A Memory Model Based On The Siamese Network For Long-Term Tracking
COMPUTER VISION - ECCV 2018 WORKSHOPS, PT I(2019)
摘要
We propose a novel memory model using deep convolutional features for long-term tracking to handle the challenging issues, including visual deformation or target disappearance. Our memory model is separated into short- and long-term stores inspired by Atkinson-Shiffrin Memory Model (ASMM). In the tracking step, the bounding box of the target is estimated by the Siamese features obtained from both memory stores to accommodate changes in the visual appearance of the target. In the re-detection step, we take features only in the long-term store to alleviate the drift problem. At this time, we adopt a coarse-to-fine strategy to detect the target in the entire image without the dependency of the previous position. In the end, we employ Regional Maximum Activation of Convolutions (R-MAC) as key criteria. Our tracker achieves an F-score of 0.52 on the LTB35 dataset, which is 0.04 higher than the performance of the state-of-the-art algorithm.
更多查看译文
关键词
Long-term tracking, Atkinson-Shiffrin Memory Model, Siamese network, Regional Maximum Activation of Convolutions
AI 理解论文
溯源树
样例
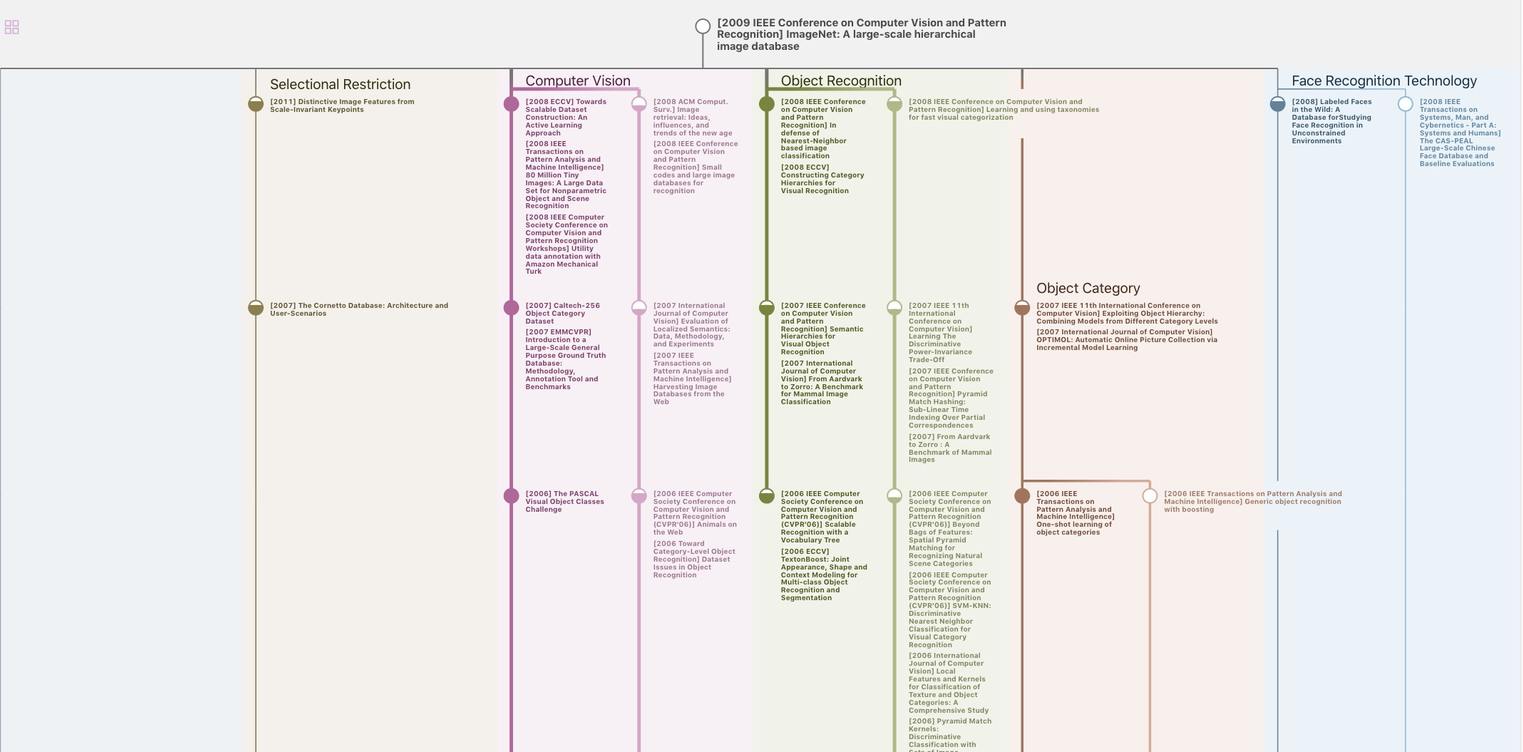
生成溯源树,研究论文发展脉络
Chat Paper
正在生成论文摘要