Large-scale Collaborative Filtering with Product Embeddings
arXiv.org(2019)
Abstract
The application of machine learning techniques to large-scale personalized recommendation problems is a challenging task. Such systems must make sense of enormous amounts of implicit feedback in order to understand user preferences across numerous product categories. This paper presents a deep learning based solution to this problem within the collaborative filtering with implicit feedback framework. Our approach combines neural attention mechanisms, which allow for context dependent weighting of past behavioral signals, with representation learning techniques to produce models which obtain extremely high coverage, can easily incorporate new information as it becomes available, and are computationally efficient. Offline experiments demonstrate significant performance improvements when compared to several alternative methods from the literature. Results from an online setting show that the approach compares favorably with current production techniques used to produce personalized product recommendations.
MoreTranslated text
AI Read Science
Must-Reading Tree
Example
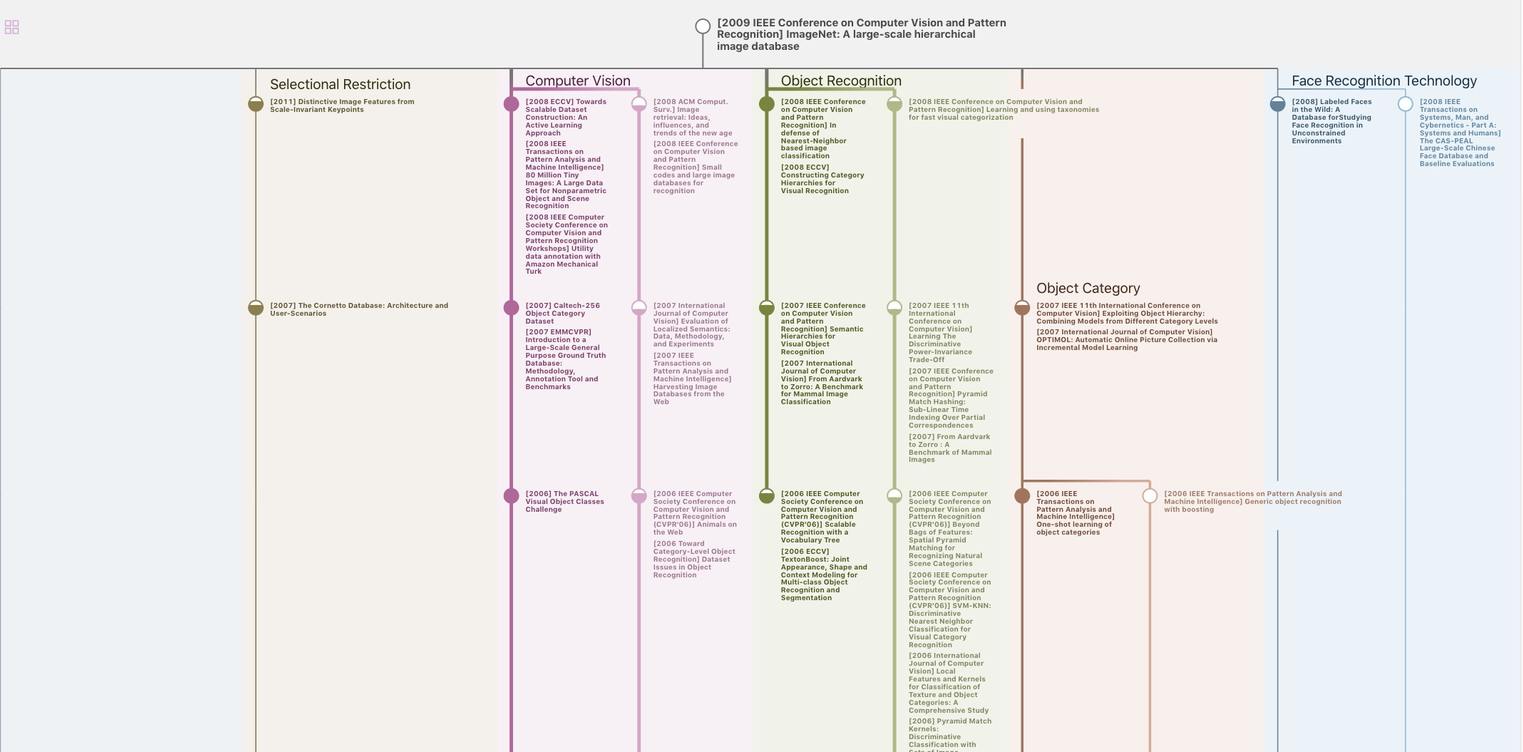
Generate MRT to find the research sequence of this paper
Chat Paper
Summary is being generated by the instructions you defined