Deep View2View Mapping for View-Invariant Lipreading.
SLT(2018)
Abstract
Recently, visual-only and audio-visual speech recognition have made significant progress thanks to deep-learning based, trainable visual front-ends (VFEs), with most research focusing on frontal or near-frontal face videos. In this paper, we seek to expand the applicability of VFEs targeted on frontal face views to non-frontal ones, without making assumptions on the VFE type, and allowing systems trained on frontal-view data to be applied on mismatched, non-frontal videos. For this purpose, we adapt the “pix2pix” model, recently proposed for image translation tasks, to transform non-frontal speaker mouth regions to frontal, employing a convolutional neural network architecture, which we call “view2view”. We develop our approach on the OuluVS2 multiview lipreading dataset, allowing training of four such networks that map views at predefined non-frontal angles (up to profile) to frontal ones, which we subsequently feed to a frontal-view VFE. We compare the “view2view” network against a baseline that performs linear cross-view regression at the VFE space. Results on visual-only, as well as audio-visual automatic speech recognition over multiple acoustic noise conditions, demonstrate that the “view2view” significantly outperforms the baseline, narrowing the performance gap from an ideal, matched scenario of view-specific systems. Improvements are retained when the approach is coupled with an automatic view estimator.
MoreTranslated text
Key words
Speech recognition,Visualization,Feature extraction,Decoding,Videos,Convolution,Face
AI Read Science
Must-Reading Tree
Example
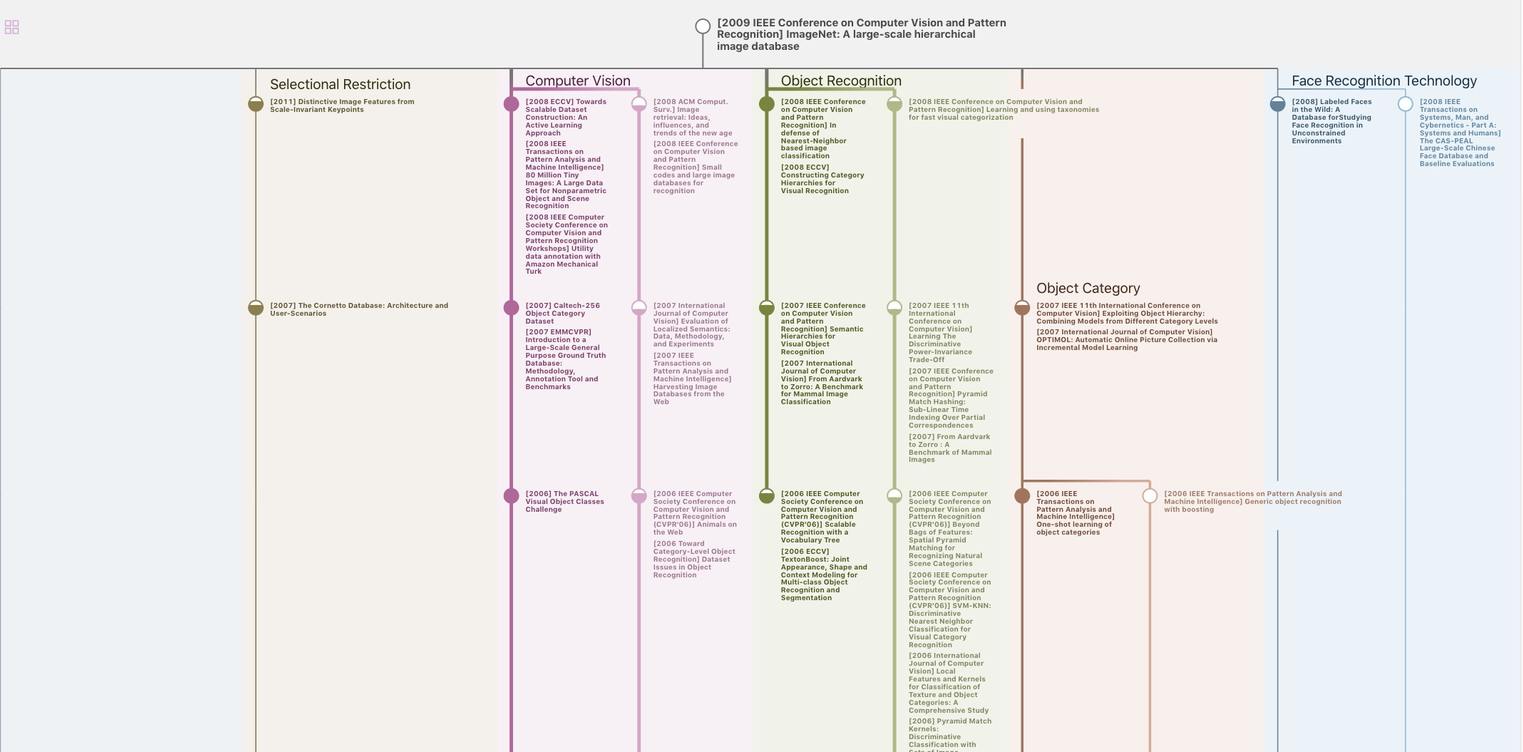
Generate MRT to find the research sequence of this paper
Chat Paper
Summary is being generated by the instructions you defined