Vision Memory For Target Object Navigation Using Deep Reinforcement Learning: An Empirical Study
2018 IEEE INTERNATIONAL CONFERENCE ON SYSTEMS, MAN, AND CYBERNETICS (SMC)(2018)
摘要
Recently, a number of methods have been conducted by combining deep neural network and reinforcement learning to solve problems. Neural networks have strong ability to deal with high dimensional data and a good mean to learn features while reinforcement learning allows a system to learn by experience optimal action control and adjust its behavior to new environments. It thus may be used in mobile robot navigation tasks with perceptron as deep neural network and learning to control by reinforcement learning.We first investigate some issues in agents learning to navigate in indoor environments such as how robot memorize vision information and how it discovers the environments. We show that agents can rely on some position with vision information in navigation task such as reduce training time and locating object efficiently. Some approaches based on deep reinforcement learning are step by step discussed and proposed to deal with each problem in target object navigation task. The key ideas include adding checking point as vision memory and using auxiliary learning task to enhance agents to discover the environment.
更多查看译文
关键词
Deep Reinforcement Learning, Mobile Robots, Indoor Navigation, Target Object Driven
AI 理解论文
溯源树
样例
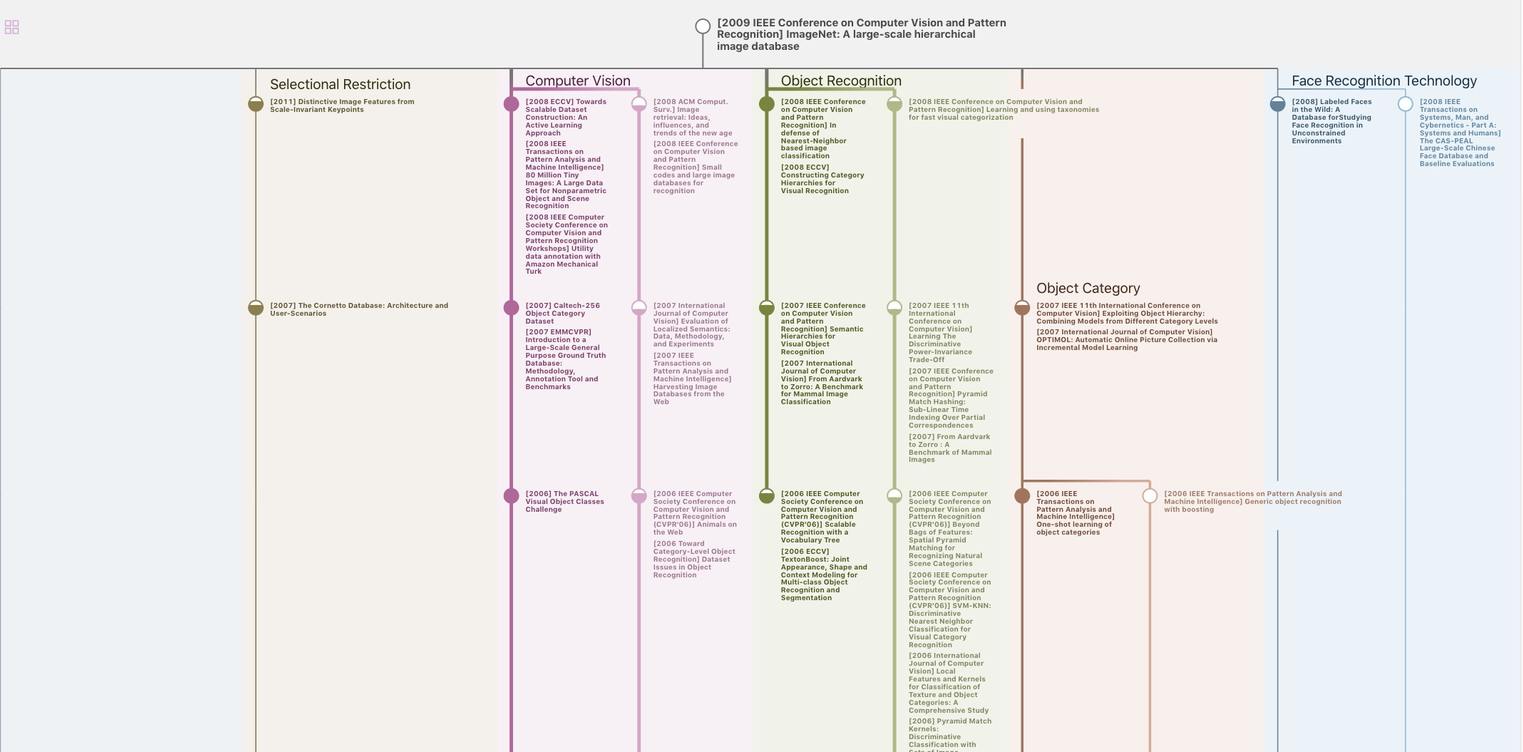
生成溯源树,研究论文发展脉络
Chat Paper
正在生成论文摘要