Feature Boosting Network For 3D Pose Estimation.
IEEE Transactions on Pattern Analysis and Machine Intelligence(2020)
摘要
In this paper, a feature boosting network is proposed for estimating 3D hand pose and 3D body pose from a single RGB image. In this method, the features learned by the convolutional layers are boosted with a new long short-term dependence-aware (LSTD) module, which enables the intermediate convolutional feature maps to perceive the graphical long short-term dependency among different hand (or body) parts using the designed Graphical ConvLSTM. Learning a set of features that are reliable and discriminatively representative of the pose of a hand (or body) part is difficult due to the ambiguities, texture and illumination variation, and self-occlusion in the real application of 3D pose estimation. To improve the reliability of the features for representing each body part and enhance the LSTD module, we further introduce a context consistency gate (CCG) in this paper, with which the convolutional feature maps are modulated according to their consistency with the context representations. We evaluate the proposed method on challenging benchmark datasets for 3D hand pose estimation and 3D full body pose estimation. Experimental results show the effectiveness of our method that achieves state-of-the-art performance on both of the tasks.
更多查看译文
关键词
Three-dimensional displays,Pose estimation,Two dimensional displays,Boosting,Logic gates,Reliability,Task analysis
AI 理解论文
溯源树
样例
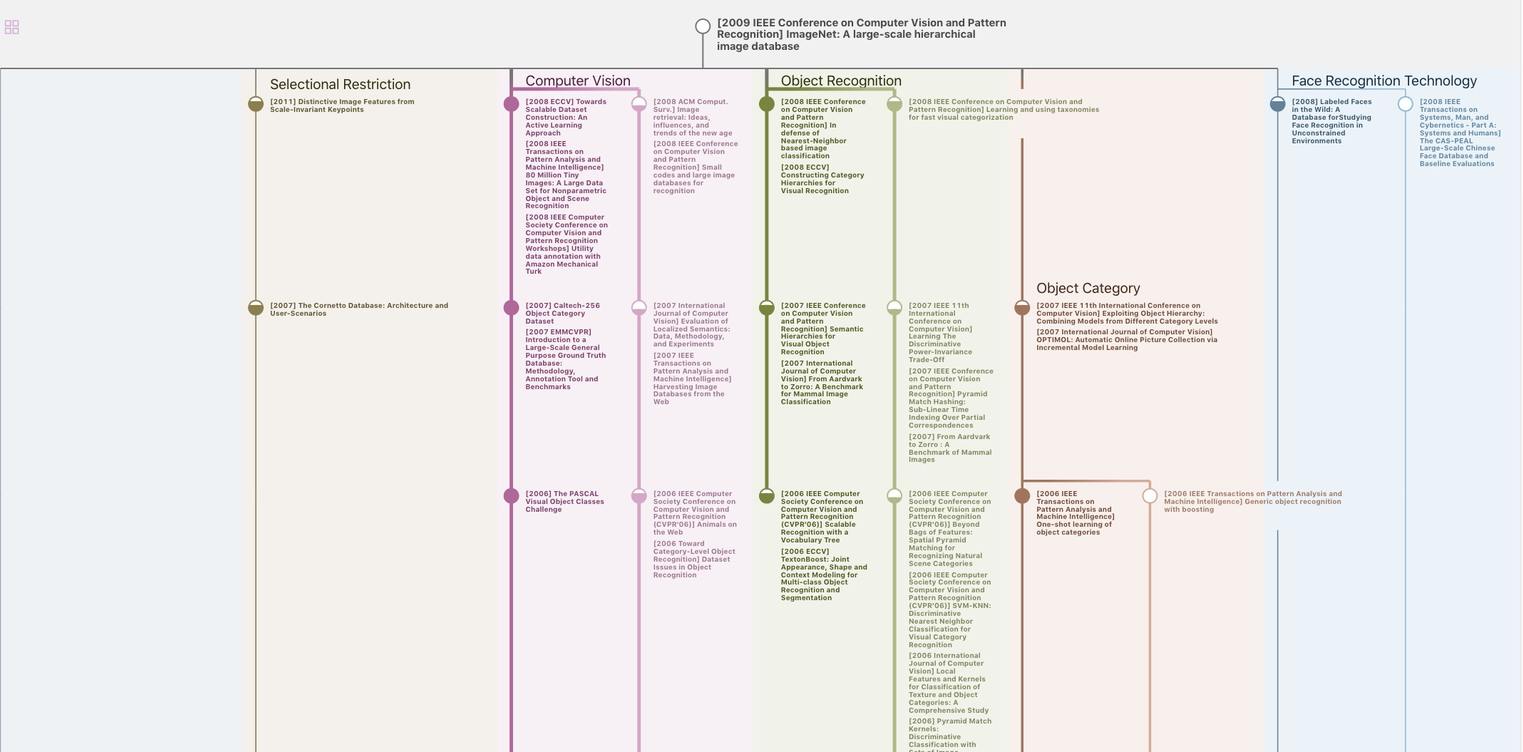
生成溯源树,研究论文发展脉络
Chat Paper
正在生成论文摘要