Uncertain Photometric Redshifts
arxiv(2016)
摘要
Photometric redshifts play an important role as a measure of distance for various cosmological topics. Spectroscopic redshifts are only available for a very limited number of objects but can be used for creating statistical models. A broad variety of photometric catalogues provide uncertain low resolution spectral information for galaxies and quasars that can be used to infer a redshift. Many different techniques have been developed to produce those redshift estimates with increasing precision. Instead of providing a point estimate only, astronomers start to generate probabilistic density functions (PDFs) which should provide a characterisation of the uncertainties of the estimation. In this work we present two simple approaches on how to generate those PDFs. We use the example of generating the photometric redshift PDFs of quasars from SDSS(DR7) to validate our approaches and to compare them with point estimates. We do not aim for presenting a new best performing method, but we choose an intuitive approach that is based on well known machine learning algorithms. Furthermore we introduce proper tools for evaluating the performance of PDFs in the context of astronomy. The continuous ranked probability score (CRPS) and the probability integral transform (PIT) are well accepted in the weather forecasting community. Both tools reflect how well the PDFs reproduce the real values of the analysed objects. As we show, nearly all currently used measures in astronomy show severe weaknesses when used to evaluate PDFs.
更多查看译文
AI 理解论文
溯源树
样例
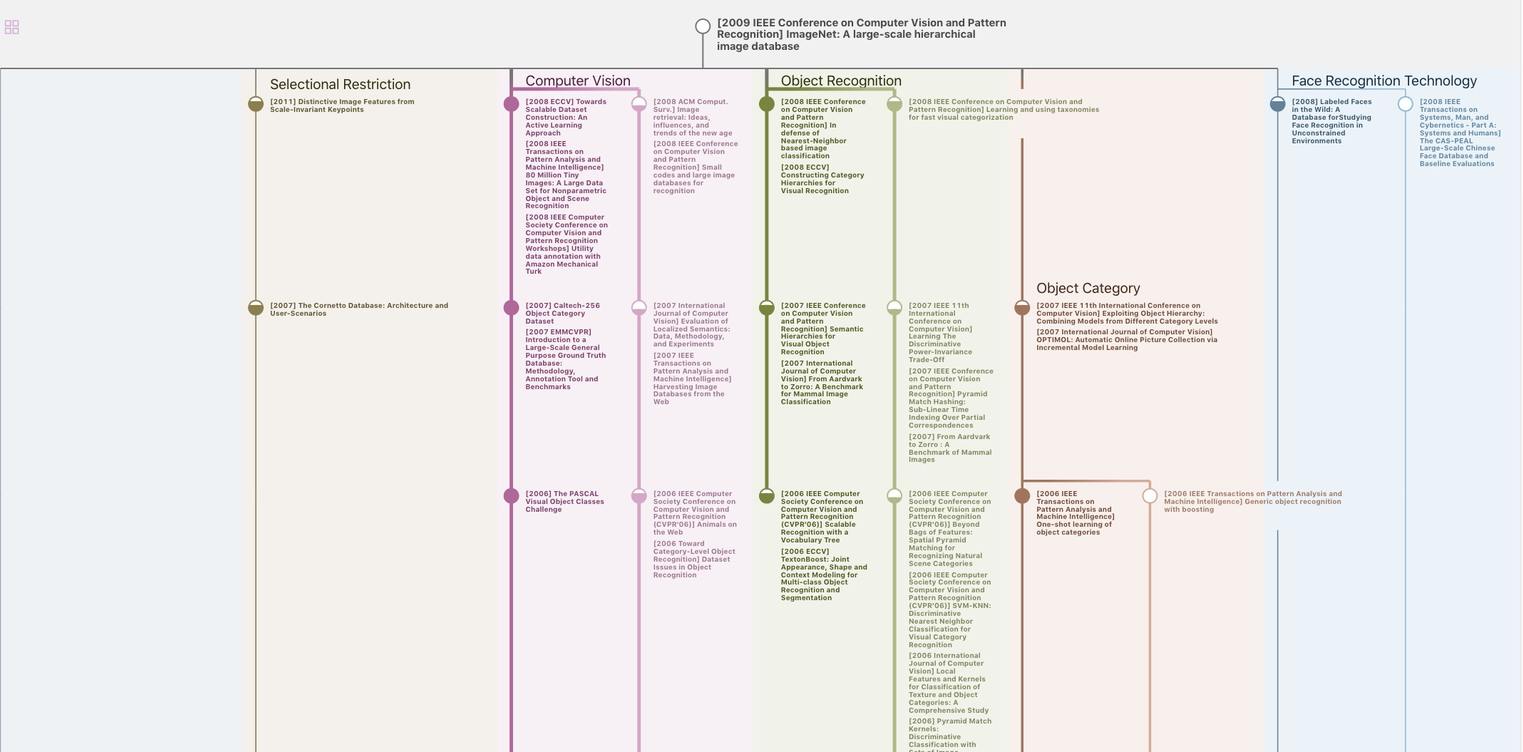
生成溯源树,研究论文发展脉络
Chat Paper
正在生成论文摘要