A novel non-parametric method for time series classification based on k-Nearest Neighbors and Dynamic Time Warping Barycenter Averaging.
Engineering Applications of Artificial Intelligence(2019)
摘要
Time series classification is one of the most important issues in time series data mining. This problem has attracted more and more attention of researchers in recent years. Among proposed methods in literature, 1-Nearest Neighbor (1-NN), its variants and improvements have been widely considered as hard to be beaten on classification of time series. In this paper, we propose a novel non-parametric method to classify time series. The proposed method, namely Weighted Local Dynamic Time Warping Barycenter Averaging k-Nearest Neighbors (WLDBAk-NN), is an improvement of Local Mean-based k-Nearest Neighbors (LMk-NN) algorithm. It improves LMk-NN in that it replaces the local mean vectors by local Dynamic Time Warping Barycenter (DBA) vectors calculated using our method, namely Weighted DBA (WDBA). By experiments, we show that (i) WLDBAk-NN outperforms the Weighted Local Mean-based k-Nearest Neighbors (WLMk-NN) algorithm, and (ii) both WLMk-NN and WLDBAk-NN outperform 1-NN, LMk-NN, k-Nearest Centroid Neighbors (k-NCN), and LMk-NCN in 85 time series datasets of UCR Time Series Classification Archive. The experimental results also show that new local mean vectors used in WLMk-NN and WLDBAk-NN significantly contribute to the improvement of the performance of time series classification.
更多查看译文
关键词
Time series,Classification,k-Nearest Neighbors,Non-parametric method
AI 理解论文
溯源树
样例
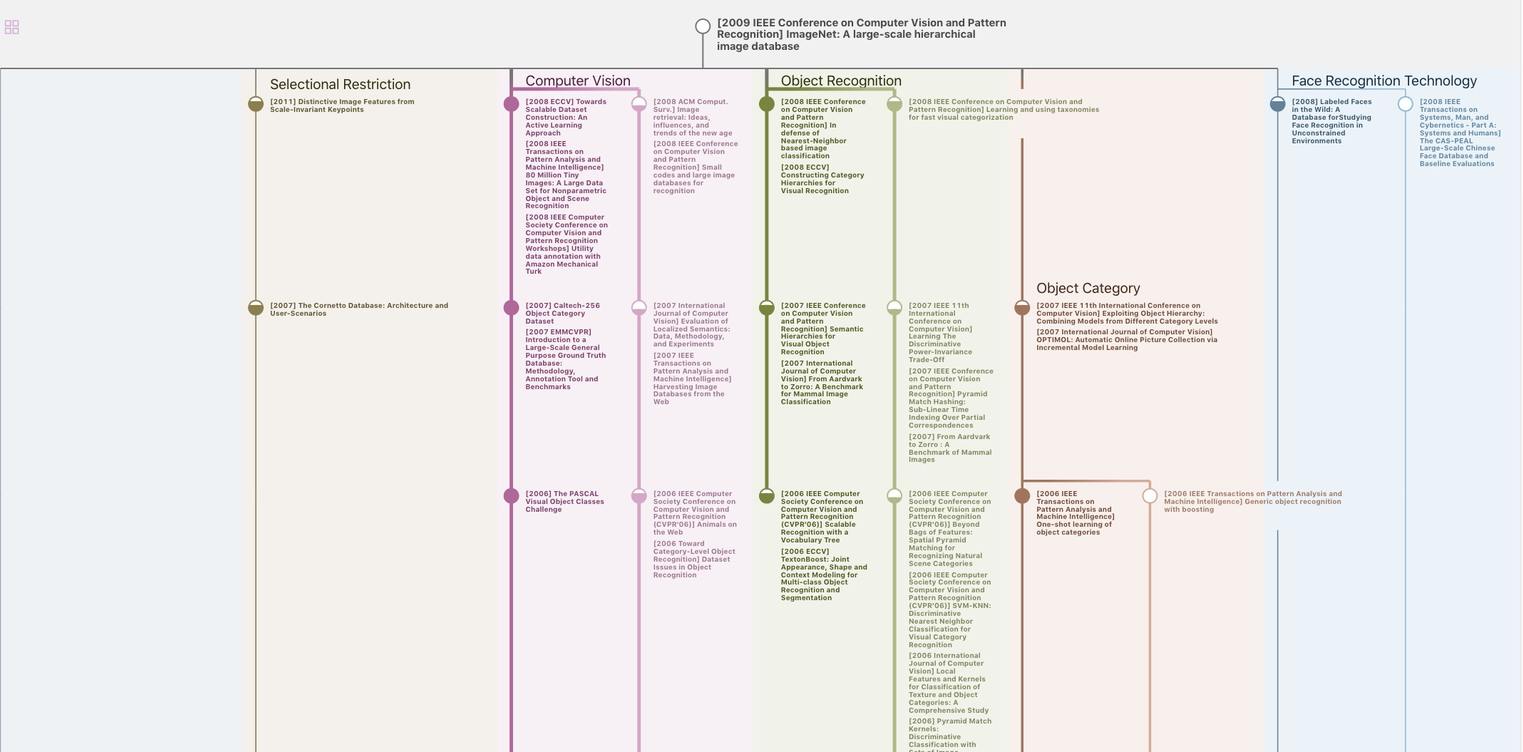
生成溯源树,研究论文发展脉络
Chat Paper
正在生成论文摘要