An Efficient Distributed Learning Algorithm Based On Effective Local Functional Approximations
JOURNAL OF MACHINE LEARNING RESEARCH(2018)
摘要
Scalable machine learning over big data is an important problem that is receiving a lot of attention in recent years. On popular distributed environments such as Hadoop running on a cluster of commodity machines, communication costs are substantial and algorithms need to be designed suitably considering those costs. In this paper we give a novel approach to the distributed training of linear classifiers (involving smooth losses and L-2 regularization) that is designed to reduce the total communication costs. At each iteration, the nodes minimize locally formed approximate objective functions; then the resulting minimizers are combined to form a descent direction to move. Our approach gives a lot of freedom in the formation of the approximate objective function as well as in the choice of methods to solve them. The method is shown to have O (log(1/epsilon)) time convergence. The method can be viewed as an iterative parameter mixing method. A special instantiation yields a parallel stochastic gradient descent method with strong convergence. When communication times between nodes are large, our method is much faster than the Terascale method (Agarwal et al., 2011), which is a state of the art distributed solver based on the statistical query model (Chu et al., 2006) that computes function and gradient values in a distributed fashion. We also evaluate against other recent distributed methods and demonstrate superior performance of our method.
更多查看译文
关键词
Distributed learning,Example partitioning,L-2 regularization
AI 理解论文
溯源树
样例
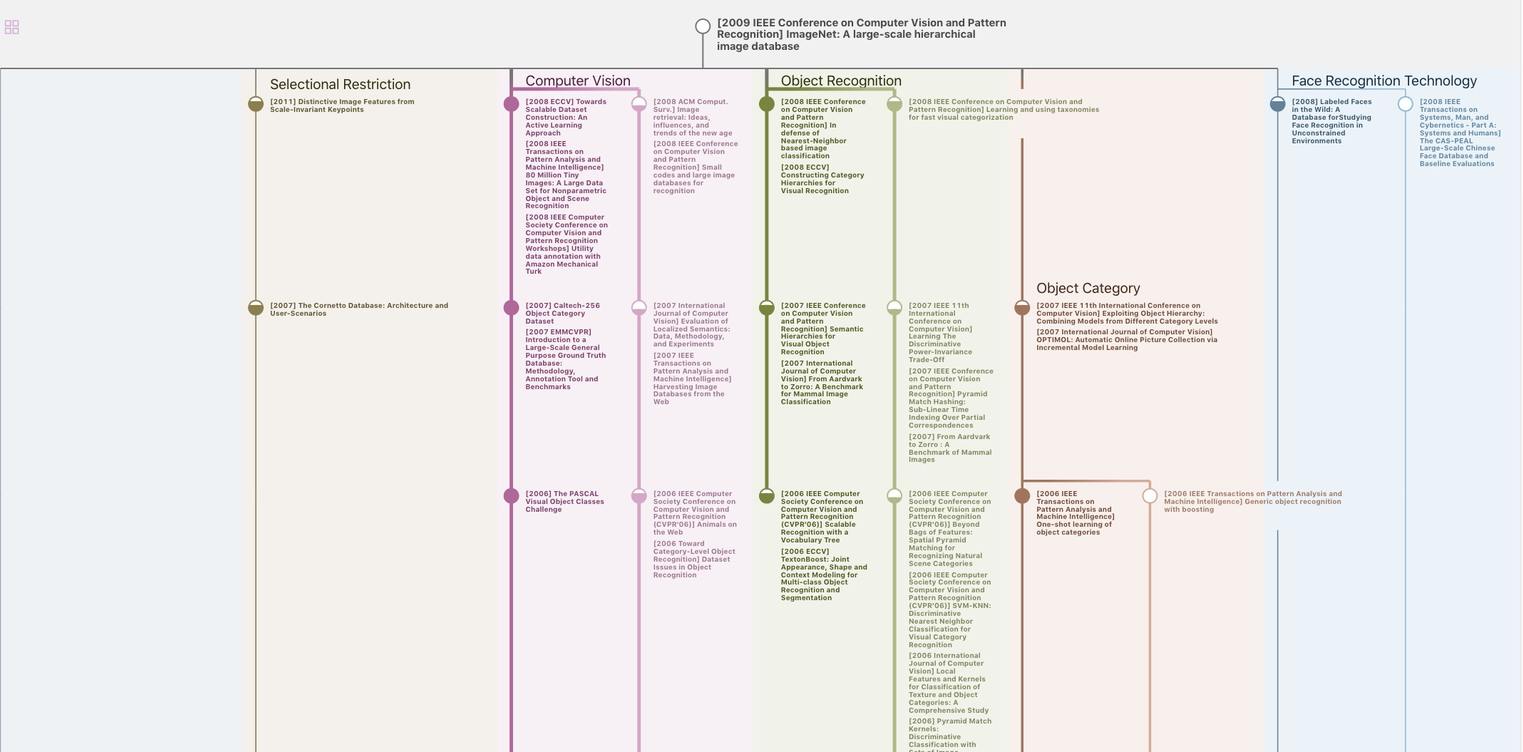
生成溯源树,研究论文发展脉络
Chat Paper
正在生成论文摘要