Efficient Approximate Algorithms for k-Regret Queries with Binary Constraints.
WISA(2018)
摘要
Extracting interesting points from a large dataset is an important problem for multi-criteria decision making. Recently, k-regret query was proposed and received attentions from the database community because it does not require any utility function from users and the output size is controllable. In this paper, we consider k-regret queries with binary constraints which doesn’t be addressed before. Given a collection of binary constraints, we study the problem of extracting the k representative points with small regret ratio while satisfying the binary constraints. To express the satisfaction of data points by the binary constraints, we propose two models named NBC and LBC in quantitative and qualitative ways respectively. In quantitative way, the satisfaction is expressed by a real number between 0 and 1 which quaNtifies the satisfaction of a point by the Binary Constraints. While in qualitative way, the satisfaction is modeled quaLitatively by a set of Binary Constraints which are satisfied by the point. Further, two efficient approximate algorithms called (NBC_P)-Greedy and (NBC_{DN})-Greedy are developed based on NBC while (LBC_{DN})-Greedy algorithm is proposed based on LBC. Extensive experiments on synthetic and real datasets confirm the efficiency and effectiveness of our proposed algorithms.
更多查看译文
关键词
k-Regret query,Binary constraints,Multi-criteria decision making,Top-k query,Skyline query
AI 理解论文
溯源树
样例
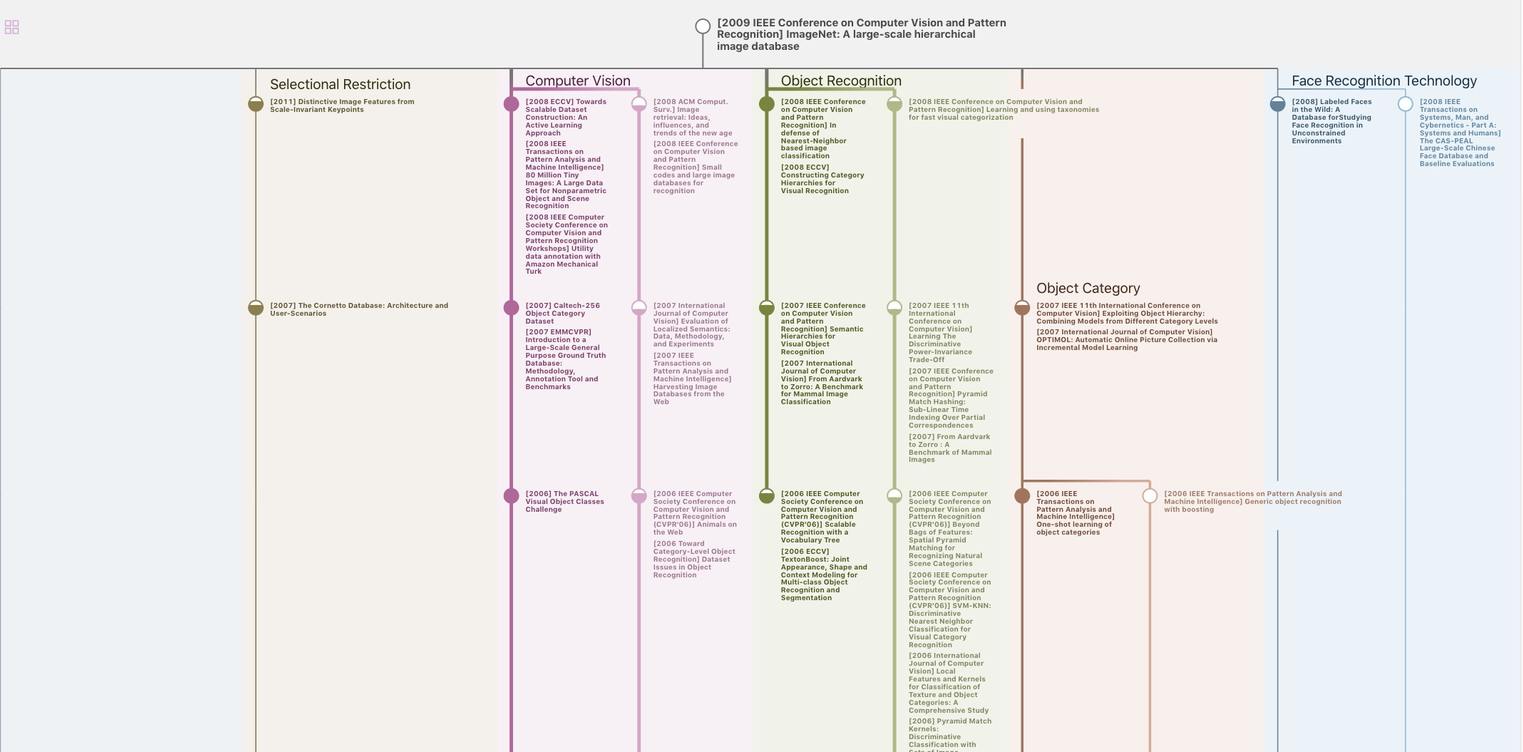
生成溯源树,研究论文发展脉络
Chat Paper
正在生成论文摘要