Compensating For Context By Learning Local Models Of Perception Performance
2018 IEEE/RSJ INTERNATIONAL CONFERENCE ON INTELLIGENT ROBOTS AND SYSTEMS (IROS)(2018)
摘要
Perception system performance can vary dramatically with contextual factors such as environmental geometry, appearance, and other phenomena. In this work we present a theoretical framework for understanding the role of context in perception and discuss three approaches for predicting probabilistic performance from observations by efficiently learning local performance models. We compare these approaches with experiments on the monocular and stereo visual odometry systems for a ground robot, and show that they can effectively predict system failures in a wide variety of environments.
更多查看译文
关键词
perception performance,perception system performance,environmental geometry,probabilistic performance,monocular odometry systems,stereo visual odometry systems,system failures,ground robot
AI 理解论文
溯源树
样例
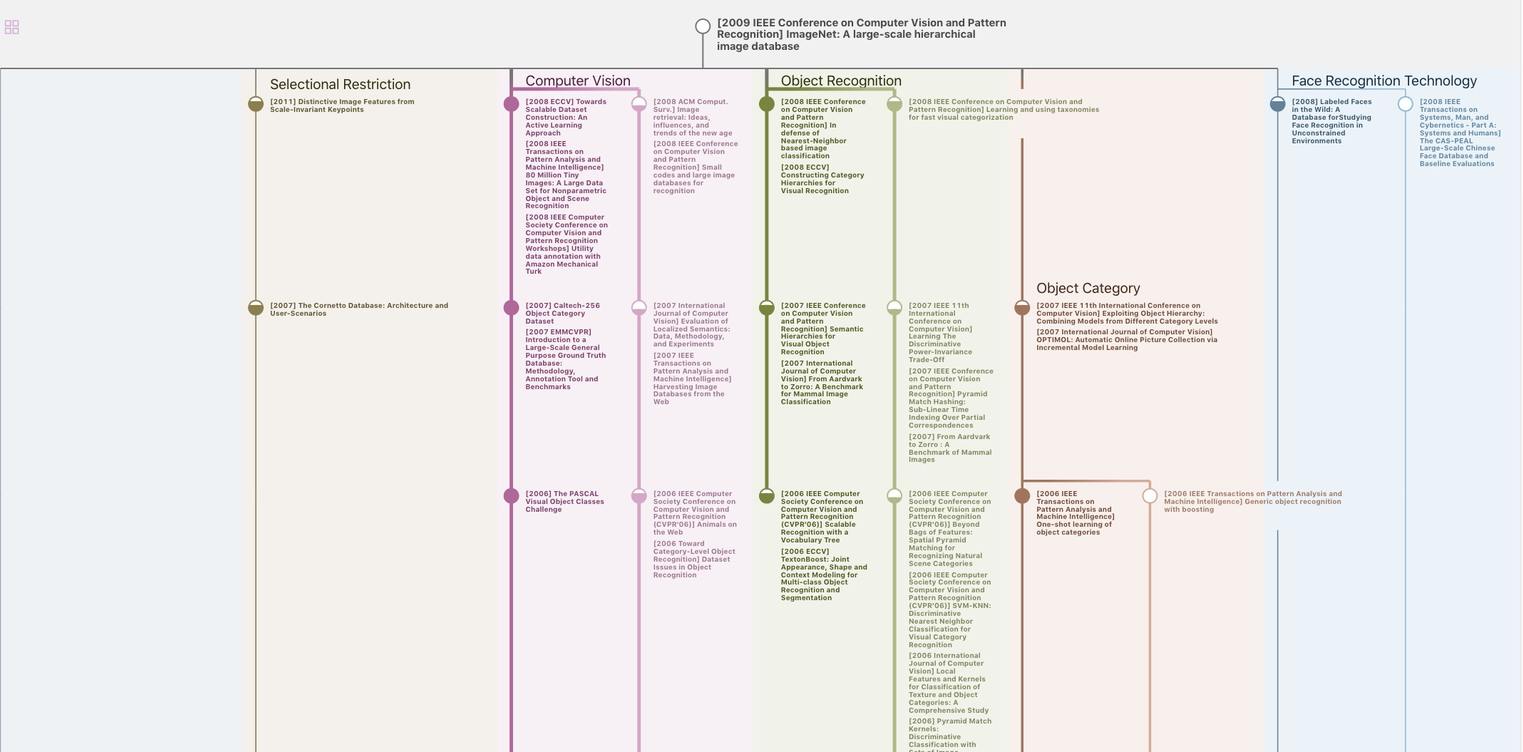
生成溯源树,研究论文发展脉络
Chat Paper
正在生成论文摘要