Clinical Value Of Machine Learning In The Automated Detection Of Focal Cortical Dysplasia Using Quantitative Multimodal Surface-Based Features
FRONTIERS IN NEUROSCIENCE(2019)
Abstract
Objective: To automatically detect focal cortical dysplasia (FCD) lesion by combining quantitative multimodal surface-based features with machine learning and to assess its clinical value.Methods: Neuroimaging data and clinical information for 74 participants (40 with histologically proven FCD type II) was retrospectively included. The morphology, intensity and function-based features characterizing FCD lesions were calculated vertex-wise on each cortical surface and fed to an artificial neural network. The classifier performance was quantitatively and qualitatively assessed by performing statistical analysis and conventional visual analysis.Results: The accuracy, sensitivity, specificity of the neural network classifier based on multimodal surface-based features were 70.5%, 70.0%, and 69.9%, respectively, which outperformed the unimodal classifier. There was no significant difference in the detection rate of FCD subtypes (Pearson's Chi-Square = 0.001, p = 0.970). Cohen's kappa score between automated detection outcomes and post-surgical resection region was 0.385 (considered as fair).Conclusion: Automated machine learning with multimodal surface features can provide objective and intelligent detection of FCD lesion in pre-surgical evaluation and can assist the surgical strategy. Furthermore, the optimal parameters, appropriate surface features and efficient algorithm are worth exploring.
MoreTranslated text
Key words
focal cortical dysplasia,machine learning,metabolic,morphological,quantitative
AI Read Science
Must-Reading Tree
Example
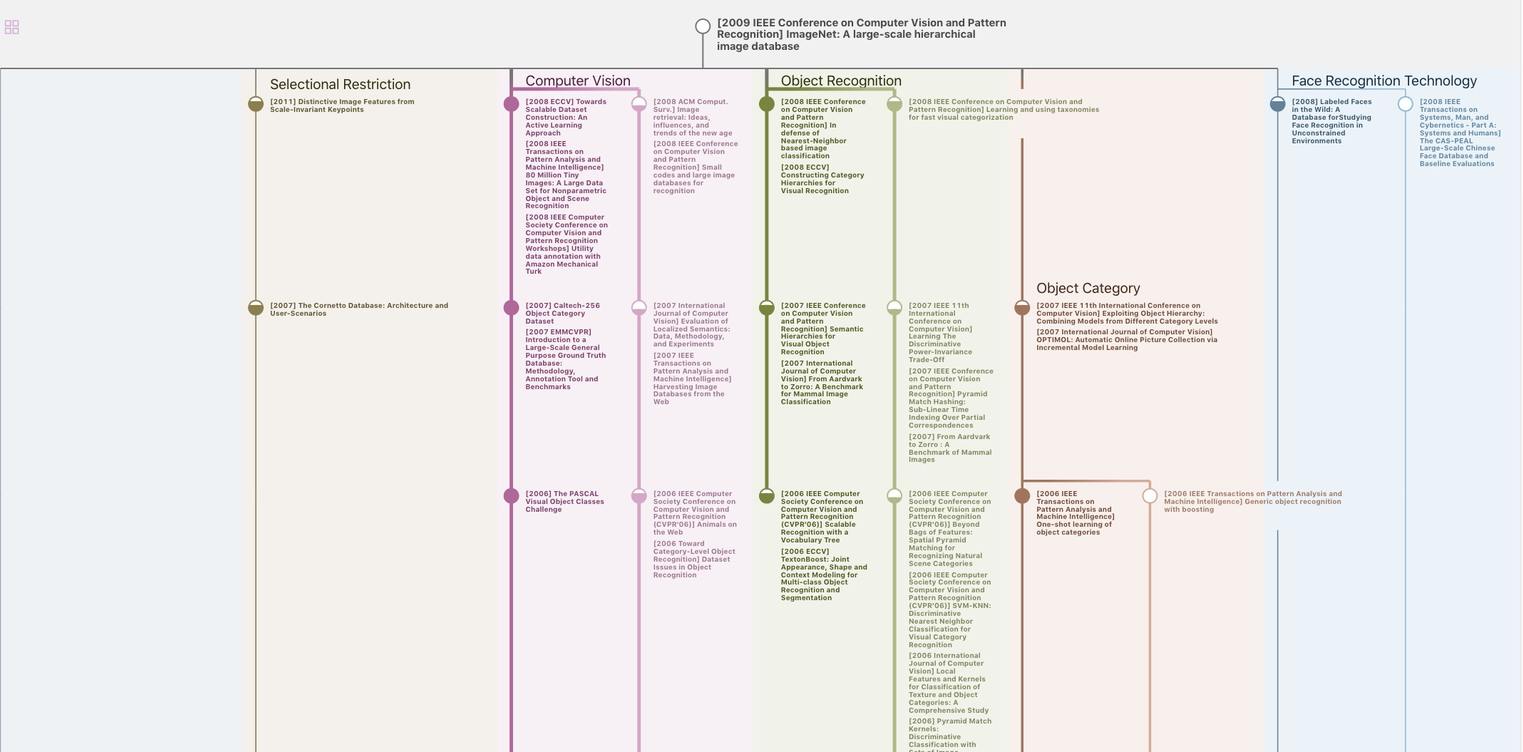
Generate MRT to find the research sequence of this paper
Chat Paper
Summary is being generated by the instructions you defined