Efficient Long-term Mapping in Dynamic Environments
2018 IEEE/RSJ International Conference on Intelligent Robots and Systems (IROS)(2018)
摘要
As autonomous robots are increasingly being introduced in real-world environments operating for long periods of time, the difficulties of long-term mapping are attracting the attention of the robotics research community. This paper proposes a full SLAM system capable of handling the dynamics of the environment across a single or multiple mapping sessions. Using the pose graph SLAM paradigm, the system works on local maps in the form of 2D point cloud data which are updated over time to store the most up-to-date state of the environment. The core of our system is an efficient ICP-based alignment and merging procedure working on the clouds that copes with non-static entities of the environment. Furthermore, the system retains the graph complexity by removing out-dated nodes upon robust inter- and intra-session loop closure detections while graph coherency is preserved by using condensed measurements. Experiments conducted with real data from longterm SLAM datasets demonstrate the efficiency, accuracy and effectiveness of our system in the management of the mapping problem during long-term robot operation.
更多查看译文
关键词
mapping problem,longterm SLAM datasets,graph coherency,intra-session loop closure detections,out-dated nodes,graph complexity,nonstatic entities,merging procedure,efficient ICP-based alignment,up-to-date state,2D point cloud data,local maps,graph SLAM paradigm,multiple mapping sessions,single mapping sessions,SLAM system,autonomous robots,dynamic environments,long-term robot operation
AI 理解论文
溯源树
样例
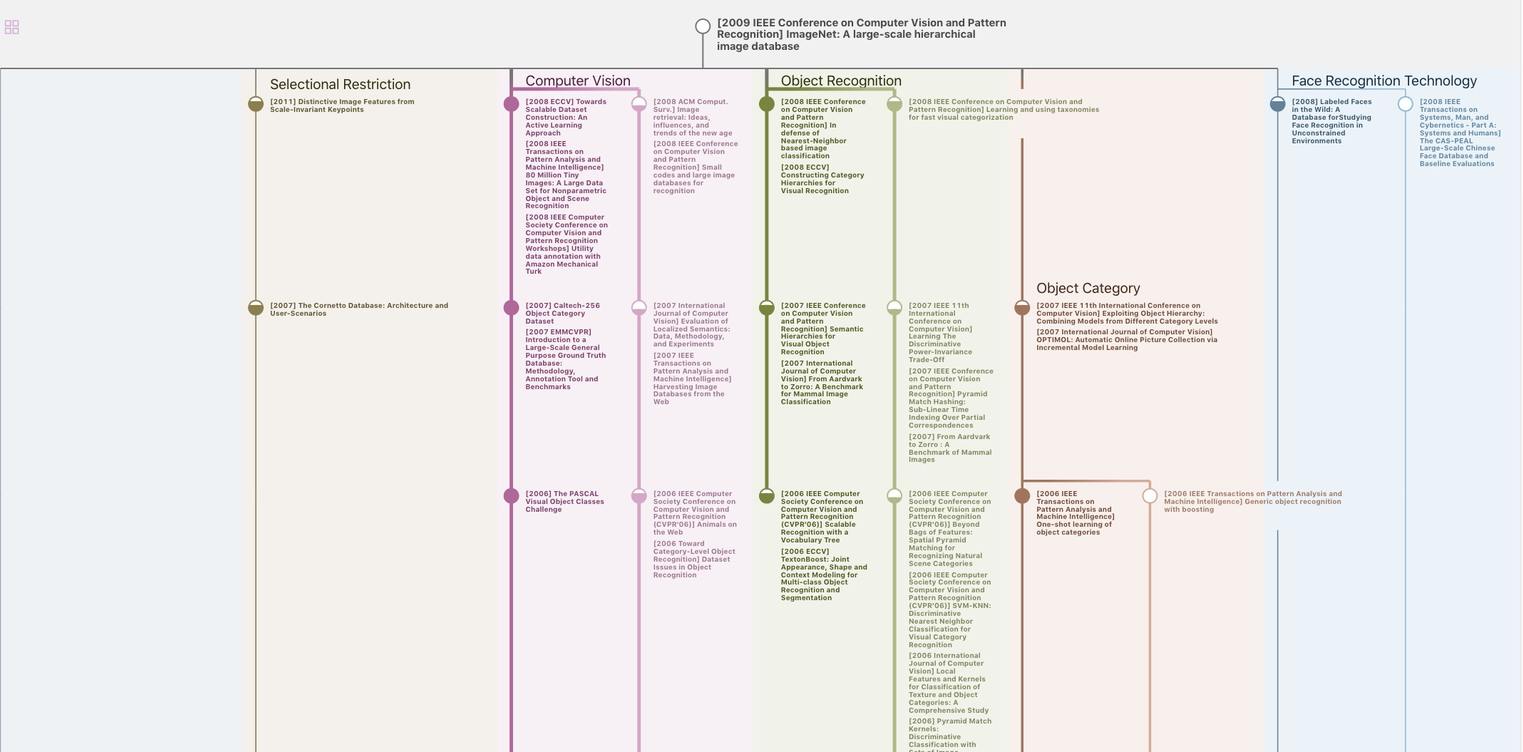
生成溯源树,研究论文发展脉络
Chat Paper
正在生成论文摘要