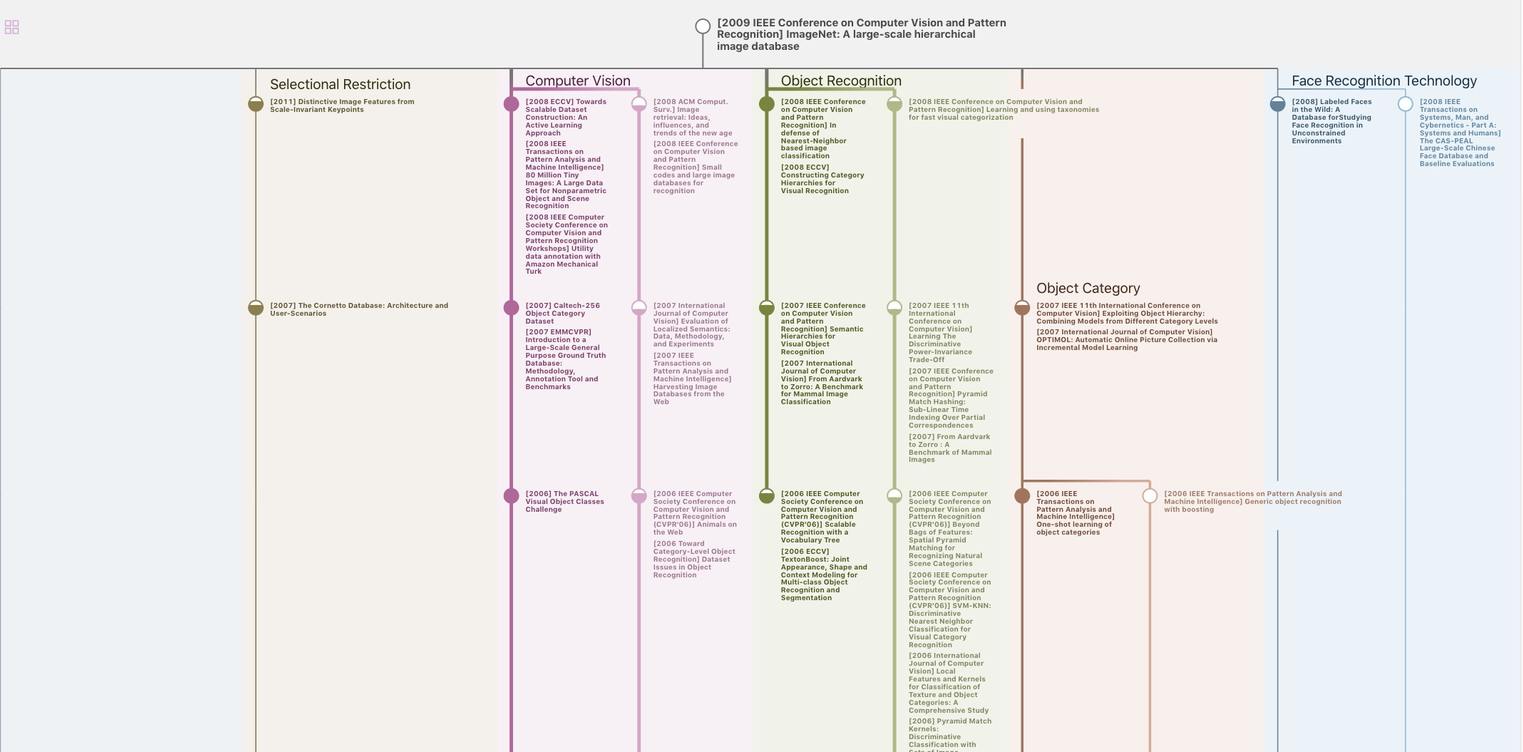
生成溯源树,研究论文发展脉络
Chat Paper
正在生成论文摘要