Automated Segmentation Based on Residual U-Net Model for MR Prostate Images.
CISP-BMEI(2018)
摘要
Computer-assisted prostate clinical diagnosis is significant for early detection and early treatment of prostate cancer. However, due to the small effective area of the prostate magnetic resonance (MR) images and similar gray values of other tissues around it, it is arduous to meet the clinical requirements by relying on the professional doctor to manually sketch the boundary, the challenges of prostate segmentation from MR images is arduous. In consideration of the superiority of convolution network in image processing field, we propose a U-Net model combined with residual connections to get more precise segmentation result on prostate MR images. First, we perform curvature-driven denoising on each prostate MR image and use histogram equalization to obtain potential boundary regions. Then, we enhance the MR image and perform network training. The results of experiments on the PROMISE12 dataset and the cooperative hospital datasets indicate that the proposed network model capable of more effective training, we got the 0.872 ± 0.053 in dice similarity coefficient (DSC), 1.45mm in average boundary distance (ABD) and 10.285mm in Harsdorf distance (HD), which illustrates the proposed method's effectiveness.
更多查看译文
关键词
Image segmentation,Training,Convolution,Biomedical imaging,Image edge detection,Feature extraction,Histograms
AI 理解论文
溯源树
样例
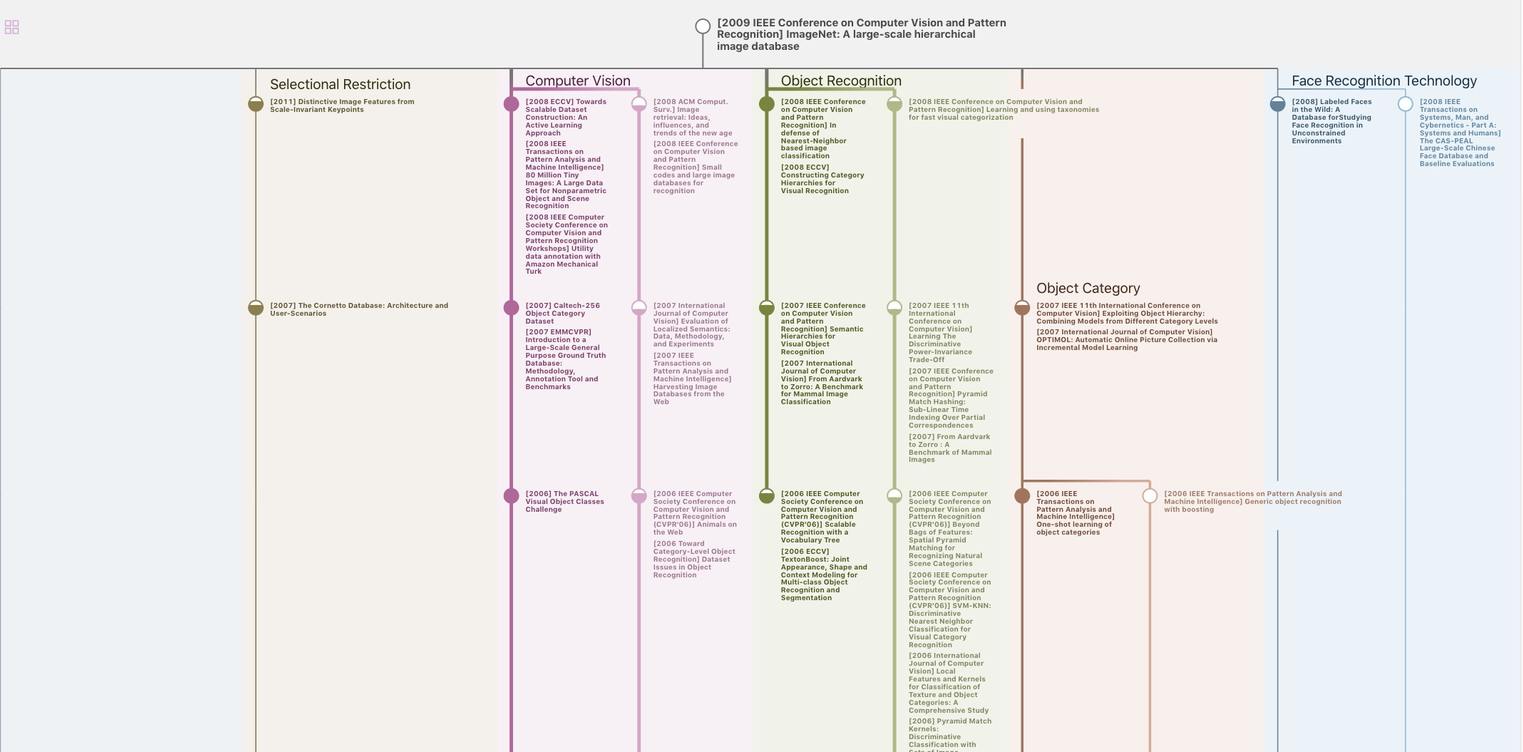
生成溯源树,研究论文发展脉络
Chat Paper
正在生成论文摘要