Towards Intelligent Arbitration Of Diverse Active Learning Queries
2018 IEEE/RSJ INTERNATIONAL CONFERENCE ON INTELLIGENT ROBOTS AND SYSTEMS (IROS)(2018)
Abstract
Active learning literature has explored the selection of optimal queries by a learning agent with respect to given criteria, but prior work in classification has focused only on obtaining labels for queried samples. In contrast, proficient learners, like humans, integrate multiple forms of information during learning. This work seeks to enable an active learner to reason about multiple query types concurrently, aimed at soliciting both instance and feature information from the teacher, and to autonomously arbitrate between queries of different types. We contribute the design of rule-based and decision-theoretic arbitration strategies and evaluate all against baselines of more traditional passive and active learning. Our findings show that all arbitration strategies lead to more efficient learning, compared to the baselines. Moreover, given a dynamically changing environment and constrained questioning budget (typical in human settings), the decision-theoretic strategy statistically outperforms all other methods since it reasons about both what query to make and when to make a query, in order to most effectively utilize its questioning budget.
MoreTranslated text
Key words
diverse active learning queries,optimal queries,learning agent,active learner,decision-theoretic arbitration strategies,decision-theoretic strategy,intelligent arbitration,rule-based arbitration strategies,passive learning
AI Read Science
Must-Reading Tree
Example
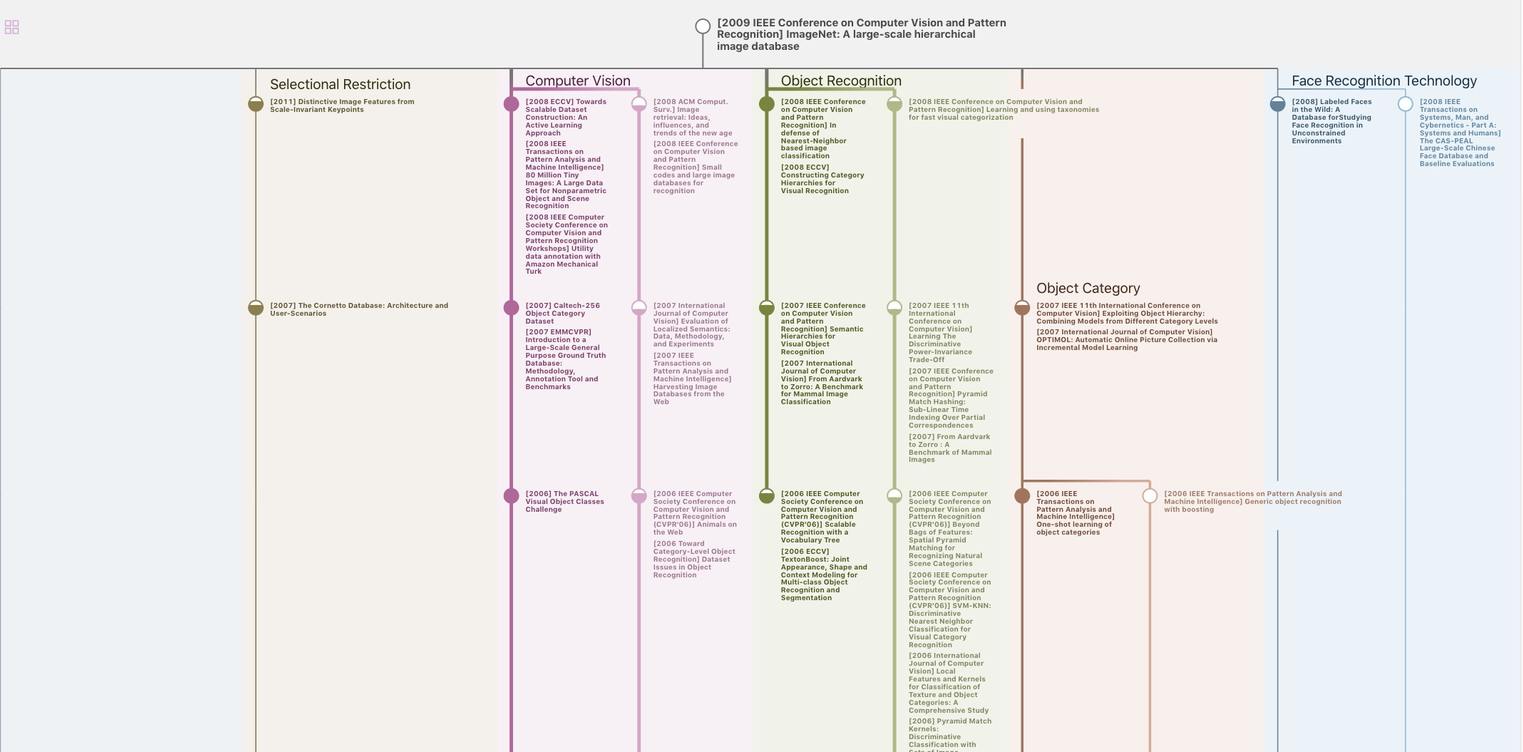
Generate MRT to find the research sequence of this paper
Chat Paper
Summary is being generated by the instructions you defined