Adepos: Anomaly Detection Based Power Saving For Predictive Maintenance Using Edge Computing
24TH ASIA AND SOUTH PACIFIC DESIGN AUTOMATION CONFERENCE (ASP-DAC 2019)(2019)
Abstract
In Industry 4.0, predictive maintenance (PdM) is one of the most important applications pertaining to the Internet of Things (IoT). Machine learning is used to predict the possible failure of a machine before the actual event occurs. However, main challenges in PdM are: (a) lack of enough data from failing machines, and (b) paucity of power and bandwidth to transmit sensor data to cloud throughout the lifetime of the machine. Alternatively, edge computing approaches reduce data transmission and consume low energy. In this paper, we propose Anomaly Detection based Power Saving (ADEPOS) scheme using approximate computing through the lifetime of the machine. In the beginning of the machine's life, low accuracy computations are used when machine is healthy. However, on detection of anomalies as time progresses, system is switched to higher accuracy modes. We show using the NASA bearing dataset that using ADEPOS, we need 8.8X less neurons on average and based on post-layout results, the resultant energy savings are 6.4-6.65X.
MoreTranslated text
Key words
Predictive maintenance, approximate computing, IoT, anomaly detection, edge computing
AI Read Science
Must-Reading Tree
Example
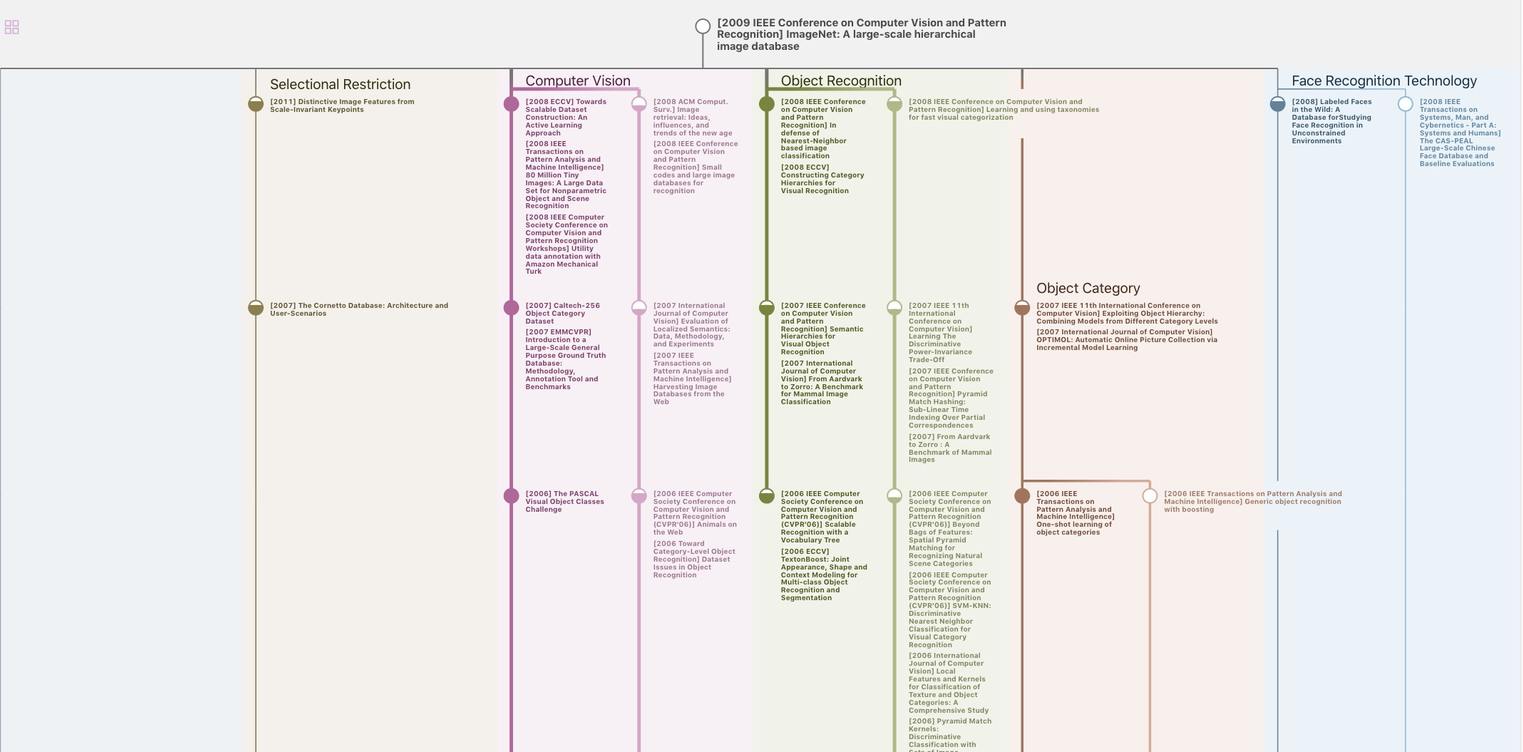
Generate MRT to find the research sequence of this paper
Chat Paper
Summary is being generated by the instructions you defined