Learning Summary Statistic for Approximate Bayesian Computation via Deep Neural Network
Statistica Sinica(2018)
摘要
Approximate Bayesian Computation (ABC) methods are used to approximate posterior distributions in models with unknown or computationally intractable likelihoods. Both the accuracy and computational efficiency of ABC depend on the choice of summary statistic, but outside of special cases where the optimal summary statistics are known, it is unclear which guiding principles can be used to construct effective summary statistics. In this paper we explore the possibility of automating the process of constructing summary statistics by training deep neural networks to predict the parameters from artificially generated data: the resulting summary statistics are approximately posterior means of the parameters. With minimal model-specific tuning, our method constructs summary statistics for the Ising model and the moving-average model, which match or exceed theoretically-motivated summary statistics in terms of the accuracies of the resulting posteriors.
更多查看译文
关键词
Approximate Bayesian computation,deep learning,summary statistic
AI 理解论文
溯源树
样例
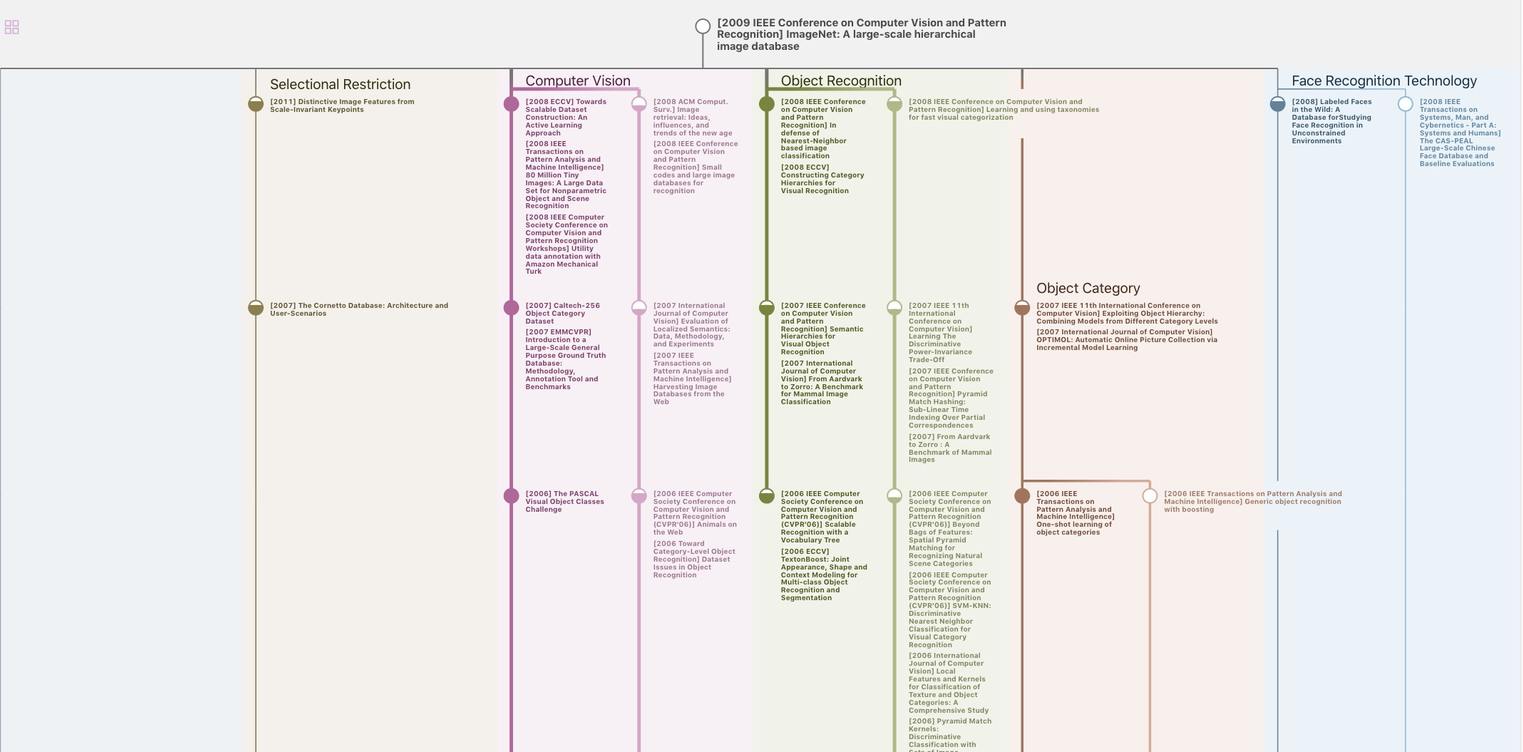
生成溯源树,研究论文发展脉络
Chat Paper
正在生成论文摘要