Scalable Algorithms For Learning High-Dimensional Linear Mixed Models
UNCERTAINTY IN ARTIFICIAL INTELLIGENCE(2018)
摘要
Linear mixed models (LMMs) are used extensively to model observations that are not independent. Parameter estimation for LMMs can be computationally prohibitive on big data. State-of-the-art learning algorithms require computational complexity which depends at least linearly on the dimension p of the covariates, and often use heuristics that do not offer theoretical guarantees. We present scalable algorithms for learning high-dimensional LMMs with sublinear computational complexity dependence on p. Key to our approach are novel dual estimators which use only kernel functions of the data, and fast computational techniques based on the subsampled randomized Hadamard transform. We provide theoretical guarantees for our learning algorithms, demonstrating the robustness of parameter estimation. Finally, we complement the theory with experiments on large synthetic and real data.
更多查看译文
关键词
scalable algorithms,models,learning,high-dimensional
AI 理解论文
溯源树
样例
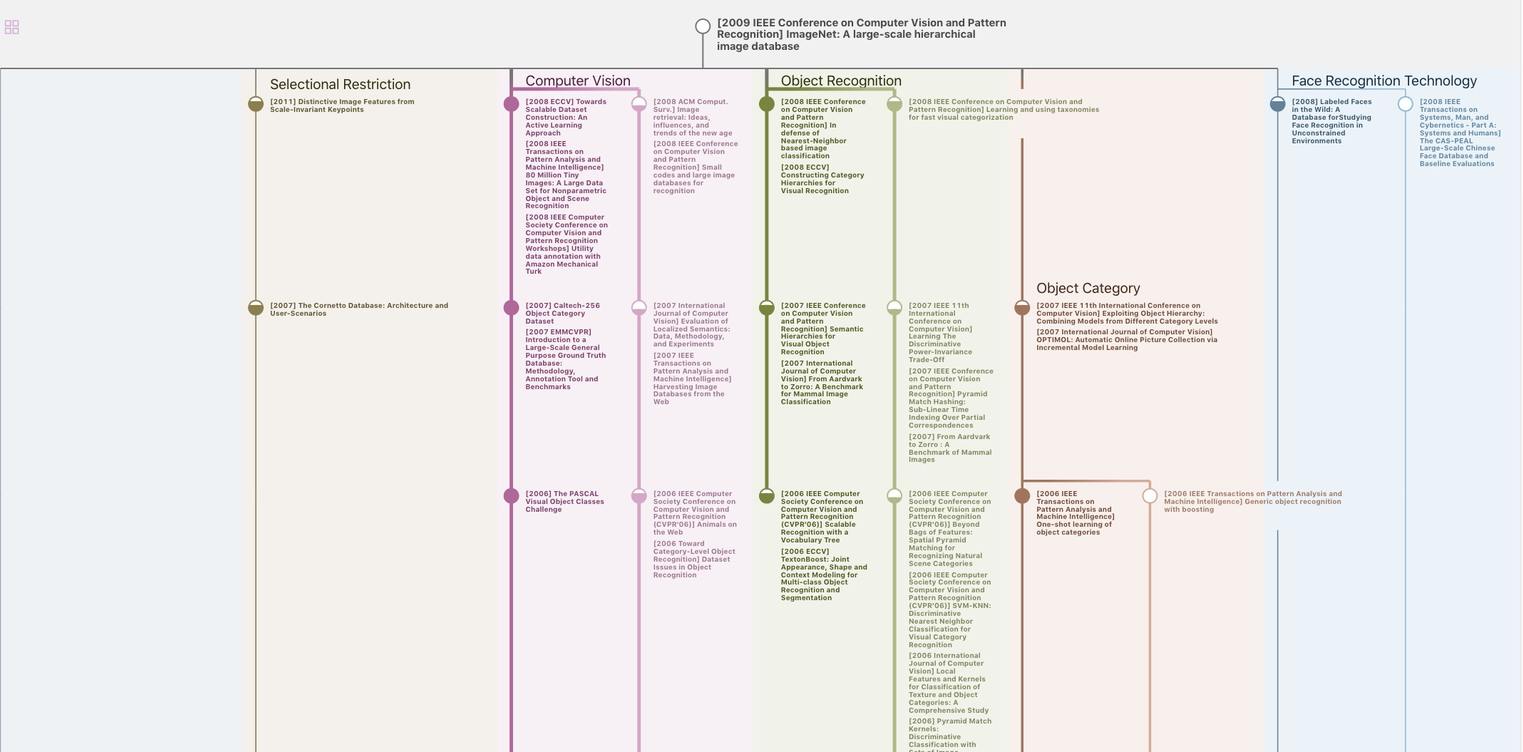
生成溯源树,研究论文发展脉络
Chat Paper
正在生成论文摘要