Photorealistic Image Synthesis for Object Instance Detection
2019 IEEE International Conference on Image Processing (ICIP)(2019)
Abstract
We present an approach to synthesize highly photorealistic images of 3D object models, which we use to train a convolutional neural network for detecting the objects in real images. The proposed approach has three key ingredients: (1) 3D object models are rendered in 3D models of complete scenes with realistic materials and lighting, (2) plausible geometric configuration of objects and cameras in a scene is generated using physics simulation, and (3) high photorealism of the synthesized images is achieved by physically based rendering. When trained on images synthesized by the proposed approach, the Faster R-CNN object detector [1] achieves a 24% absolute improvement of mAP@.75IoU on Rutgers APC [2] and 11% on LineMod-Occluded [3] datasets, compared to a baseline where the training images are synthesized by rendering object models on top of random photographs. This work is a step towards being able to effectively train object detectors without capturing or annotating any real images. A dataset of 400K synthetic images with ground truth annotations for various computer vision tasks will be released on the project website: thodan.github.io/objectsynth.
MoreTranslated text
Key words
photorealistic image synthesis,object instance detection,highly photorealistic images,object models,convolutional neural network,physically based rendering,R-CNN object detector,training images,synthetic images,LineMod-Occluded datasets,random photographs,computer vision tasks,ground truth annotations,temperature 400.0 K
AI Read Science
Must-Reading Tree
Example
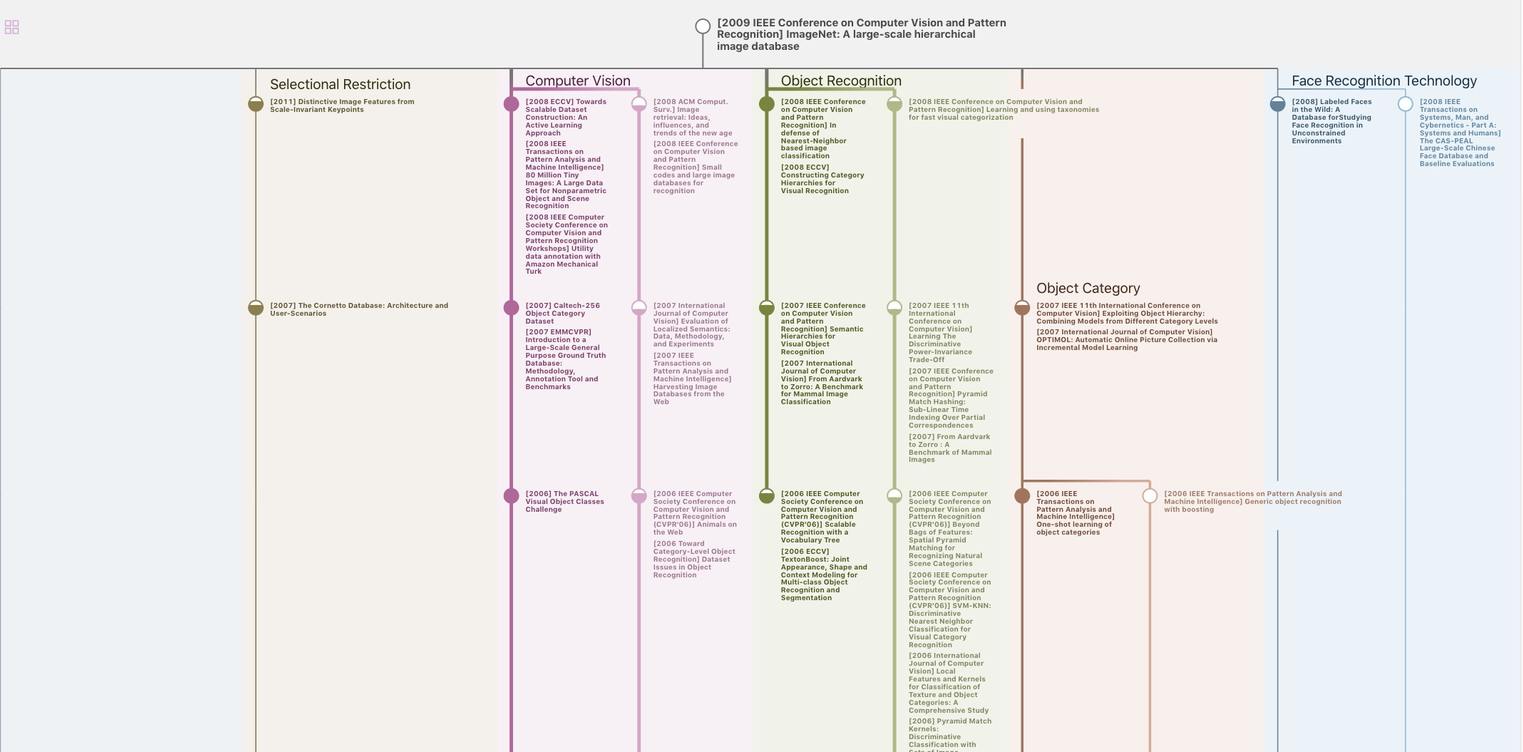
Generate MRT to find the research sequence of this paper
Chat Paper
Summary is being generated by the instructions you defined