Attaining human-level performance with atlas location autocontext for anatomical landmark detection in 3D CT data
COMPUTER VISION - ECCV 2018 WORKSHOPS, PT III(2018)
Abstract
We present an efficient neural network method for locating anatomical landmarks in 3D medical CT scans, using atlas location autocontext in order to learn long-range spatial context. Location predictions are made by regression to Gaussian heatmaps, one heatmap per landmark. This system allows patchwise application of a shallow network, thus enabling multiple volumetric heatmaps to be predicted concurrently without prohibitive GPU memory requirements. Further, the system allows inter-landmark spatial relationships to be exploited using a simple overdetermined affine mapping that is robust to detection failures and occlusion or partial views. Evaluation is performed for 22 landmarks defined on a range of structures in head CT scans. Models are trained and validated on 201 scans. Over the final test set of 20 scans which was independently annotated by 2 human annotators, the neural network reaches an accuracy which matches the annotator variability, with similar human and machine patterns of variability across landmark classes.
MoreTranslated text
AI Read Science
Must-Reading Tree
Example
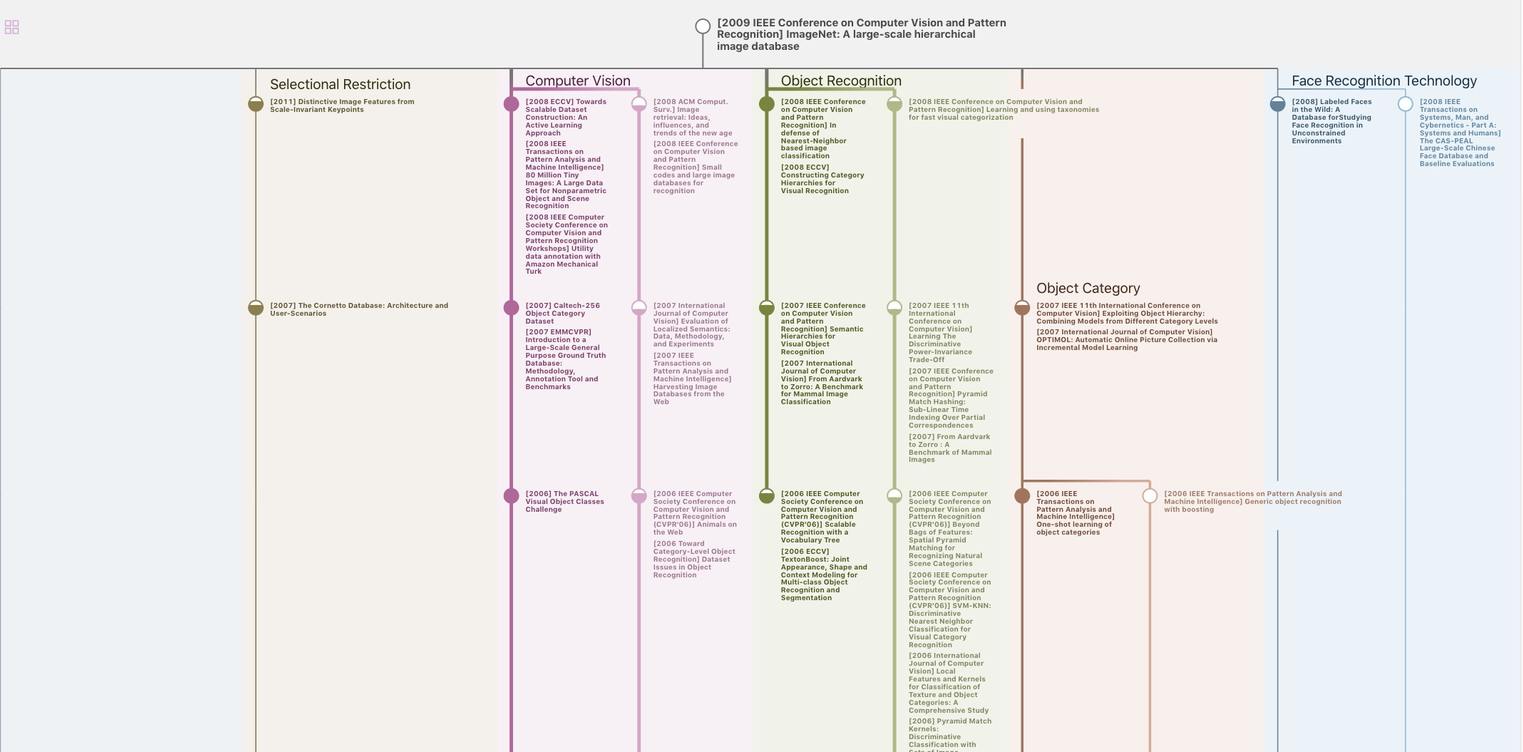
Generate MRT to find the research sequence of this paper
Chat Paper
Summary is being generated by the instructions you defined