Physicochemical property based computational scheme for classifying DNA sequence elements of Saccharomyces cerevisiae.
Computational Biology and Chemistry(2019)
摘要
GenerationE of huge “omics” data necessitates the development and application of computational methods to annotate the data in terms of biological features. In the context of DNA sequence, it is important to unravel the hidden physicochemical signatures. For this purpose, we have considered various sequence elements such as promoter, ACS, LTRs, telomere, and retrotransposon of the model organism Saccharomyces cerevisiae. Contributions due to di-nucleotides play a major role in studying the DNA conformation profile. The physicochemical parameters used are hydrogen bonding energy, stacking energy and solvation energy per base pair. Our computational study shows that all sequence elements in this study have distinctive physicochemical signatures and the same can be exploited for prediction experiments. The order that we see in a DNA sequence is dictated by biological regions and hence, there exists role of dependency in the sequence makeup, keeping this in mind we are proposing two computational schemes (a) using a windowing block size procedure and (b) using di-nucleotide transitions. We obtained better discriminating profile when we analyzed the sequence data in windowing manner. In the second novel approach, we introduced the di-nucleotide transition probability matrix (DTPM) to study the hidden layer of information embedded in the sequences. DTPM has been used as weights for scanning and predictions. This proposed computational scheme incorporates the memory property which is more realistic to study the physicochemical properties embedded in DNA sequences. Our analysis shows that the DTPM scheme performs better than the existing method in this applied region. Characterization of these elements will be a key to genome editing applications and advanced machine learning approaches may also require such distinctive profiles as useful input features.
更多查看译文
关键词
DNA sequence elements,Physicochemical properties,DTPM,Computational scheme,Machine learning
AI 理解论文
溯源树
样例
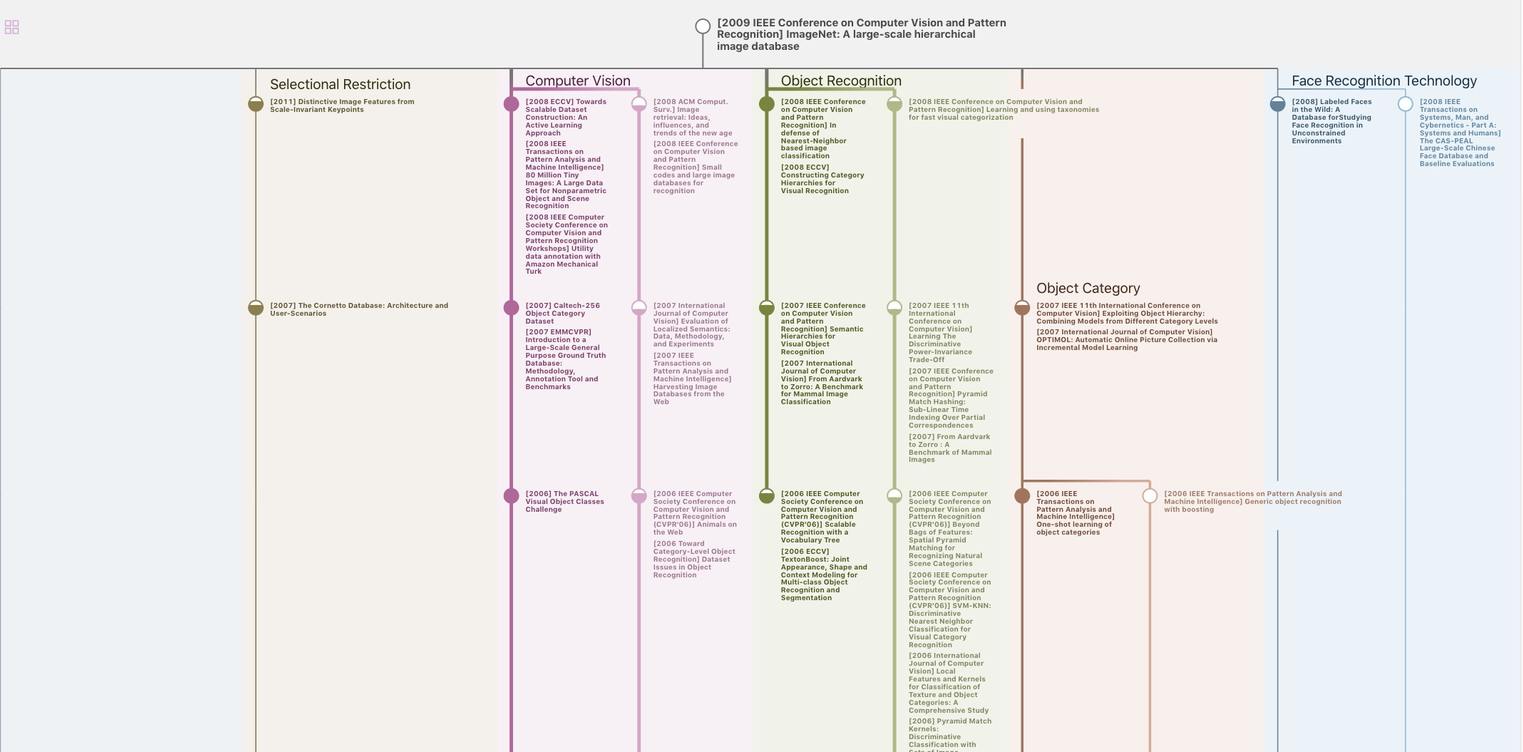
生成溯源树,研究论文发展脉络
Chat Paper
正在生成论文摘要