Interpretable multiple data streams clustering with clipped streams representation for the improvement of electricity consumption forecasting
Data Mining and Knowledge Discovery(2018)
Abstract
This paper presents a new interpretable approach for multiple data streams clustering in a smart grid used for the improvement of forecasting accuracy of aggregated electricity consumption and grid analysis named ClipStream . Consumers time series streams are compressed and represented by interpretable features extracted from the clipped representation. The proposed representation has low computational complexity and is incremental in the sense of the windowing method. From the extracted features, outlier consumers can be simply and quickly detected. The clustering phase consists of three parts: clustering non-outlier representations, the aggregation of consumption within clusters, and unsupervised change detection procedure on aggregated time series streams windows. ClipStream behaviour and its forecasting accuracy improvement were evaluated on four different real datasets containing variable patterns of electricity consumption. The clustering accuracy with the proposed feature extraction method from the clipped representation was evaluated on 85 time series datasets from a large public repository. The results of experiments proved the stability of the proposed ClipStream in the sense of improving forecasting accuracy and showed the suitability of the proposed representation in many tested applications.
MoreTranslated text
Key words
Data streams clustering,Time series representations,Electricity consumption forecasting
AI Read Science
Must-Reading Tree
Example
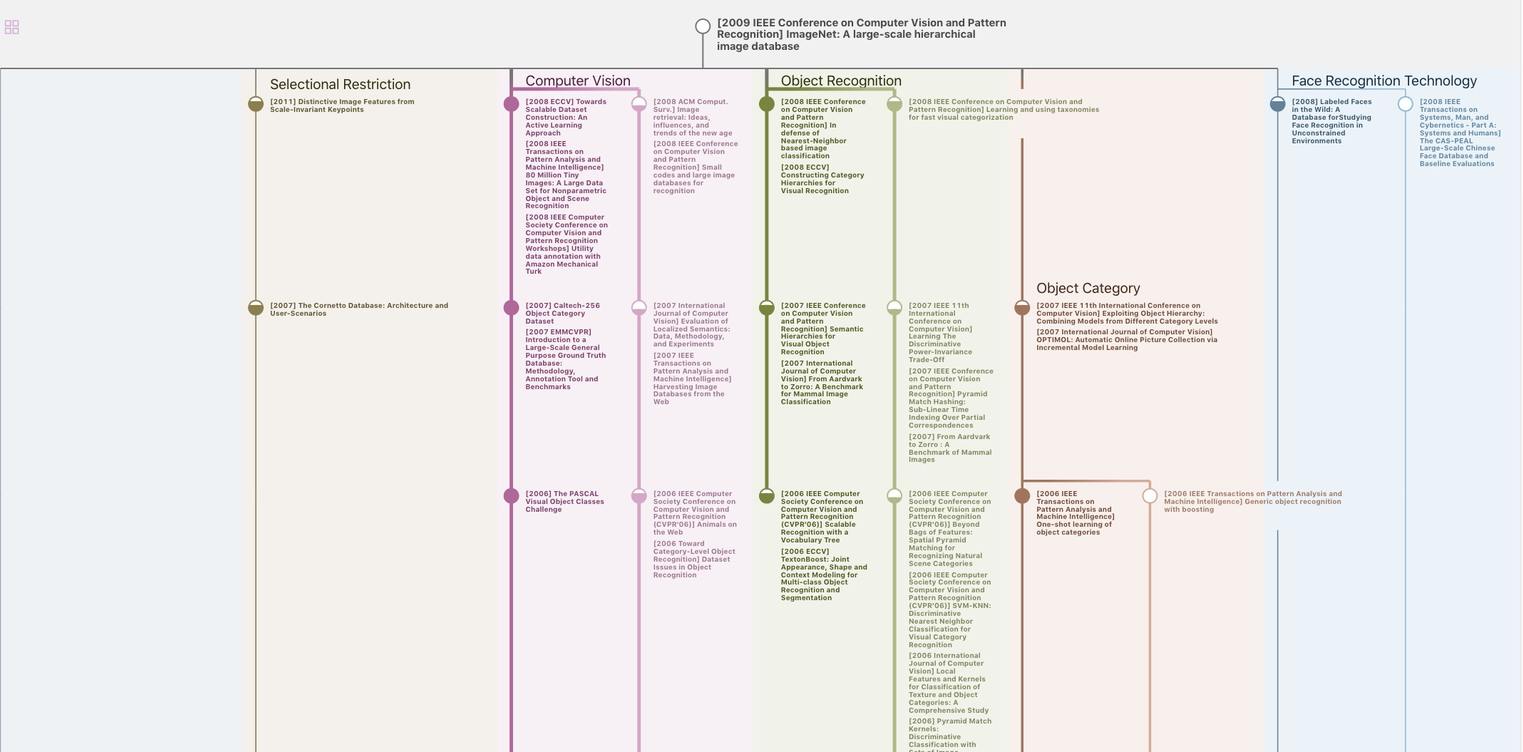
Generate MRT to find the research sequence of this paper
Chat Paper
Summary is being generated by the instructions you defined