Overcomplete Independent Component Analysis Via Sdp
22ND INTERNATIONAL CONFERENCE ON ARTIFICIAL INTELLIGENCE AND STATISTICS, VOL 89(2019)
摘要
We present a novel algorithm for over-complete independent components analysis (ICA), where the number of latent sources k exceeds the dimension p of observed variables. Previous algorithms either suffer from high computational complexity or make strong assumptions about the form of the mixing matrix. Our algorithm does not make any sparsity assumption yet enjoys favorable computational and theoretical properties. Our algorithm consists of two main steps: (a) estimation of the Hessians of the cumulant generating function (as opposed to the fourth and higher order cumulants used by most algorithms) and (b) a novel semi-definite programming (SDP) relaxation for recovering a mixing component. We show that this relaxation can be efficiently solved with a projected accelerated gradient descent method, which makes the whole algorithm computationally practical. Moreover, we conjecture that the proposed program recovers a mixing component at the rate k < p(2)/4 and prove that a mixing component can be recovered with high probability when k < (2 - epsilon)p log p when the original components are sampled uniformly at random on the hypersphere. Experiments are provided on synthetic data and the CIFAR-10 dataset of real images.
更多查看译文
AI 理解论文
溯源树
样例
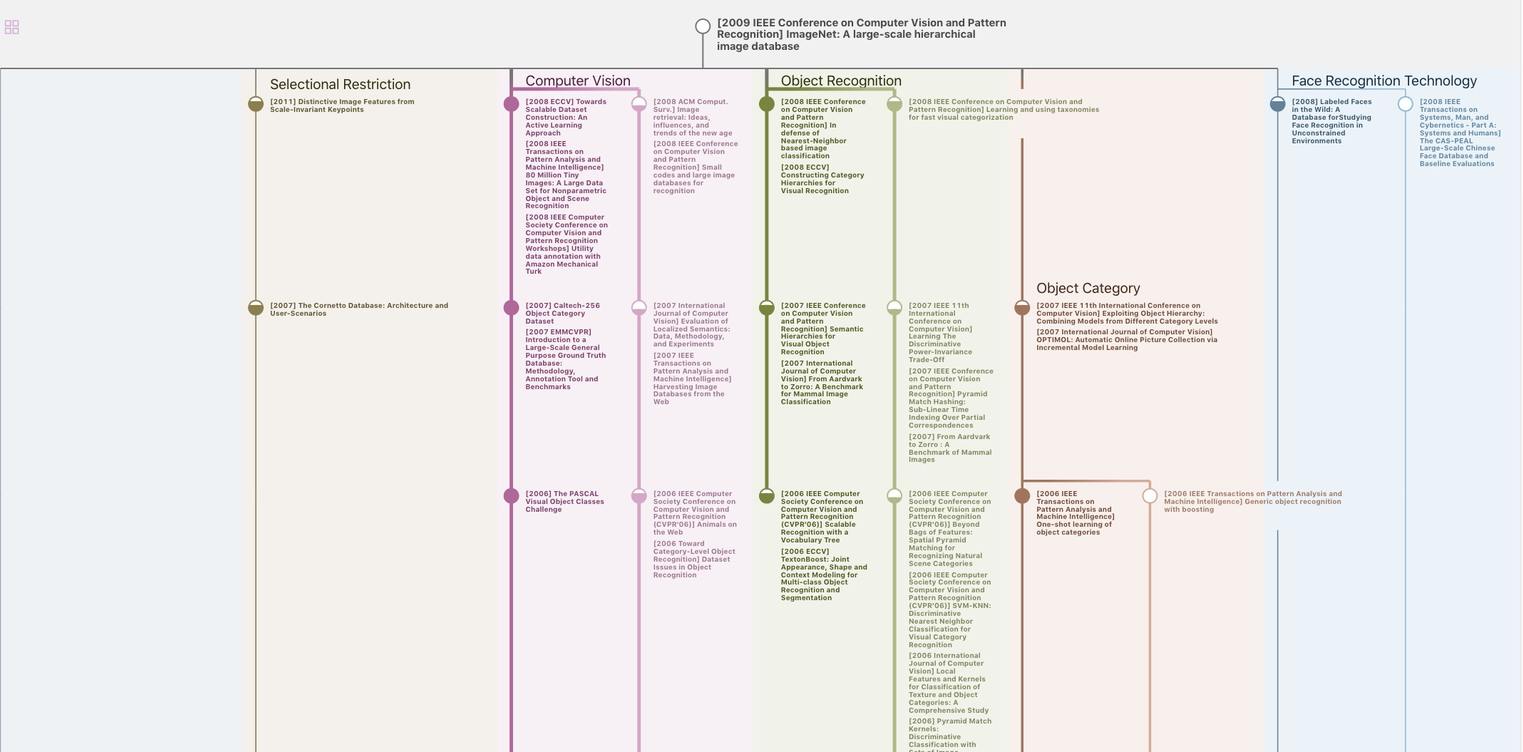
生成溯源树,研究论文发展脉络
Chat Paper
正在生成论文摘要