On-line Application Autotuning Exploiting Ensemble Models.
arXiv: Distributed, Parallel, and Cluster Computing(2019)
摘要
Application autotuning is a promising path investigated in literature to improve computation efficiency. In this context, the end-users define high-level requirements and an autonomic manager is able to identify and seize optimization opportunities by leveraging trade-offs between extra-functional properties of interest, such as execution time, power consumption or quality of results. The relationship between an application configuration and the extra-functional properties might depend on the underlying architecture, on the system workload and on features of the current input. For these reasons, autotuning frameworks rely on application knowledge to drive the adaptation strategies. The autotuning task is typically done offline because having it in production requires significant effort to reduce its overhead. In this paper, we enhance a dynamic autotuning framework with a module for learning the application knowledge during the production phase, in a distributed fashion. We leverage two strategies to limit the overhead introduced at the production phase. On one hand, we use a scalable infrastructure capable of leveraging the parallelism of the underlying platform. On the other hand, we use ensemble models to speed up the predictive capabilities, while iteratively gathering production data. Experimental results on synthetic applications and on a use case show how the proposed approach is able to learn the application knowledge, by exploring a small fraction of the design space.
更多查看译文
AI 理解论文
溯源树
样例
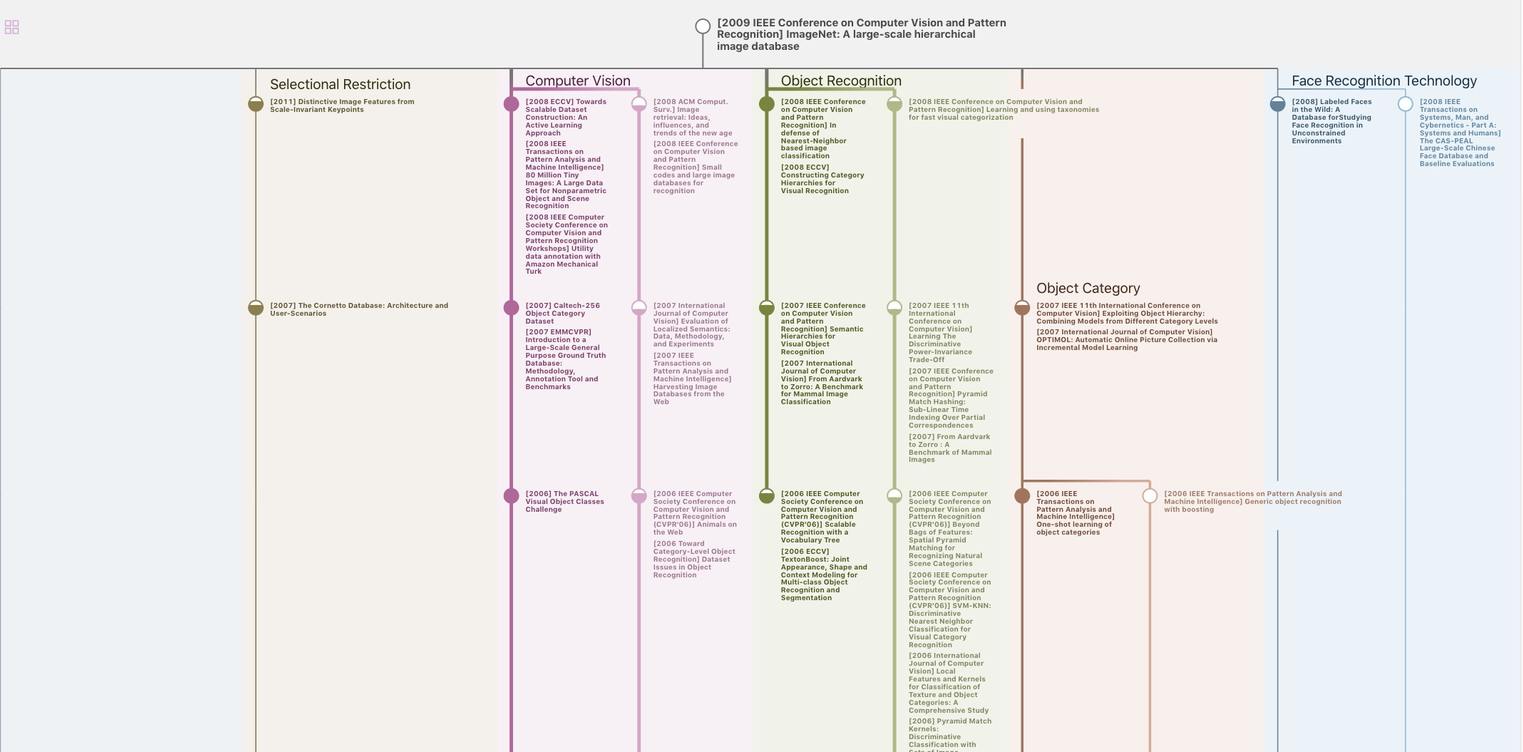
生成溯源树,研究论文发展脉络
Chat Paper
正在生成论文摘要