Research on CNN Parallel Computing and Learning Architecture Based on Real-Time Streaming Architecture.
ICDCIT(2019)
摘要
Convolutional neural network (CNN) is a deep feed-forward artificial neural network, which is widely used in image recognition. However, this mode highlights the problems that the training time is too long and memory is insufficient. Traditional acceleration methods are mainly limited to optimizing for an algorithm. In this paper, we propose a method, namely CNN-S, to improve training efficiency and cost based on Storm and is suitable for every algorithm. This model divides data into several sub sets and processes data on several machine in parallel flexibly. The experimental results show that in the case of achieving a recognition accuracy rate of 95%, the training time of single serial model is around 913 s, and in CNN-S model only needs 248 s. The acceleration ratio can reach 3.681. This shows that the CNN-S parallel model has better performance than single serial mode on training efficiency and cost of system resource.
更多查看译文
关键词
CNN, Parallel computing, Apache storm, Real time
AI 理解论文
溯源树
样例
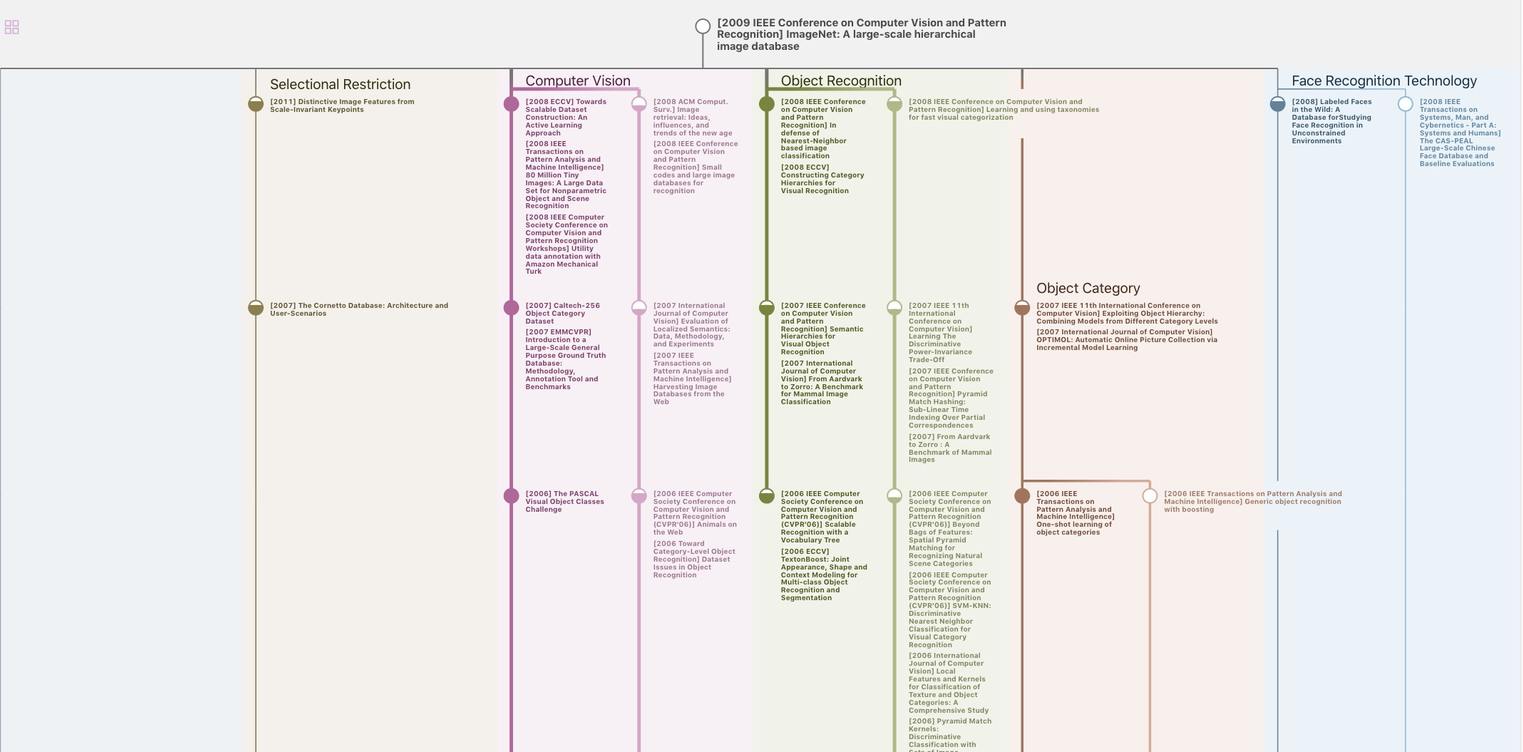
生成溯源树,研究论文发展脉络
Chat Paper
正在生成论文摘要