Distant Vehicle Detection: How Well Can Region Proposal Networks Cope With Tiny Objects At Low Resolution?
COMPUTER VISION - ECCV 2018 WORKSHOPS, PT I(2019)
摘要
High-performance faster R-CNN has been applied to many detection tasks. Detecting tiny objects at very low resolution remains a challenge, however, and a few studies addressed explicitly the detection of such objects yet. Focusing on distant object detection at very low resolution images for driver assistance systems, we introduce post-trained net surgery to (1) analyze the network activation patterns, (2) study the potential of prior information to improve localization and binary classification performance, and (3) to support the development of priors for improving the network performance.We use post-trained net surgery to analyze the feature maps used for bounding box regression and classification for RPNs in detail, and to discuss the complexity of the network activation patterns. Using these findings, we show that incorporating prior maps into the network architecture improves the performance of bounding box regression and binary classification for small object detection in low resolution images.
更多查看译文
关键词
Saliency maps, Region proposal network, Low resolution, Object detection
AI 理解论文
溯源树
样例
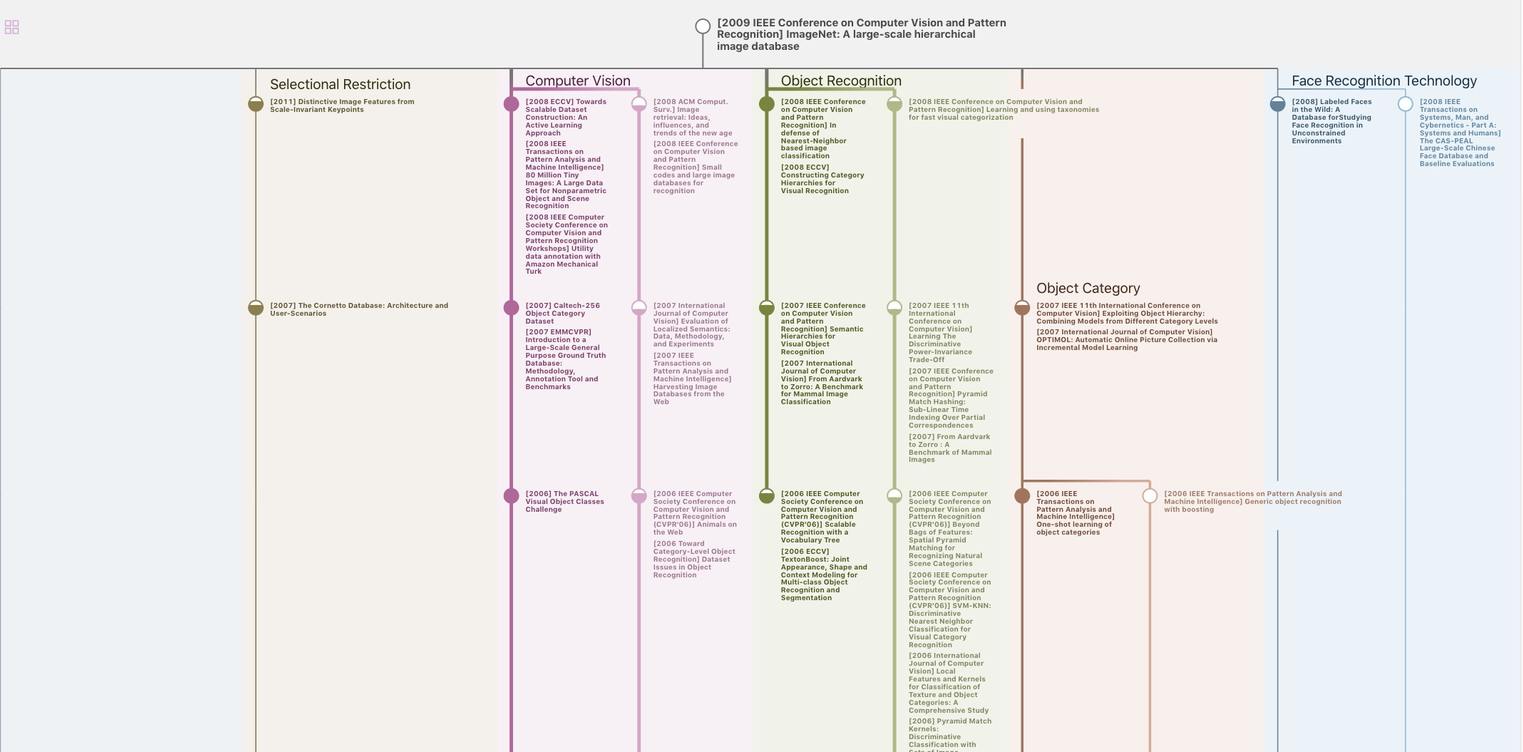
生成溯源树,研究论文发展脉络
Chat Paper
正在生成论文摘要