Personalized Emotion Recognition by Personality-Aware High-Order Learning of Physiological Signals
ACM Transactions on Multimedia Computing, Communications, and Applications (TOMM)(2019)
摘要
Due to the subjective responses of different subjects to physical stimuli, emotion recognition methodologies from physiological signals are increasingly becoming personalized. Existing works mainly focused on modeling the involved physiological corpus of each subject, without considering the psychological factors, such as interest and personality. The latent correlation among different subjects has also been rarely examined. In this article, we propose to investigate the influence of personality on emotional behavior in a hypergraph learning framework. Assuming that each vertex is a compound tuple (subject, stimuli), multi-modal hypergraphs can be constructed based on the personality correlation among different subjects and on the physiological correlation among corresponding stimuli. To reveal the different importance of vertices, hyperedges, and modalities, we learn the weights for each of them. As the hypergraphs connect different subjects on the compound vertices, the emotions of multiple subjects can be simultaneously recognized. In this way, the constructed hypergraphs are vertex-weighted multi-modal multi-task ones. The estimated factors, referred to as emotion relevance, are employed for emotion recognition. We carry out extensive experiments on the ASCERTAIN dataset and the results demonstrate the superiority of the proposed method, as compared to the state-of-the-art emotion recognition approaches.
更多查看译文
关键词
Personalized emotion recognition, hypergraph learning, multi-modal fusion, personality-sensitive learning, physiological signal analysis
AI 理解论文
溯源树
样例
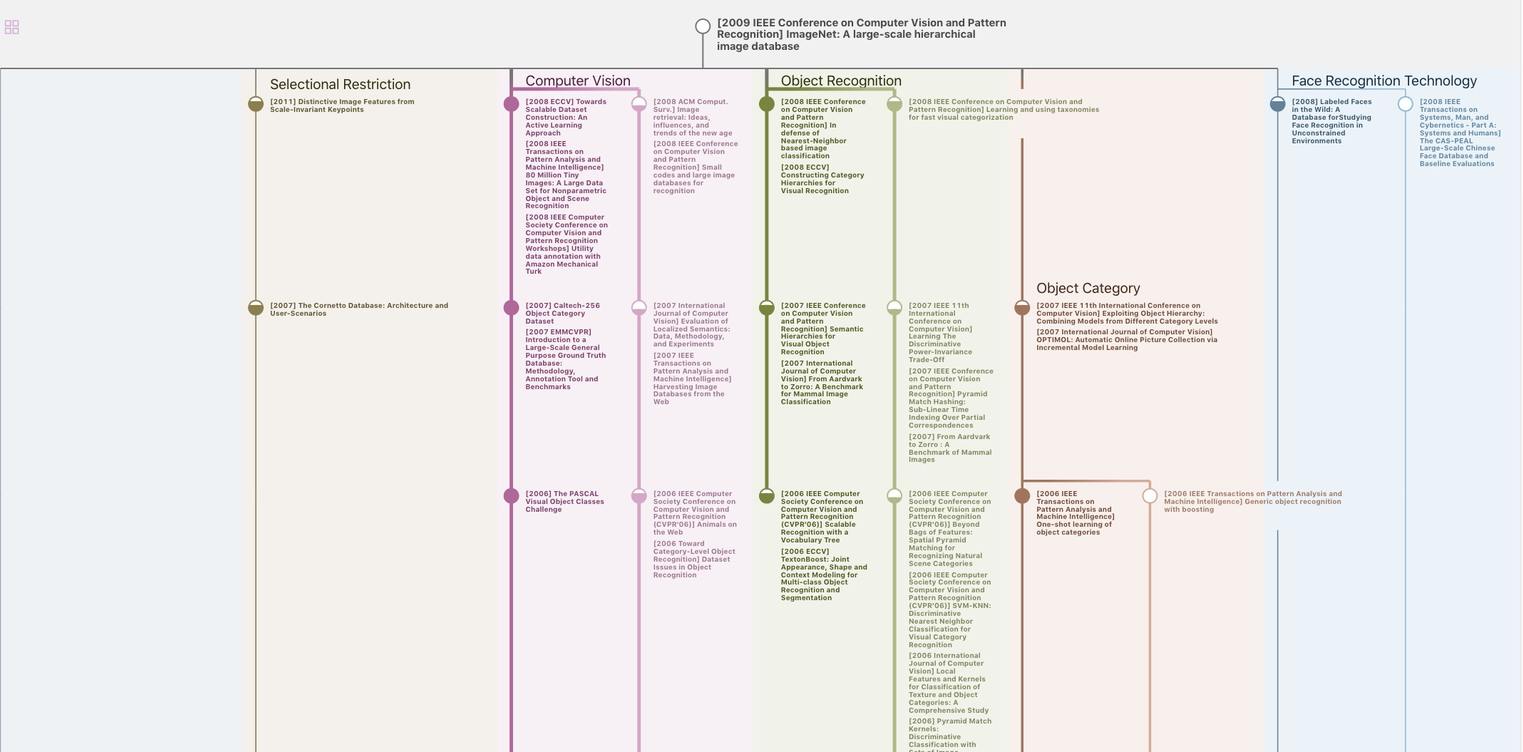
生成溯源树,研究论文发展脉络
Chat Paper
正在生成论文摘要