Quasi-Newton methods: superlinear convergence without line searches for self-concordant functions.
OPTIMIZATION METHODS & SOFTWARE(2019)
摘要
We consider the use of a curvature-adaptive step size in gradient-based iterative methods, including quasi-Newton methods, for minimizing self-concordant functions, extending an approach first proposed for Newton's method by Nesterov. This step size has a simple expression that can be computed analytically; hence, line searches are not needed. We show that using this step size in the BFGS method (and quasi-Newton methods in the Broyden convex class other than the DFP method) results in superlinear convergence for strongly convex self-concordant functions. We present numerical experiments comparing gradient descent and BFGS methods using the curvature-adaptive step size to traditional methods on deterministic logistic regression problems, and to versions of stochastic gradient descent on stochastic optimization problems.
更多查看译文
关键词
Quasi-Newton methods,BFGS,self-concordant functions,damped Newton method
AI 理解论文
溯源树
样例
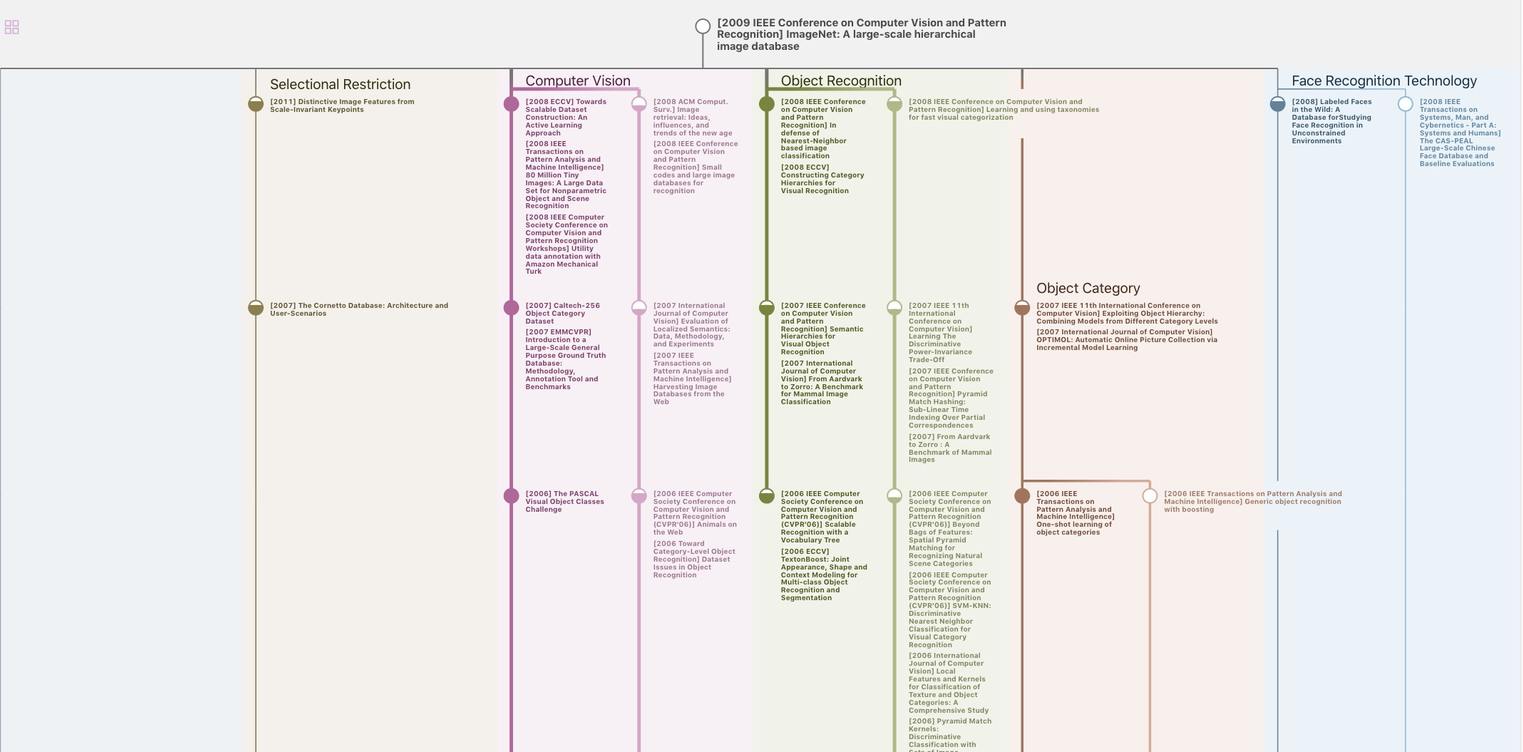
生成溯源树,研究论文发展脉络
Chat Paper
正在生成论文摘要