Prediction of water quality effect on saturated hydraulic conductivity of soil by artificial neural networks
Paddy and Water Environment(2018)
摘要
This study was conducted to investigate the impact of water salinity (EC w ) and sodicity (SAR w ) on saturated ( K s ) and relative ( K r ) hydraulic conductivities in two clay (C) and sandy clay loam (SCL) soils. The results showed that the K s decreased with increasing SAR w , and in all of water quality treatments, the K s of SCL soil was higher than that of the C soil. Sodicity effect (even at high SAR w ) on the K r of clay soil was minimized by high salinity. Although K r of both soils similarly responded to EC w and SAR w , microstructure of clay soil was more sensitive to water quality. Effect of EC w on soil structure was greater than that of SAR w . In order to assess the applicability of artificial neural networks (ANNs) in estimating K s and K r , two types of FFBP and CFBP ANNs and two training algorithms, namely Levenberg–Marquardt (LM) and Bayesian regulation, were employed with two strategies of uniform threshold and different threshold functions. Multiple linear regressions were also used for K s and K r prediction. Based on the ANN results of second strategy, best topology (4–5–4–1) was belonged to CFBP network with LM algorithm, LOGSIG–LOGSIG–TANSIG threshold functions, and values of MAE and R 2 are equal to 0.1761 and 0.9945, respectively. Overall, the efficacy of ANNs is much greater than regression method for K s prediction.
更多查看译文
关键词
Artificial neural networks,Salinity,Soil hydraulic conductivity,Water quality
AI 理解论文
溯源树
样例
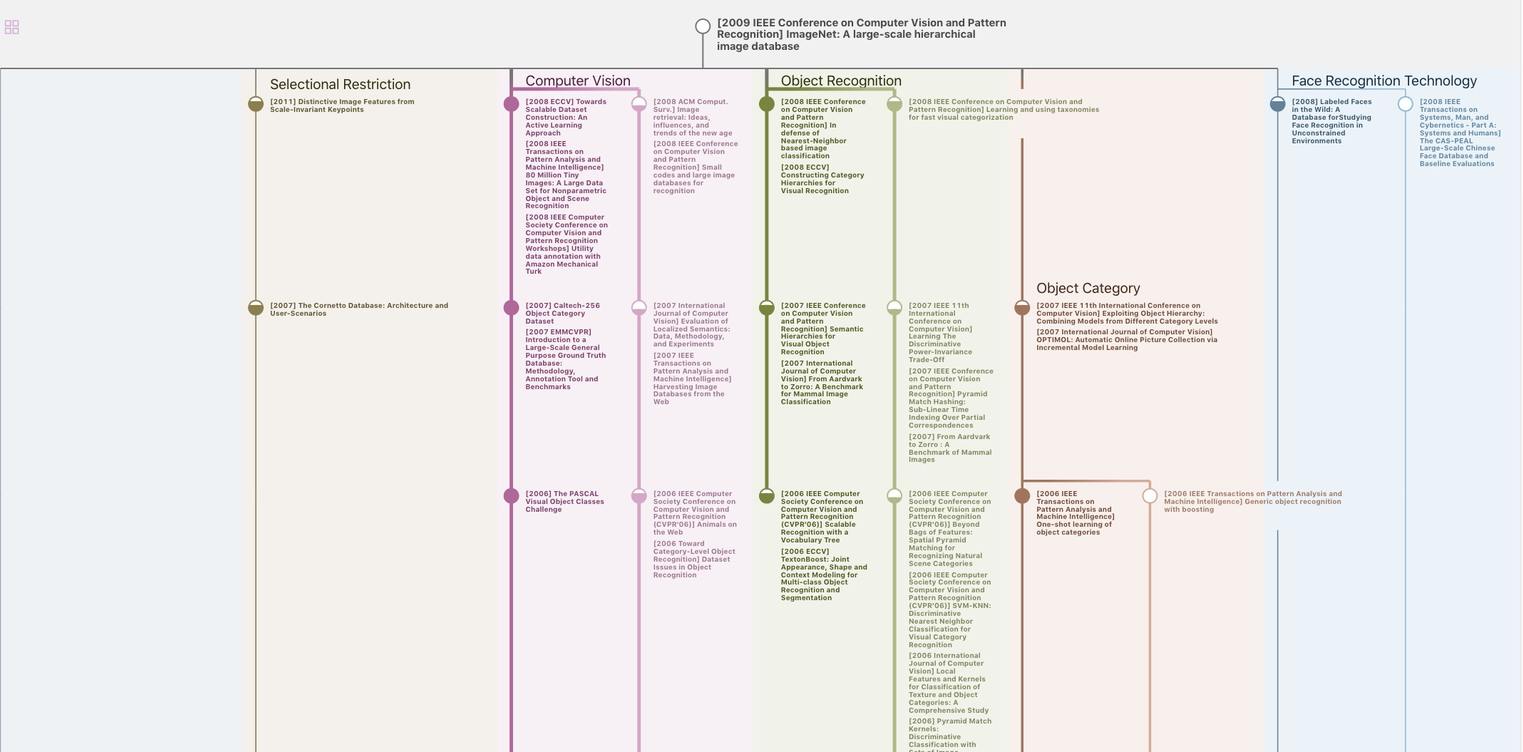
生成溯源树,研究论文发展脉络
Chat Paper
正在生成论文摘要