Compressed Diffusion
2019 13th International conference on Sampling Theory and Applications (SampTA)(2019)
Abstract
Diffusion maps are a commonly used kernel-based method for manifold learning, which can reveal intrinsic structures in data and embed them in low dimensions. However, as with most kernel methods, its implementation requires a heavy computational load, reaching up to cubic complexity in the number of data points. This limits its usability in modern data analysis. Here, we present a new approach to computing the diffusion geometry, and related embeddings, from a compressed diffusion process between data regions rather than data points. Our construction is based on an adaptation of the previously proposed measure-based Gaussian correlation (MGC) kernel that robustly captures the local geometry around data points. We use this MGC kernel to efficiently compress diffusion relations from pointwise to data region resolution. Finally, a spectral embedding of the data regions provides coordinates that are used to interpolate and approximate the pointwise diffusion map embedding of data. We analyze theoretical connections between our construction and the original diffusion geometry of diffusion maps, and demonstrate the utility of our method in analyzing big datasets, where it outperforms competing approaches.
MoreTranslated text
Key words
data points,data analysis,compressed diffusion process,data regions,measure-based Gaussian correlation kernel,MGC kernel,diffusion relations,data region resolution,diffusion geometry,pointwise diffusion map embedding,manifold learning
AI Read Science
Must-Reading Tree
Example
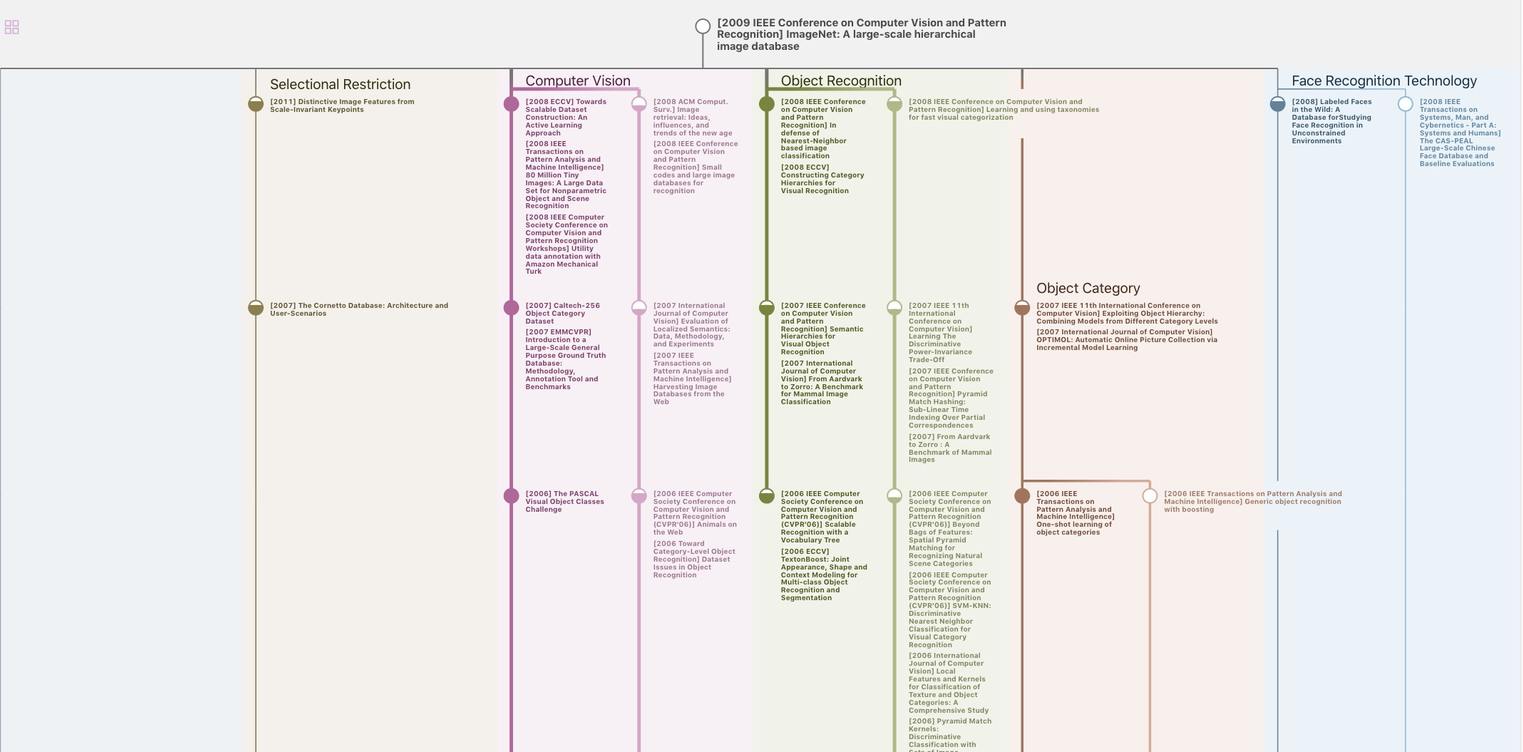
Generate MRT to find the research sequence of this paper
Chat Paper
Summary is being generated by the instructions you defined