Power Allocation in Multi-User Cellular Networks: Deep Reinforcement Learning Approaches
IEEE Transactions on Wireless Communications(2020)
摘要
The model-based power allocation has been investigated for decades, but this approach requires mathematical models to be analytically tractable and it has high computational complexity. Recently, the data-driven model-free approaches have been rapidly developed to achieve near-optimal performance with affordable computational complexity, and deep reinforcement learning (DRL) is regarded as one such approach having great potential for future intelligent networks. In this paper, a dynamic downlink power control problem is considered for maximizing the sum-rate in a multi-user wireless cellular network. Using cross-cell coordinations, the proposed multi-agent DRL framework includes off-line and on-line centralized training and distributed execution, and a mathematical analysis is presented for the top-level design of the near-static problem. Policy-based REINFORCE, value-based deep Q-learning (DQL), actor-critic deep deterministic policy gradient (DDPG) algorithms are proposed for this sum-rate problem. Simulation results show that the data-driven approaches outperform the state-of-art model-based methods on sum-rate performance. Furthermore, the DDPG outperforms the REINFORCE and DQL in terms of both sum-rate performance and robustness.
更多查看译文
关键词
Deep reinforcement learning,deep deterministic policy gradient,policy-based,interfering broadcast channel,power control,resource allocation
AI 理解论文
溯源树
样例
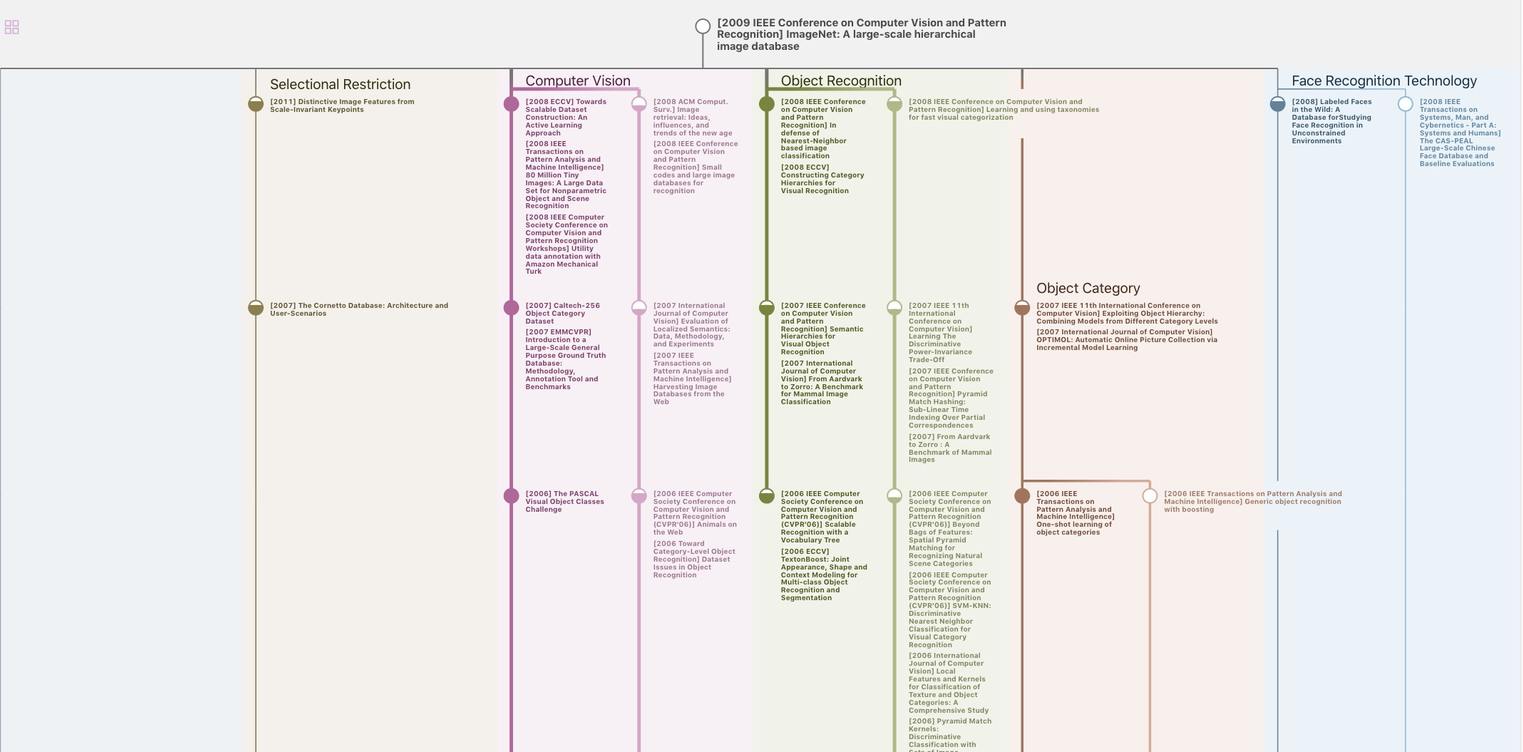
生成溯源树,研究论文发展脉络
Chat Paper
正在生成论文摘要