Hard Sample Mining and Learning for Skeleton-Based Human Action Recognition and Identification.
IEEE ACCESS(2019)
摘要
The analysis of human actions, such as recognition and person identification, is important but challenging. Previous studies usually separate person identification from action recognition. In this paper, we propose a skeleton-based end-to-end model that can simultaneously implement both person identification and action recognition and strengthen the learning of hard samples. Inspired by the recent application of recurrent neural networks and convolution neural networks to action analysis, the models of the temporal and spatial domains are created by modeling the global, local, and detailed feature-related information of the human skeleton. Moreover, during training, we add a mining and learning mechanism for hard samples that mines and focuses on them to avoid overfitting. This mining and learning mechanism enables the model to avoid a bottleneck and improve performance. The proposed model is experimentally verified on three datasets, where the results show that it can yield the state-of-the-art performance.
更多查看译文
关键词
Action recognition,person identification,convolutional neural networks,long short-term memory
AI 理解论文
溯源树
样例
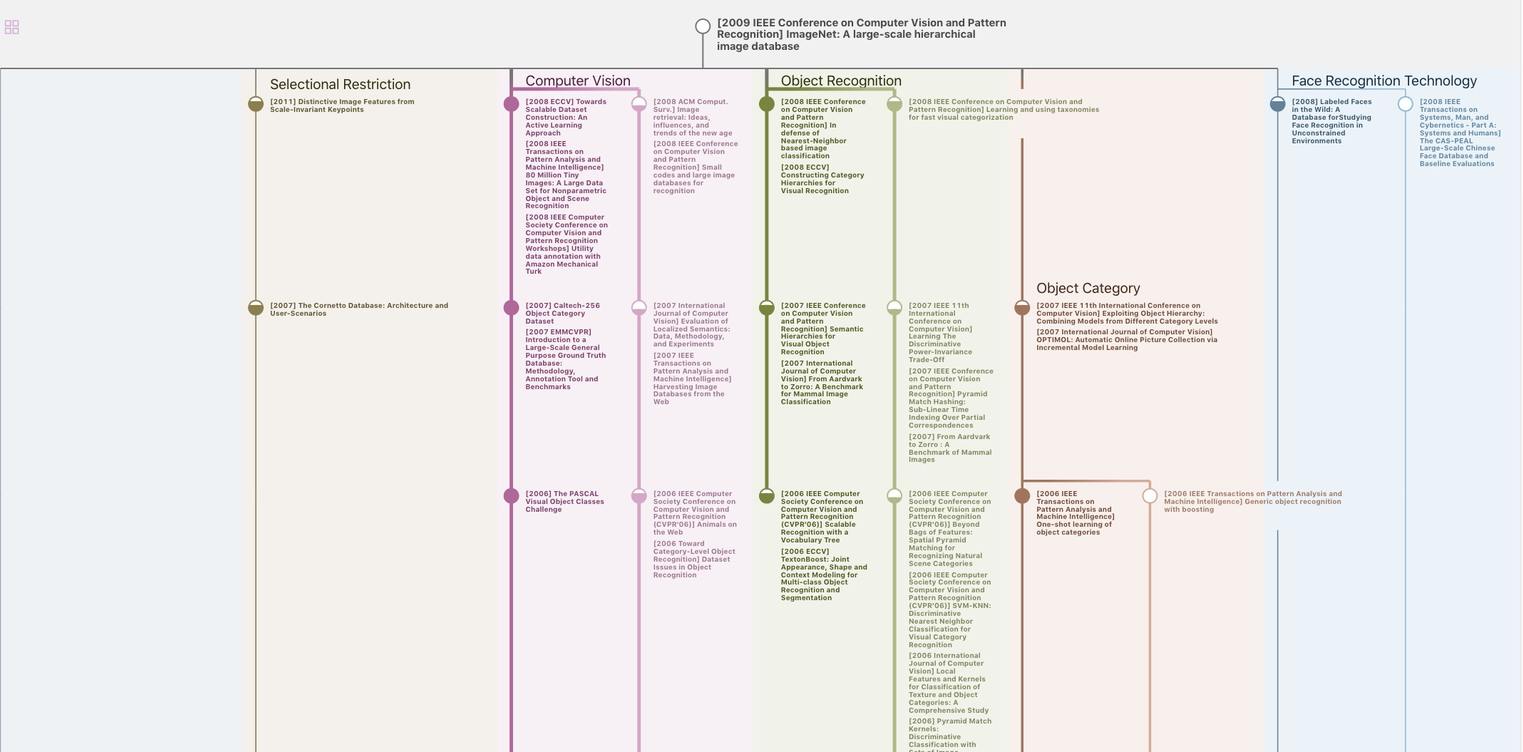
生成溯源树,研究论文发展脉络
Chat Paper
正在生成论文摘要